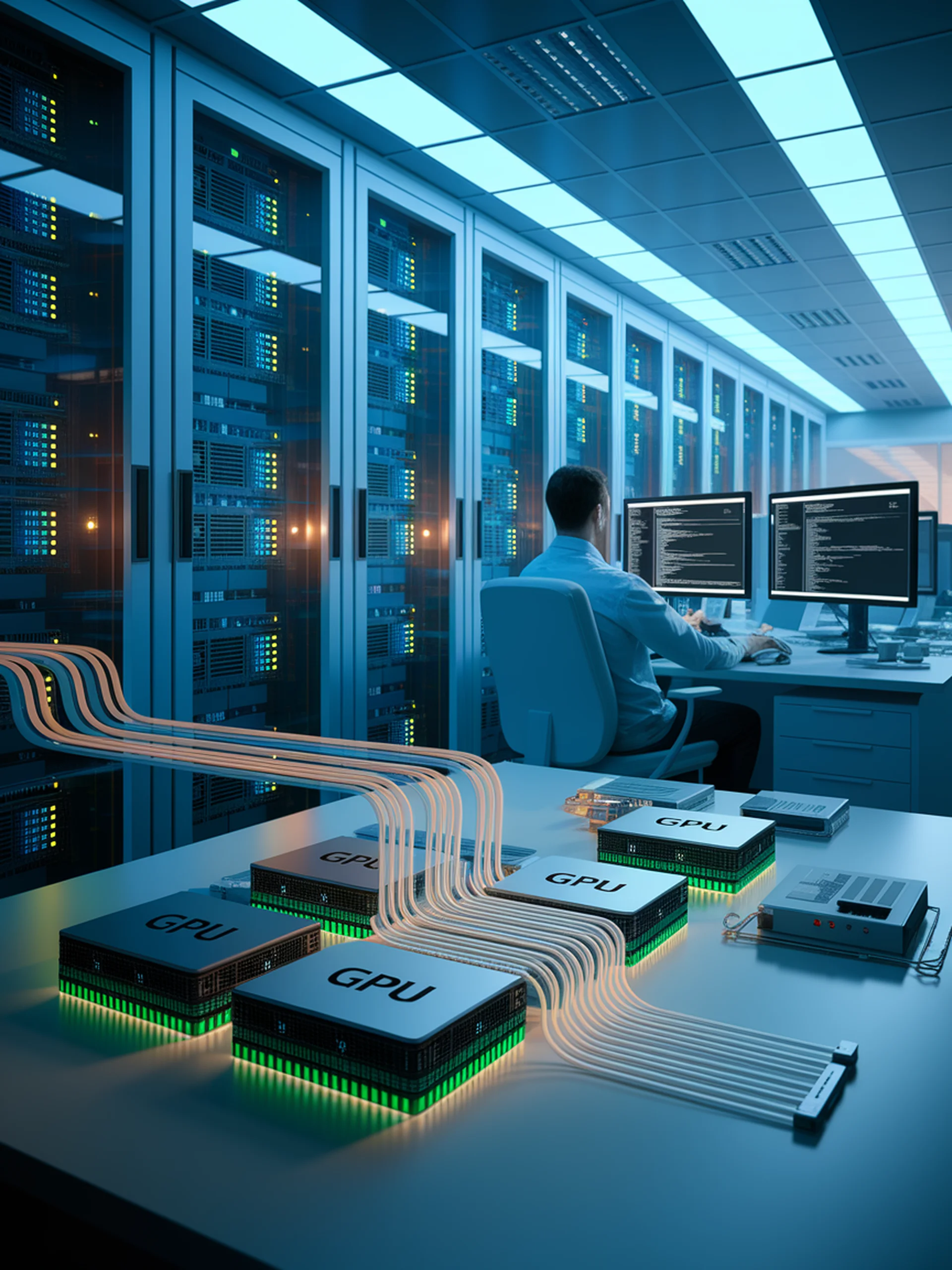
Breaking GPU Memory Barriers
Automating Efficient Heterogeneous Training for Large Language Models
AutoHete is a novel system that enables training larger LLMs with limited GPU resources by automatically optimizing heterogeneous CPU-GPU memory allocation.
- Reduces communication overhead by up to 29.3% compared to existing methods
- Automatically determines optimal parameter placement between CPU and GPU memory
- Achieves up to 98% computation-communication overlap for efficient training
- Enables researchers with limited GPU resources to train larger models
This innovation democratizes LLM training by making it accessible to more researchers and organizations without requiring massive GPU infrastructure investments, potentially accelerating innovation in the field.
AutoHete: An Automatic and Efficient Heterogeneous Training System for LLMs