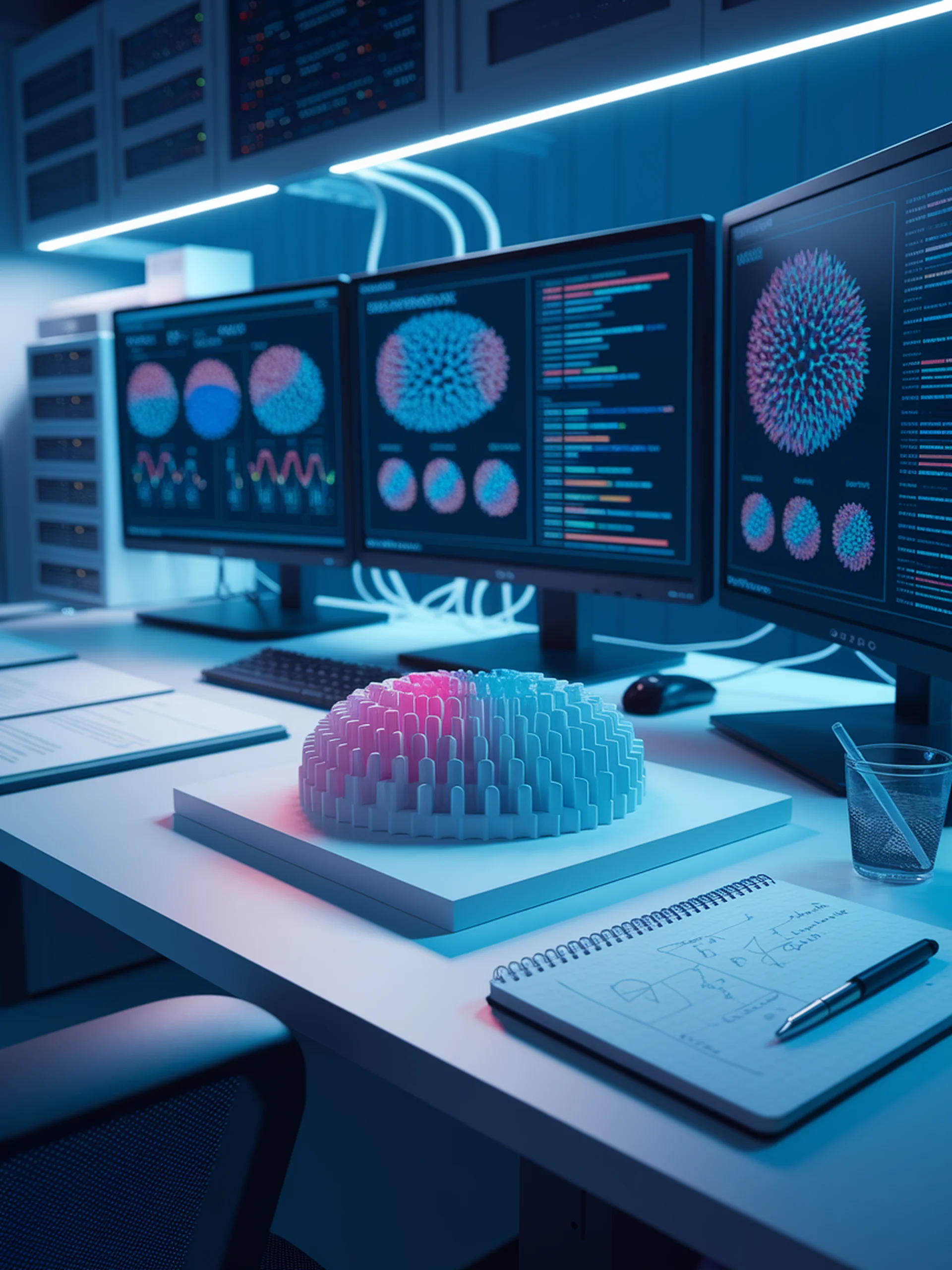
LLM Quantization Breakthrough
Using Kurtosis to Tackle Outliers in Model Compression
KurTail introduces a novel approach to compress large language models while preserving performance by addressing the outlier problem in quantization.
- Leverages Kurtosis-based rotation to mitigate activation outliers that typically hinder efficient quantization
- Enables effective 4-bit quantization of weights, activations, and other model components
- Optimizes for tailedness in weight distributions, significantly improving compression reliability
- Maintains model performance while reducing size and computational requirements
This engineering innovation matters because it enables more efficient deployment of large language models on resource-constrained devices, potentially democratizing access to AI technology while reducing energy consumption.