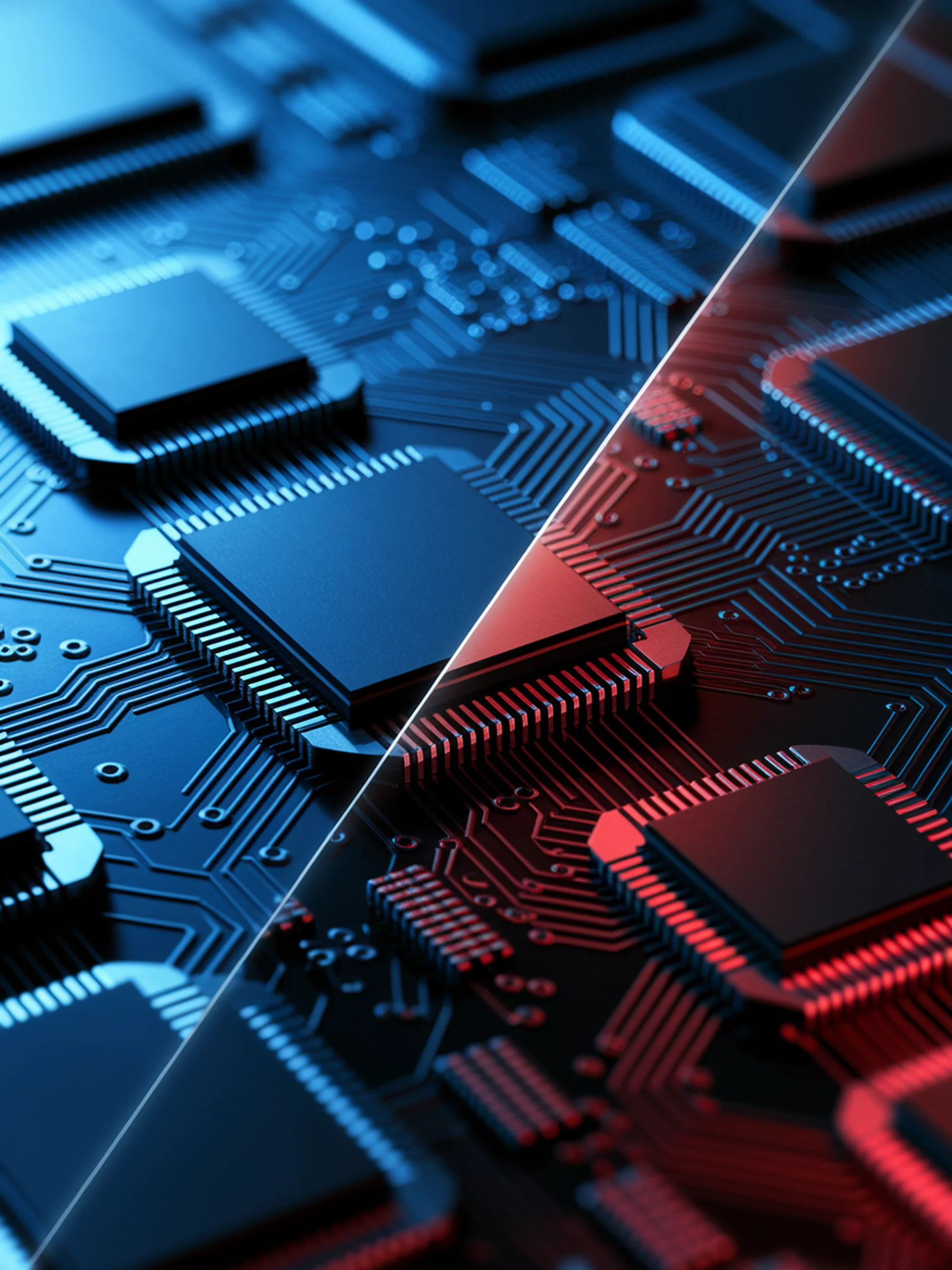
Security Implications of LVLM Compression
How model compression affects vision-language models' trustworthiness
This research introduces a comprehensive benchmarking framework for evaluating how compression techniques affect Large Vision-Language Models (LVLMs) beyond just performance metrics.
Key Findings:
- Compression impacts ethical behavior including toxicity identification and bias detection
- Different compression methods (KV cache, weight compression, quantization) have varying effects on LVLM capabilities
- Security vulnerabilities may emerge from compressed models that aren't present in full-size versions
- Framework enables systematic evaluation of compression trade-offs
Why This Matters:
As organizations deploy compressed LVLMs to meet computational constraints, understanding security implications becomes critical. This research provides a structured approach to evaluate whether compression compromises models' ability to detect harmful content or introduces new biases.
LVLM-Compress-Bench: Benchmarking the Broader Impact of Large Vision-Language Model Compression