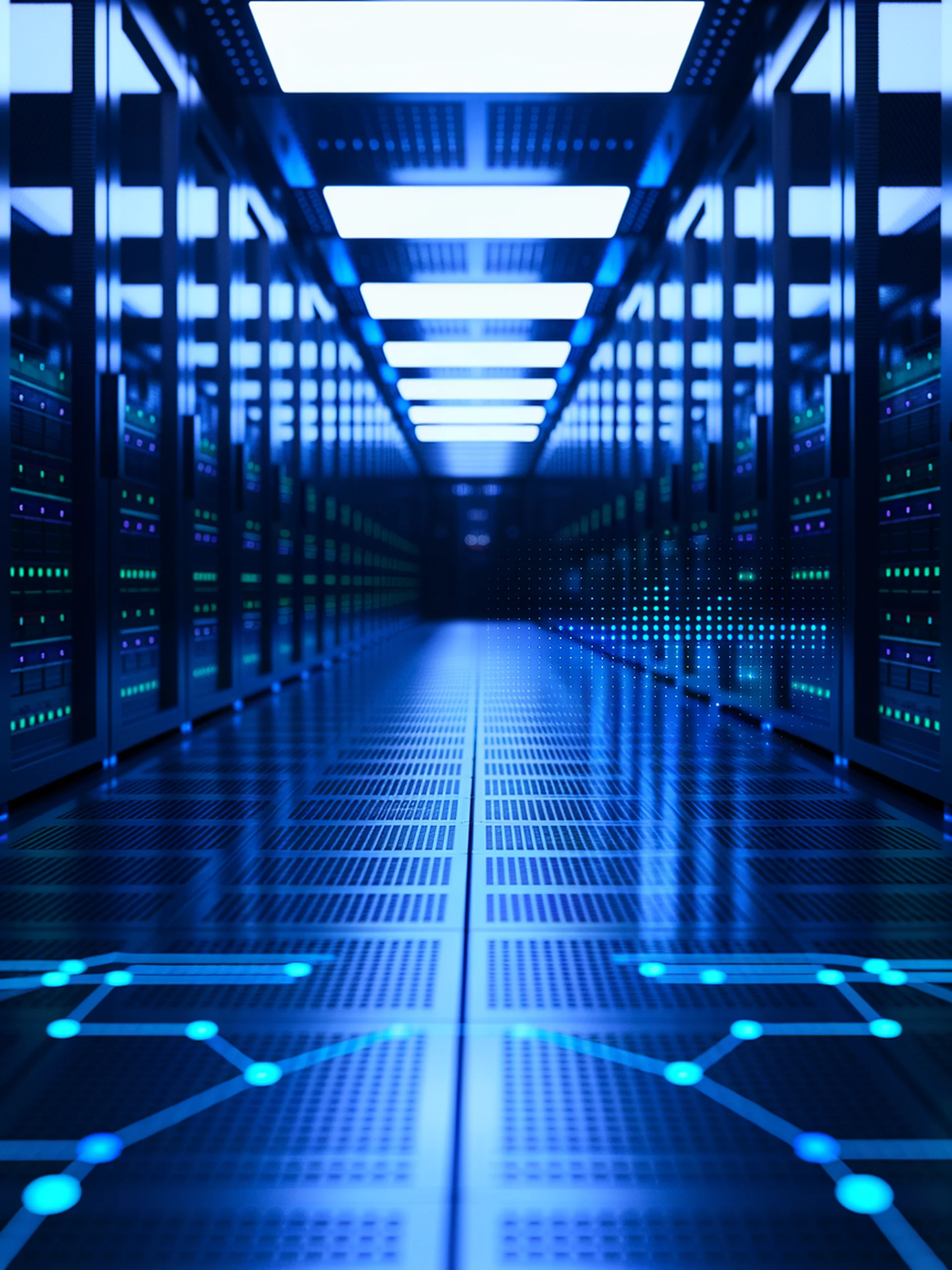
Progressive Sparse Attention
Optimizing LLM Performance for Long-Context Tasks
This research introduces Progressive Sparse Attention (PSA), a novel algorithm-system co-design that significantly improves LLM inference efficiency for long contexts.
- Achieves 3.5-5.4× lower memory usage while maintaining model accuracy
- Utilizes a progressive selection strategy that dynamically increases attention tokens
- Implements a specialized cache management system that reduces memory fragmentation
- Delivers up to 2.3× throughput improvement for long-context LLM serving
For engineering teams, PSA offers a practical solution to the memory bottleneck in LLM deployment, enabling more efficient handling of long documents without specialized hardware requirements.
Progressive Sparse Attention: Algorithm and System Co-design for Efficient Attention in LLM Serving