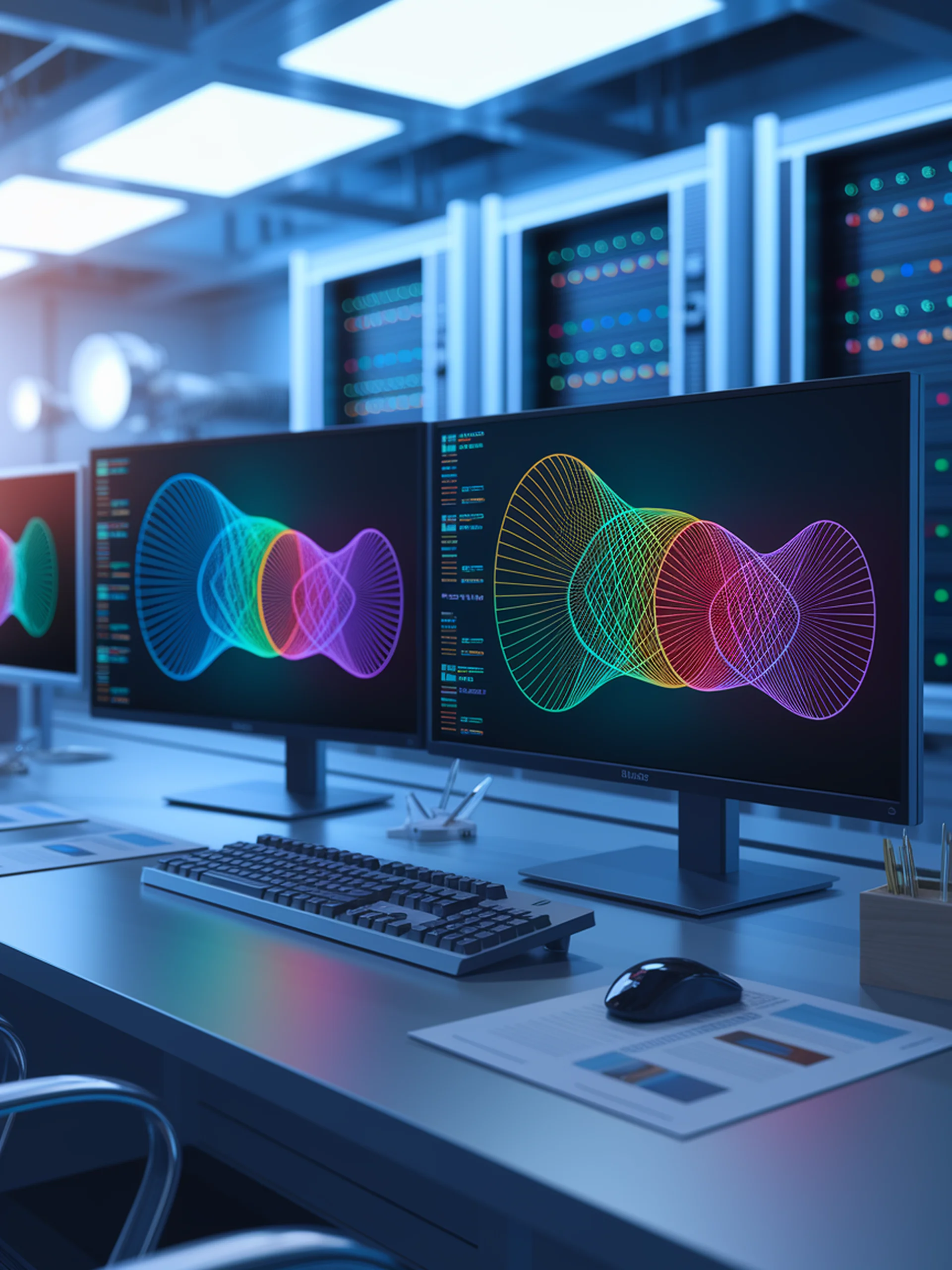
Unlocking the Secrets of Rotary Embeddings in LLMs
Revealing hidden patterns in positional encoding mechanisms
This research provides deep analysis of Rotary Positional Encodings (RoPE) in large language models, revealing consistent patterns across model layers and attention heads.
- Identifies specific patterns and outliers in queries and keys when using rotary embeddings
- Demonstrates consistency of these patterns both within individual models and across different models
- Offers insights into how position information is encoded in transformer architectures
- Advances understanding of the fundamental mechanisms enabling LLMs to process sequential information
This engineering-focused analysis helps demystify how modern language models maintain awareness of word position and sequence order, potentially enabling more efficient model design and improved performance in future architectures.
Rotary Outliers and Rotary Offset Features in Large Language Models