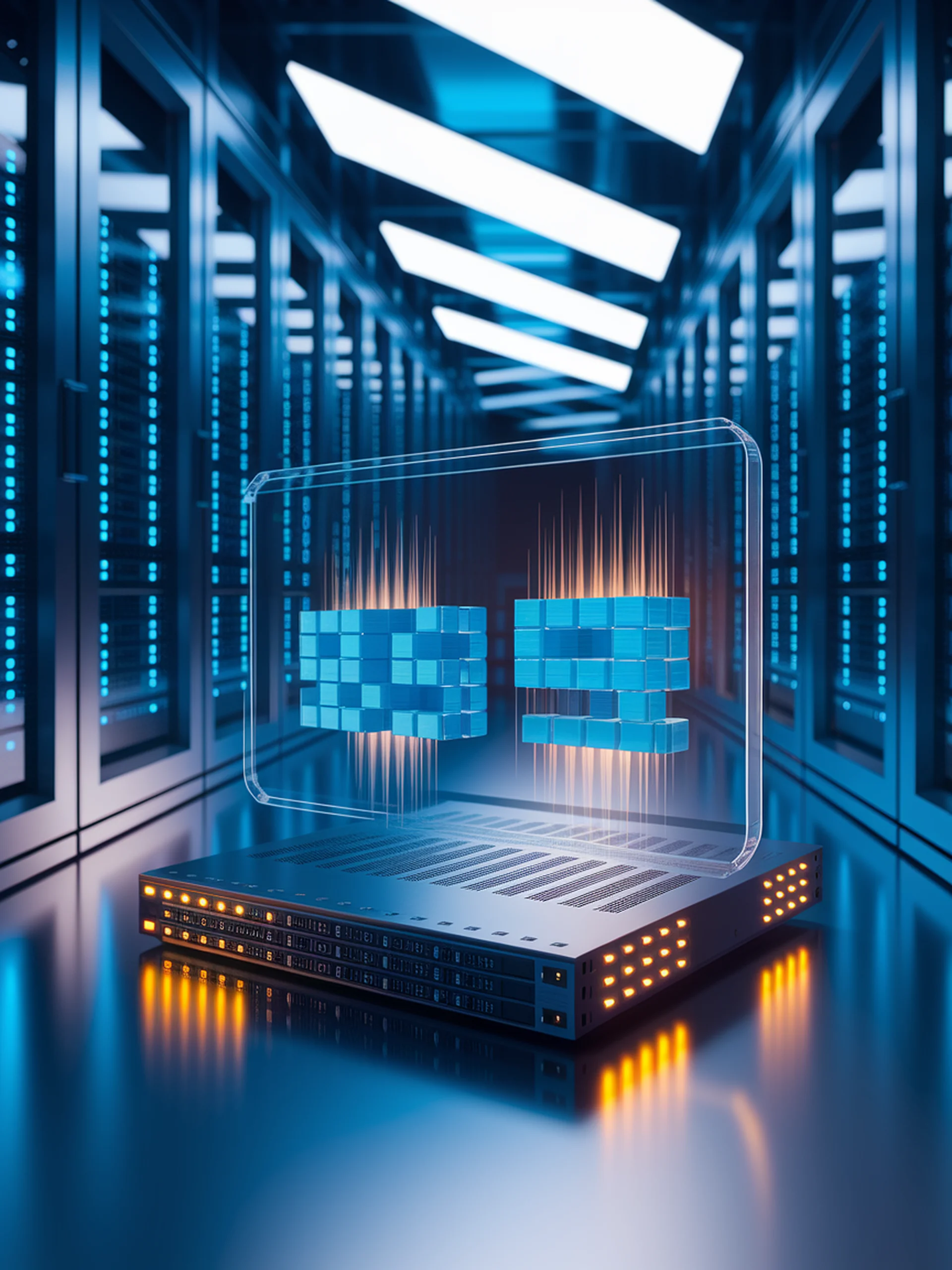
Memory-Efficient LLMs for Long Contexts
Zero-shot compression technique for KV caches without retraining
ZSMerge introduces a novel approach to compress Key-Value caches in LLMs, enabling efficient processing of longer contexts without additional training.
- Achieves up to 4x memory reduction with minimal performance degradation
- Uses semantic clustering to identify and merge similar tokens in the KV cache
- Implements dynamic compression strategies that adapt to different content types
- Requires zero additional training or model modifications
This research addresses a critical engineering challenge for deploying LLMs in memory-constrained environments, making long-context processing more accessible and practical for real-world applications.
ZSMerge: Zero-Shot KV Cache Compression for Memory-Efficient Long-Context LLMs