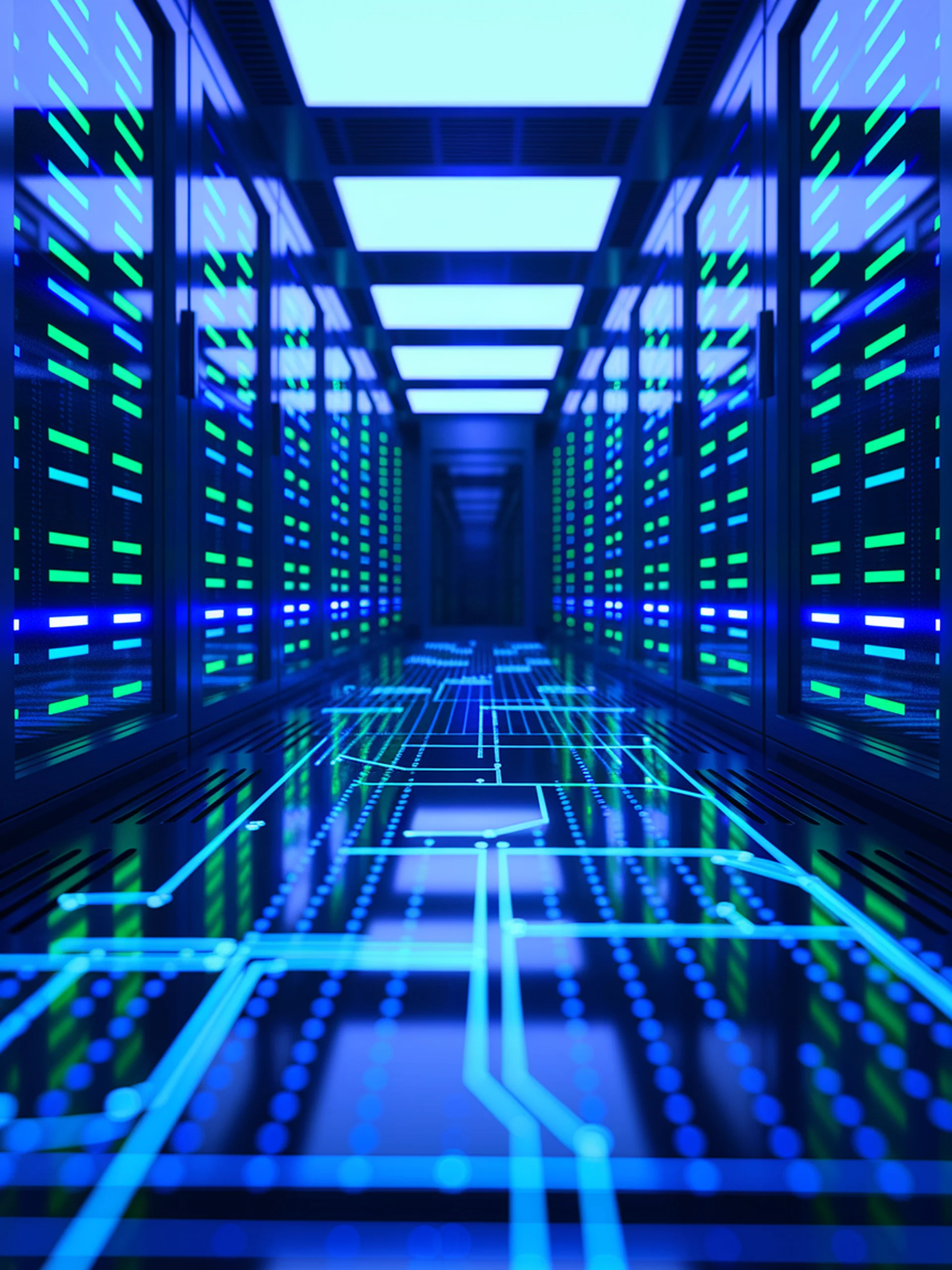
Accelerating LLM Inference with PASA
A robust low-precision attention algorithm that eliminates overflow issues
PASA introduces a mathematically-equivalent, low-precision algorithm that significantly accelerates attention calculation for long-sequence inference tasks in large language models.
- Online pseudo-average shifting technique enables half-precision computation throughout Flash Attention
- Global recovering mechanism ensures computational stability without overflow
- Delivers faster inference speed without sacrificing model accuracy
- Particularly valuable for text and image/video generation tasks with long sequences
This engineering breakthrough addresses a critical bottleneck in LLM deployment, making inference more efficient and accessible for production environments where computational resources are constrained.