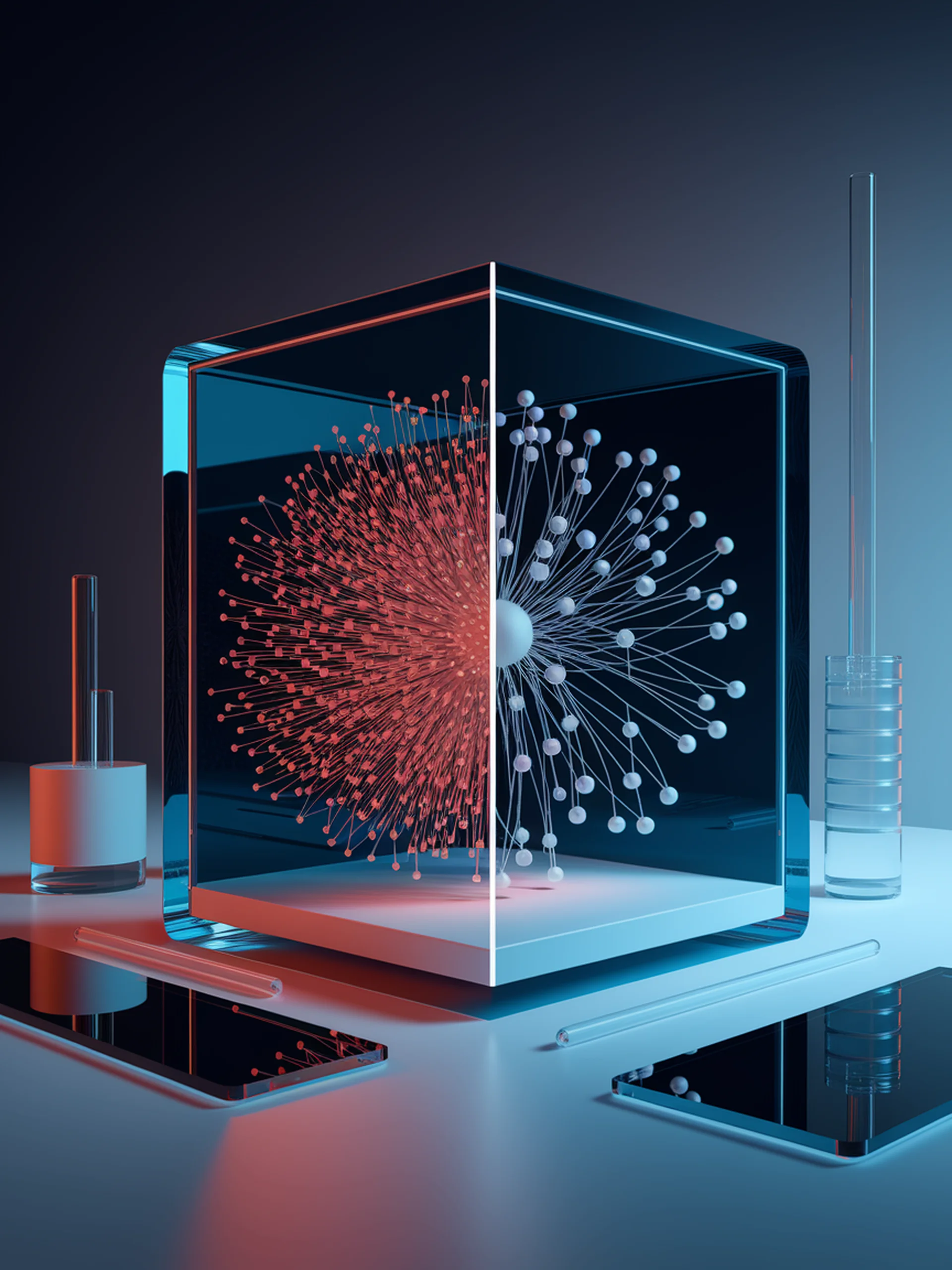
Shrinking Multimodal Models Without Losing Power
Leveraging Attention Sparsity for Extreme Model Compression
CASP introduces a breakthrough compression technique for Large Multimodal Models (LMMs) that exploits the natural sparsity in attention matrices to achieve extreme compression ratios.
- Capitalizes on inherent attention sparsity in multimodal inputs to enable more aggressive compression
- Implements low-rank decomposition and optimal bit allocation techniques for efficient compression
- Achieves up to 32x compression while maintaining model performance
- Enables deployment of powerful LMMs on resource-constrained devices
This engineering innovation addresses critical challenges in deploying large AI models in real-world applications, reducing computational costs while preserving functionality.
CASP: Compression of Large Multimodal Models Based on Attention Sparsity