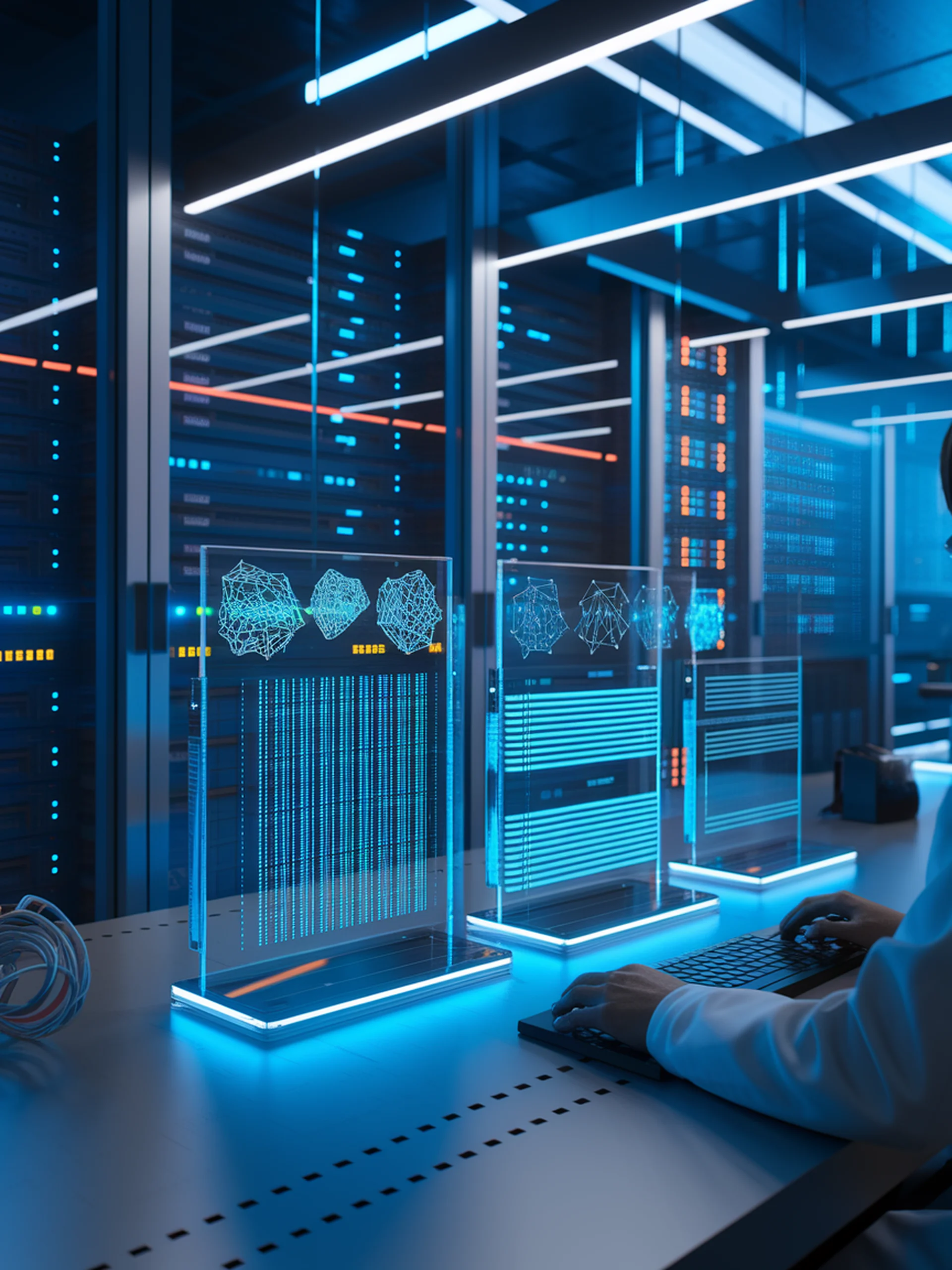
Streamlining LLMs with Linear Recurrence
Transforming standard models into more efficient structures without retraining
Liger efficiently converts standard transformer-based LLMs into linear recurrent structures with significant performance advantages.
- Enables linear-time training and constant-memory inference
- Preserves model quality while improving deployment efficiency
- Eliminates the need for costly pretraining of non-standard architectures
- Creates more accessible, resource-efficient AI systems
This engineering breakthrough addresses a critical challenge in AI deployment by making large language models more computationally efficient and accessible for real-world applications, potentially reducing infrastructure costs and environmental impact.
Liger: Linearizing Large Language Models to Gated Recurrent Structures