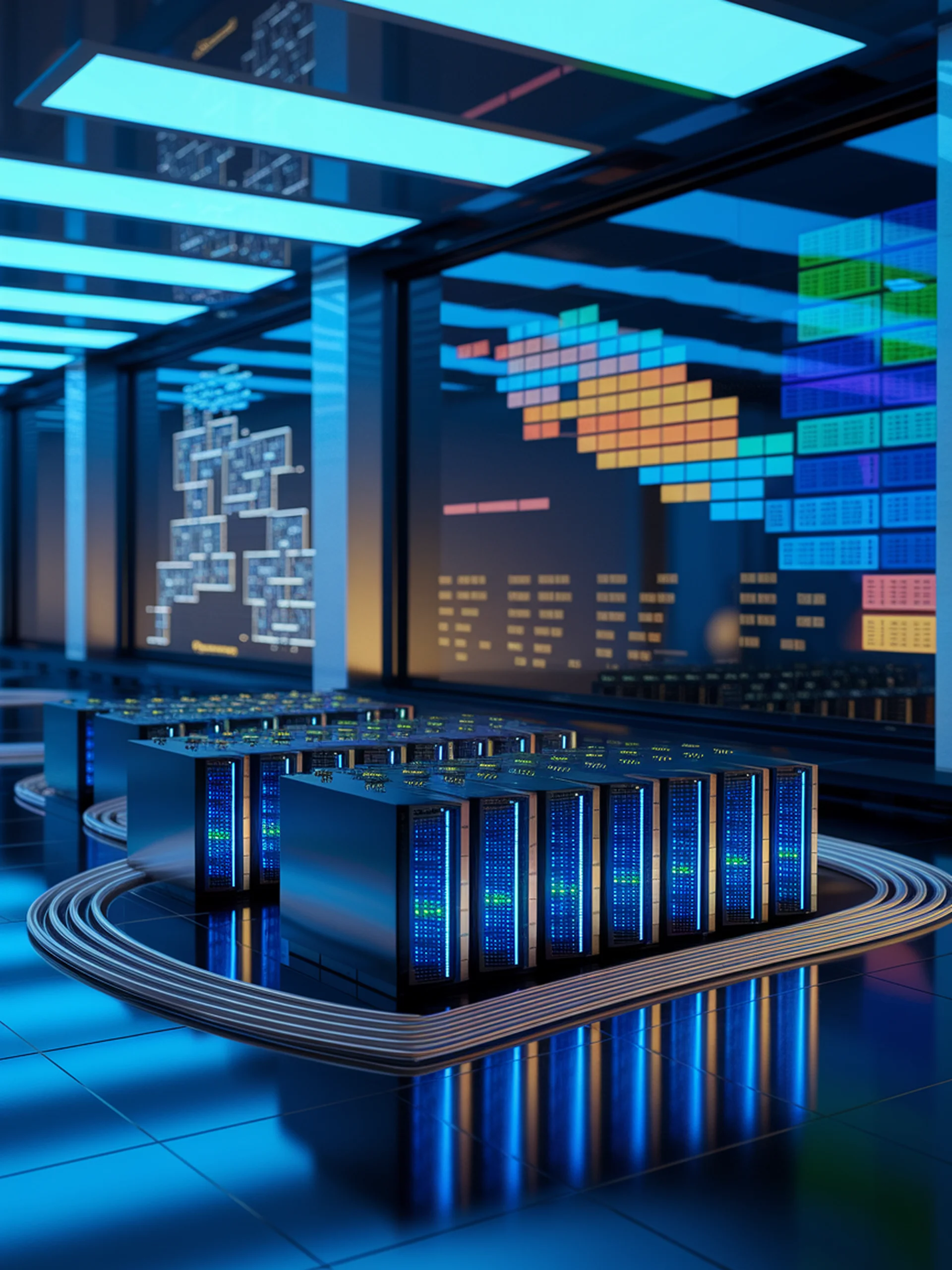
ChunkFlow: Solving the Long Context Challenge
A more efficient approach to fine-tuning LLMs on variable-length sequences
ChunkFlow introduces a novel technique for efficiently fine-tuning large language models on datasets with both short and long sequences without the traditional memory and computational bottlenecks.
- Addresses the long-tail distribution in training data where most sequences are short with occasional longer ones
- Optimizes memory usage through innovative chunking that balances computational load across GPUs
- Improves training efficiency by focusing computational resources where they're most needed
- Solves distributed training challenges like load imbalance in data parallelism
This engineering breakthrough matters because it makes long-context LLM fine-tuning more practical and accessible, potentially enabling more powerful models that can handle documents of varying lengths without excessive computational costs.
Original Paper: Efficient Long Context Fine-tuning with Chunk Flow