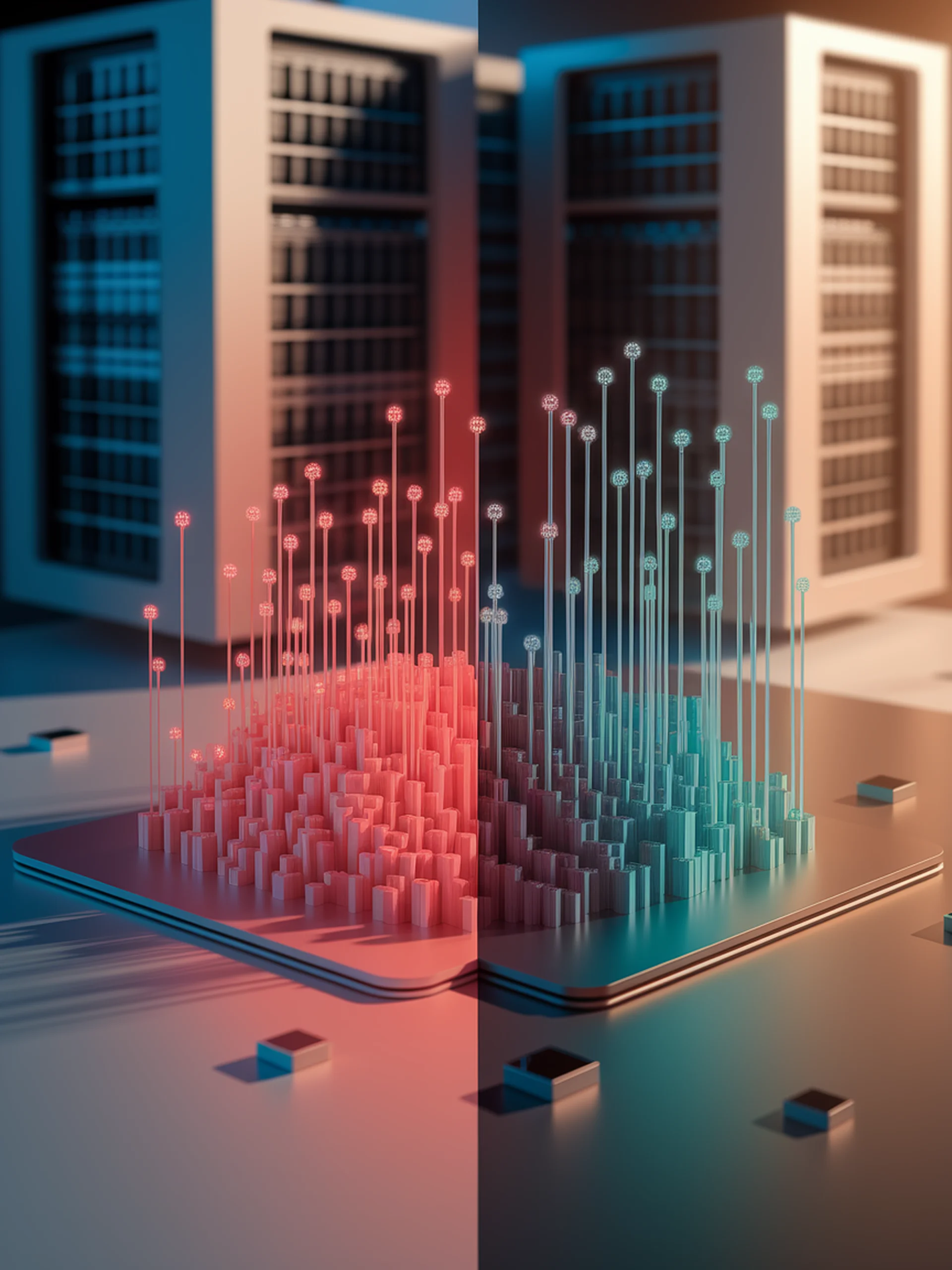
Smarter LLM Compression
Using entropy to selectively quantize models across architectures
Entropy-Weighted Quantization (EWQ) is a novel approach that intelligently compresses large language models by analyzing entropy patterns across transformer blocks.
- Identifies which model components can be safely quantized with minimal performance impact
- Works universally across different model architectures and sizes
- Outperforms uniform quantization techniques while maintaining model quality
- Reduces memory requirements without architecture-specific tuning
This advancement enables more efficient deployment of LLMs across diverse computing environments, making powerful AI models accessible with fewer computational resources.
Universality of Layer-Level Entropy-Weighted Quantization Beyond Model Architecture and Size