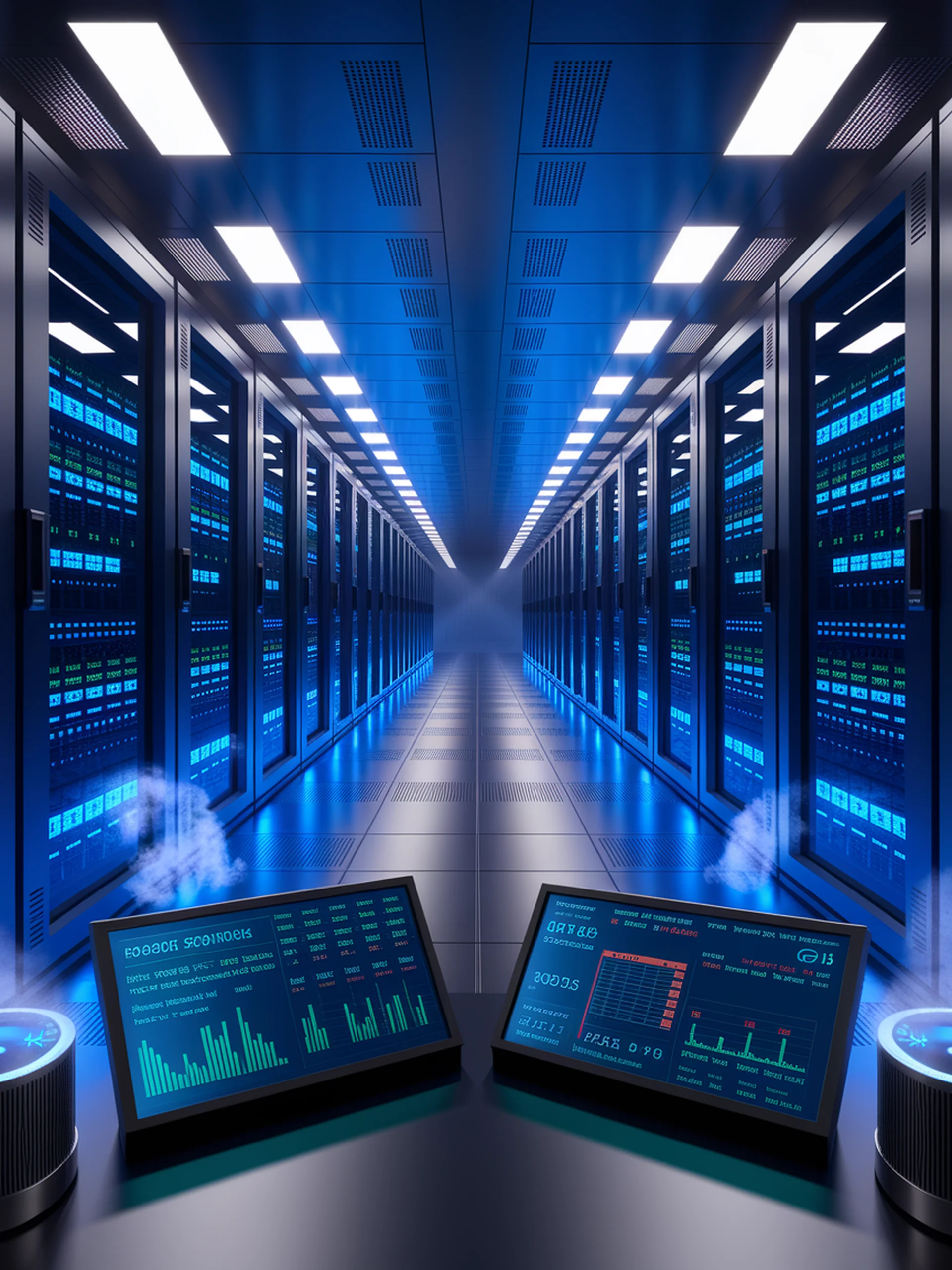
Optimizing GPU Usage for Serverless AI
A Dynamic Resource Allocation System for Large Language Models
Dilu introduces introspective elasticity to solve GPU fragmentation in serverless deep learning, particularly for resource-intensive LLMs.
- Reduces GPU wastage by 15-94% through fine-grained dynamic resource allocation
- Enables on-demand GPU resourcing that adapts to workload shifts
- Maintains quality of service while improving cost-effectiveness
- Addresses a critical engineering challenge for serverless deep learning deployments
This research significantly improves resource utilization for AI serving platforms, allowing organizations to maximize GPU investments while maintaining performance for large language model inference.
Dilu: Enabling GPU Resourcing-on-Demand for Serverless DL Serving via Introspective Elasticity