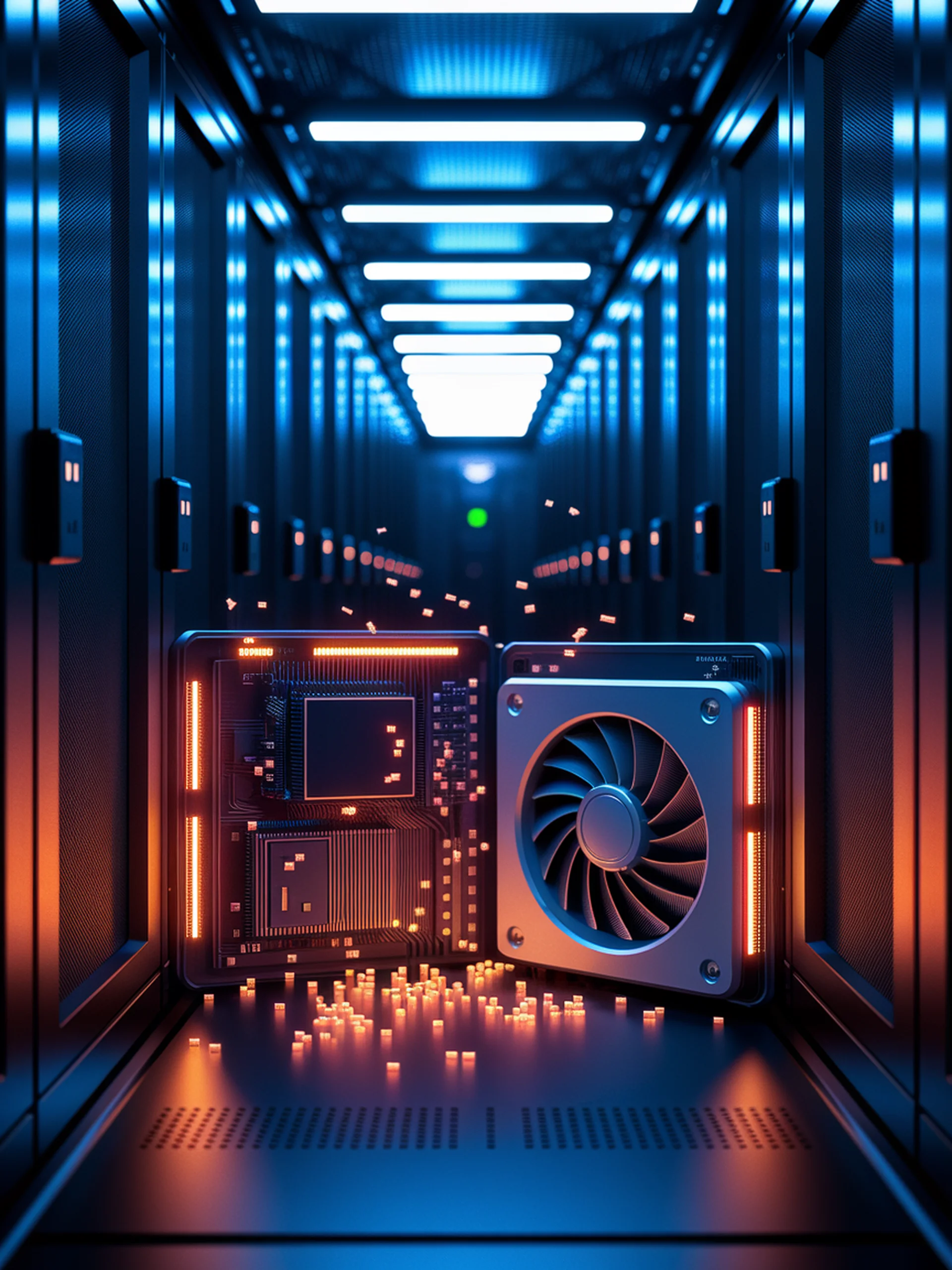
Unlocking LLM Inference Bottlenecks
How Memory Management Constraints GPU Performance in Large-Batch Processing
This research reveals that GPU memory management bottlenecks, not compute limitations, are the true barrier to scaling large-batch LLM inference.
- Memory fragmentation and allocation inefficiencies create significant performance degradation
- The paper introduces a novel Batching Configuration Advisor (BCA) that intelligently optimizes memory usage
- Tests show up to 33% throughput improvement across various models without requiring code changes
- For smaller models, memory constraints impact performance much earlier than previously believed
For engineering teams, this research provides immediate practical value by offering a tool to maximize existing hardware efficiency, potentially reducing inference costs and latency without additional hardware investment.
Mind the Memory Gap: Unveiling GPU Bottlenecks in Large-Batch LLM Inference