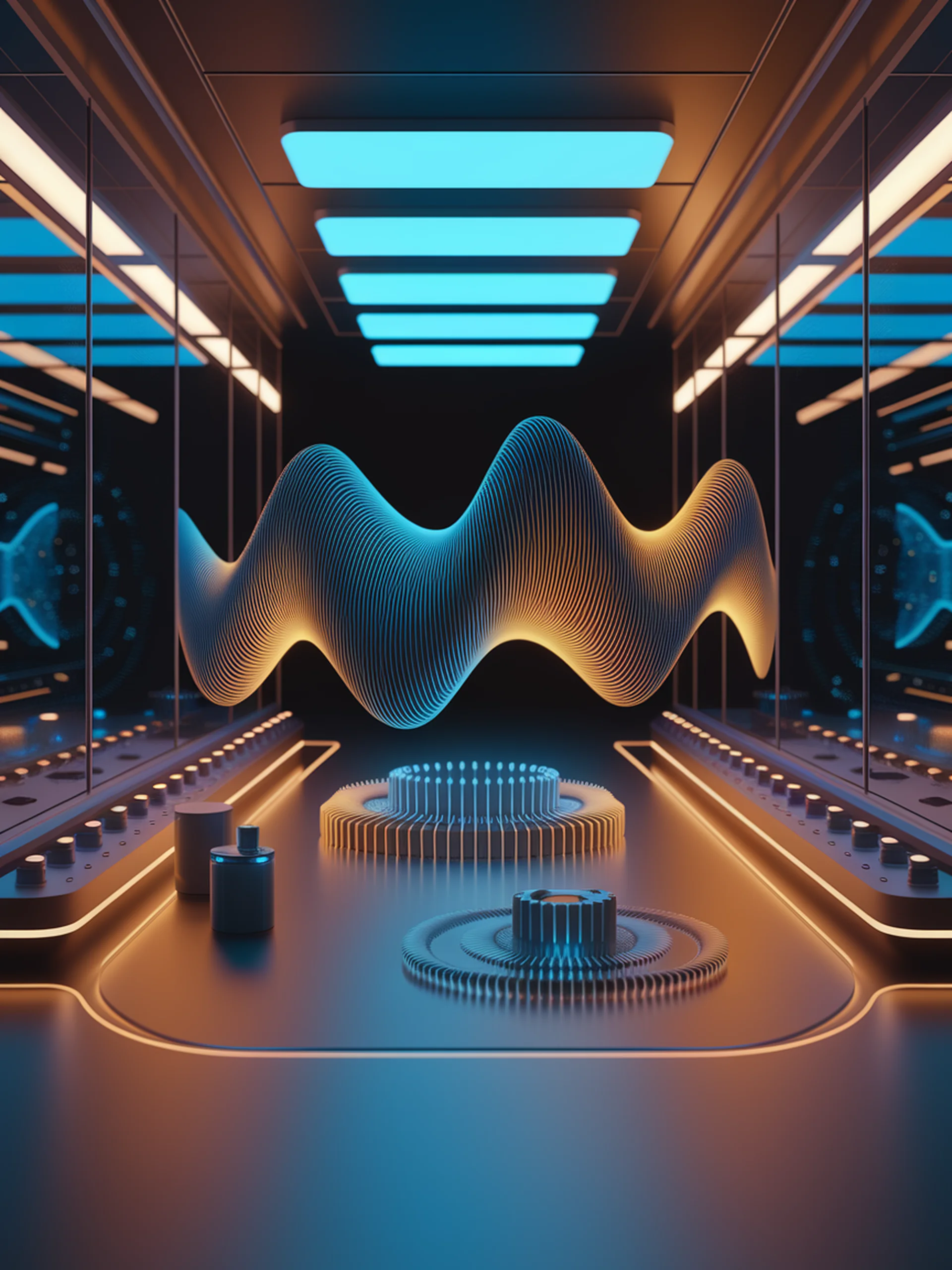
FANformer: Enhancing LLMs Through Periodicity
A novel architecture improving how language models recognize patterns
FANformer introduces effective periodicity modeling to address fundamental limitations in how Transformer-based LLMs learn structured knowledge and patterns.
- Improves learning efficiency by better modeling periodic patterns in data
- Enhances model's ability to establish underlying principles from training data
- Represents a significant architectural improvement to traditional Transformer models
- Demonstrates how cognitive-inspired design can improve LLM performance
This engineering advancement matters because it tackles a core limitation in how today's LLMs process and understand structured information, potentially leading to more efficient and capable language models for enterprise applications.
FANformer: Improving Large Language Models Through Effective Periodicity Modeling