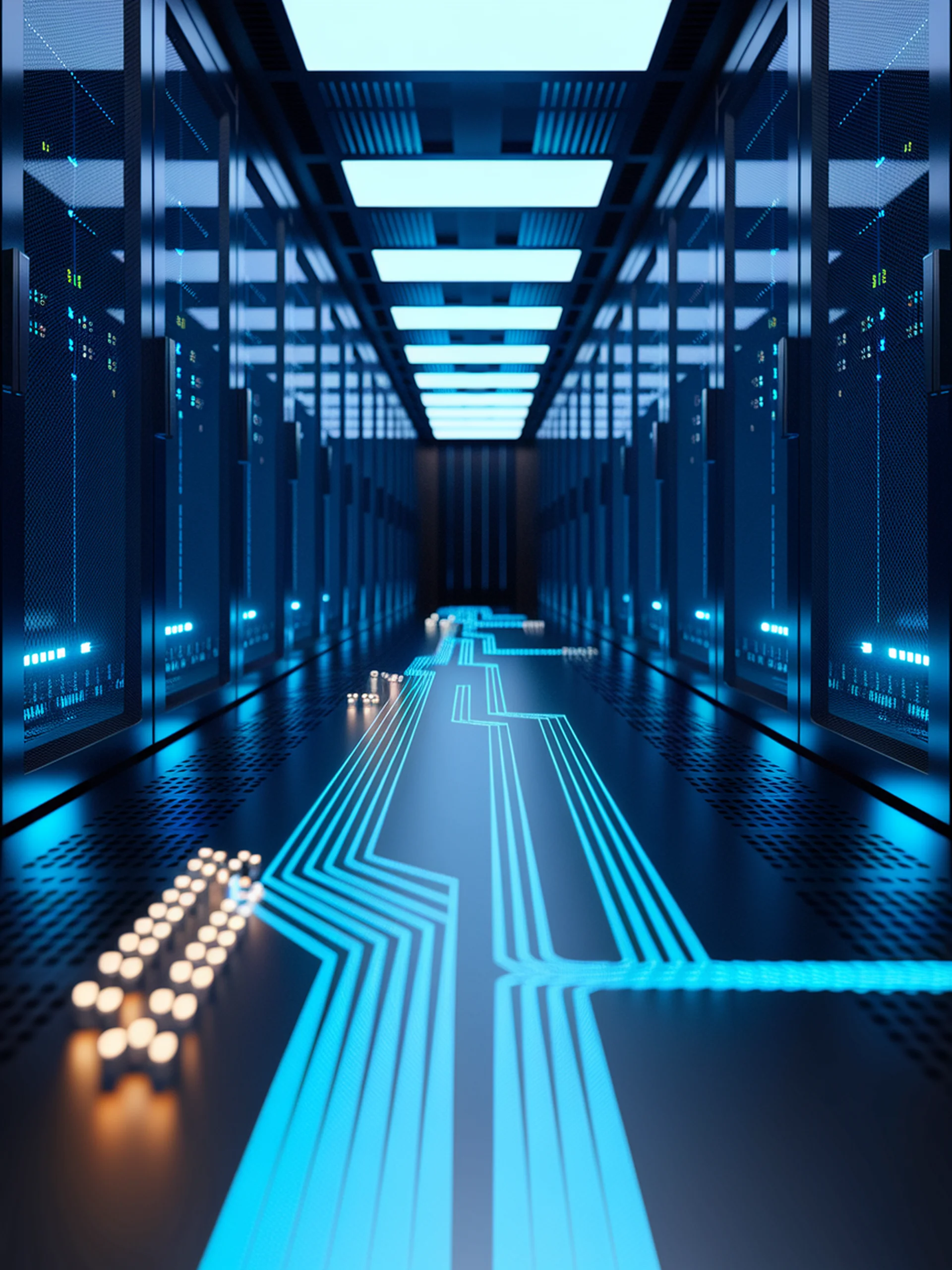
Smarter Memory Management for LLMs
How models can self-optimize their memory use for long contexts
This research introduces a technique that lets large language models intelligently manage their own memory by identifying which information to keep and which to discard during processing of very long texts.
- Addresses a critical memory bottleneck when processing contexts of 128K-1M tokens
- Leverages the model's own attention patterns to determine which tokens can be safely evicted
- Demonstrates that LLMs implicitly "know" which information is important to retain
- Achieves significant efficiency gains without compromising performance
For engineering teams, this approach offers a practical path to deploying long-context models without requiring specialized hardware or drastic architectural changes, making advanced AI capabilities more accessible and cost-effective.
LLMs Know What to Drop: Self-Attention Guided KV Cache Eviction for Efficient Long-Context Inference