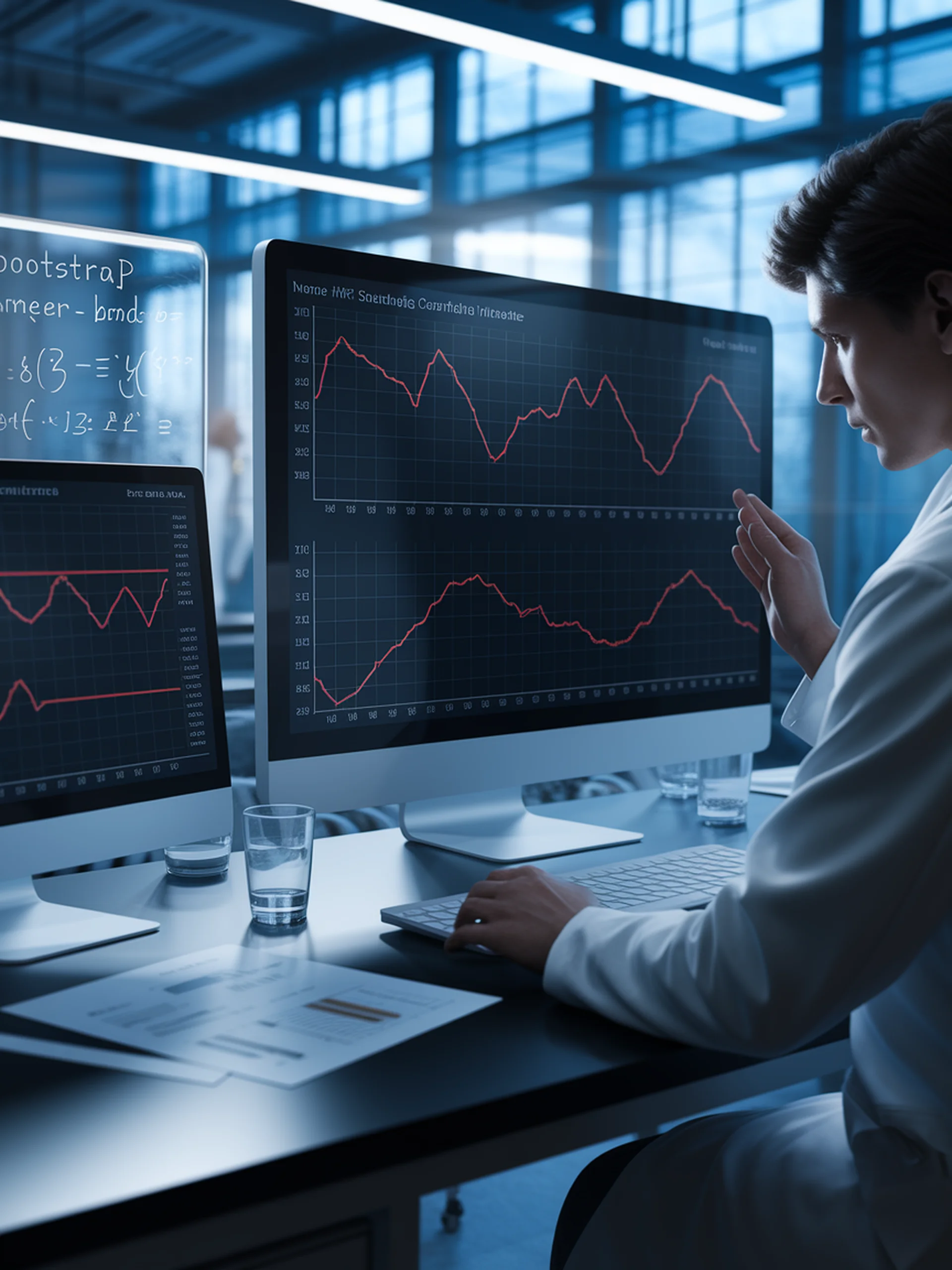
Statistical Pitfalls in LLM Evaluation
Why the Central Limit Theorem fails for small datasets
This research challenges standard statistical practices in LLM evaluation, demonstrating that Central Limit Theorem (CLT) methods produce unreliable error estimates with small datasets.
- CLT-based methods require hundreds of datapoints to provide valid uncertainty estimates
- With fewer samples, error bars are systematically too narrow and p-values too small
- Better alternatives include bootstrap methods and Bayesian approaches
- Proper statistical evaluation prevents overconfidence in model capabilities, crucial for secure deployment
For security professionals, this means many published LLM comparisons may have overstated significance, potentially leading to flawed deployment decisions based on statistical artifacts rather than meaningful performance differences.
Position: Don't use the CLT in LLM evals with fewer than a few hundred datapoints