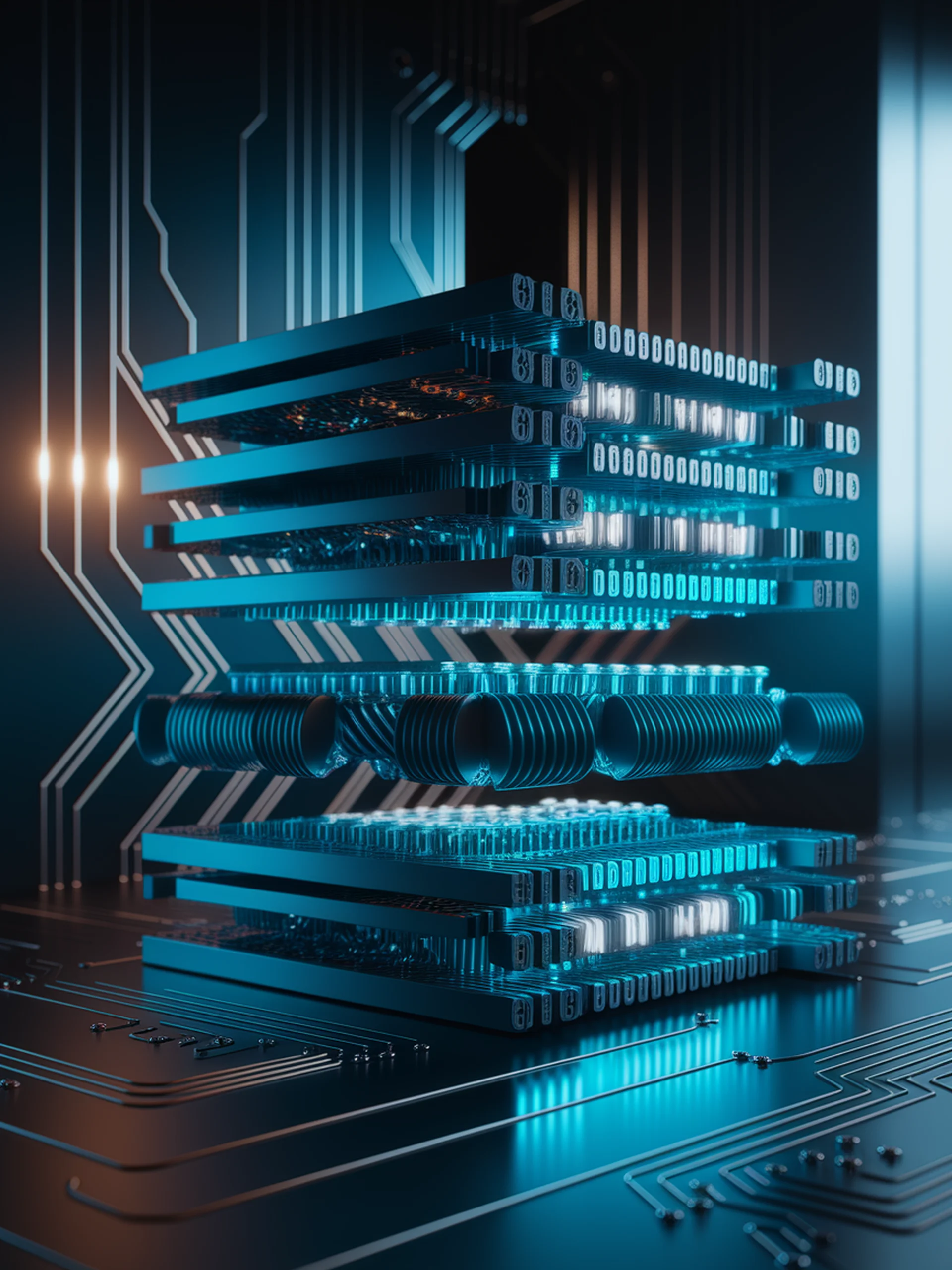
TransformerX: Reimagining LLM Architecture
Enhancing LLM efficiency with multi-scale convolution and adaptive mechanisms
This research introduces a novel architecture that addresses key computational and performance limitations in large language models.
- Multi-scale convolution integrated into transformer blocks improves feature interaction capabilities
- Learnable dense residual skip connections mitigate gradient vanishing issues
- Multi-token prediction mechanism increases training and inference efficiency
- Adaptive activation functions enhance model flexibility across diverse tasks
These engineering innovations significantly improve computational efficiency while maintaining or enhancing performance, representing an important step toward more resource-efficient yet powerful language models.