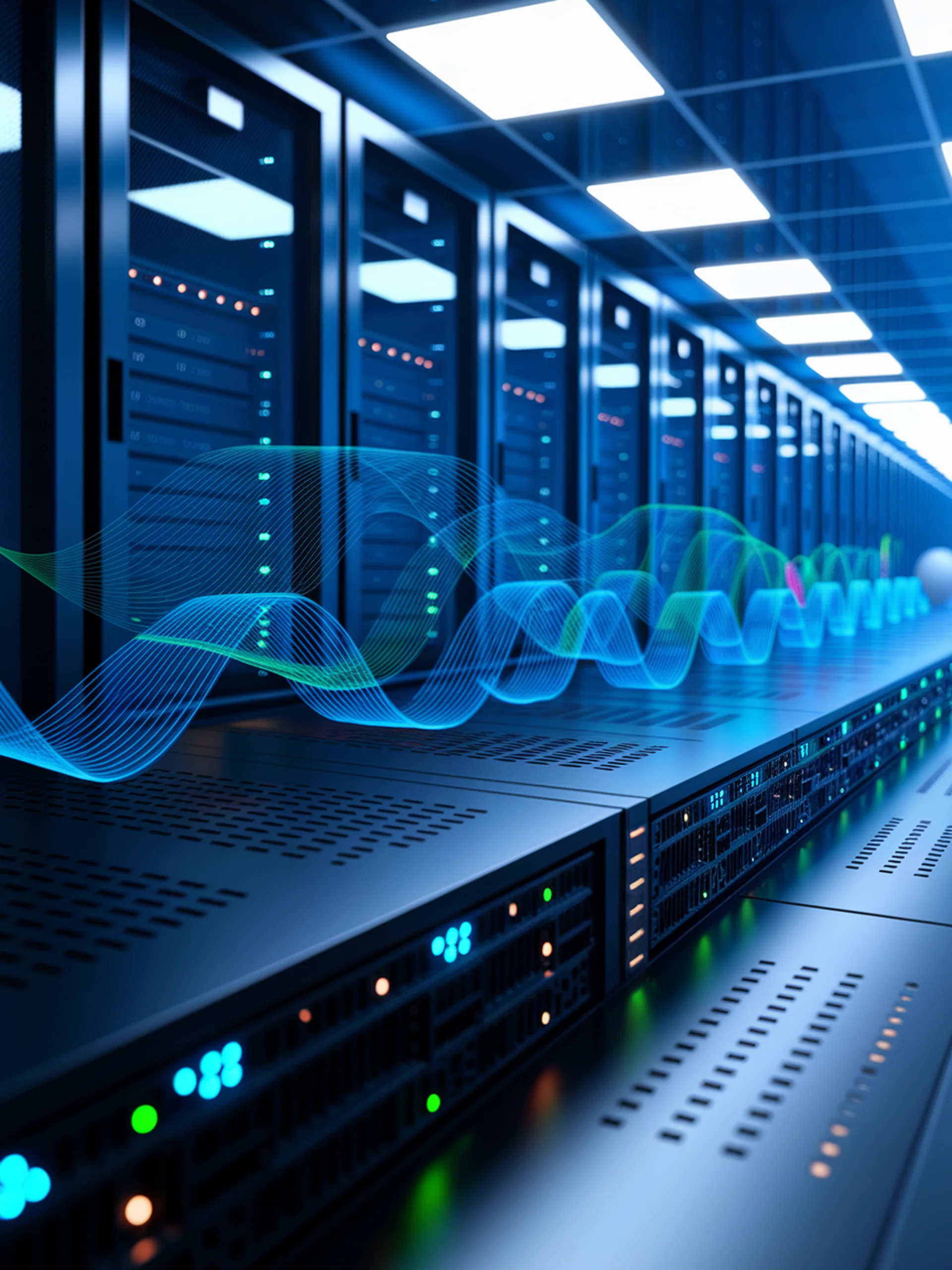
Boosting LLM Performance: Dynamic Batch Optimization
Memory-aware, SLA-constrained approach to maximize inference throughput
This research introduces an adaptive batching system that maximizes LLM inference throughput while maintaining service quality commitments.
- Dynamically adjusts batch sizes based on real-time GPU memory conditions
- Enforces service-level agreements (SLAs) through intelligent request scheduling
- Overcomes limitations of static batching approaches used in current systems
- Significantly improves throughput efficiency on memory-constrained GPU deployments
For engineering teams, this innovation enables more efficient utilization of existing hardware resources when serving large language models at scale, potentially reducing infrastructure costs while maintaining performance guarantees.