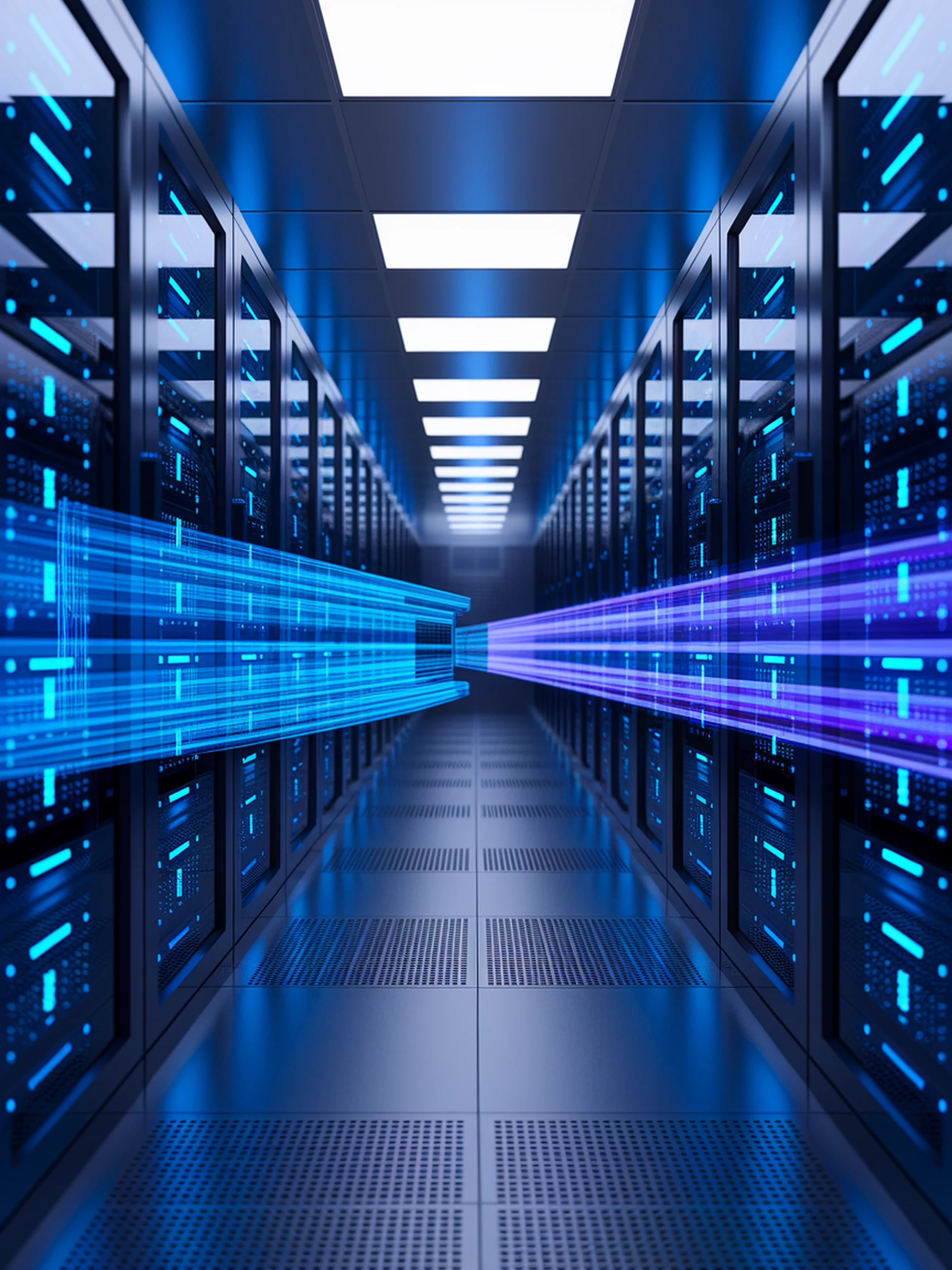
Memory Optimization for LLM Training
Enhancing Pipeline Parallelism with Strategic Memory Offloading
PipeOffload introduces a novel approach to memory management for training large language models, addressing a critical bottleneck in pipeline parallelism.
- Achieves up to 16.1% higher throughput by optimizing memory offload strategies
- Enables training with 2x larger batch sizes without additional hardware
- Implements adaptive offloading decisions based on microbatch execution patterns
- Reduces activation memory requirements while maintaining computational efficiency
This research enables more efficient scaling of LLM training pipelines, making it possible to train larger models with existing infrastructure or reduce hardware costs for current model sizes.
PipeOffload: Improving Scalability of Pipeline Parallelism with Memory Optimization