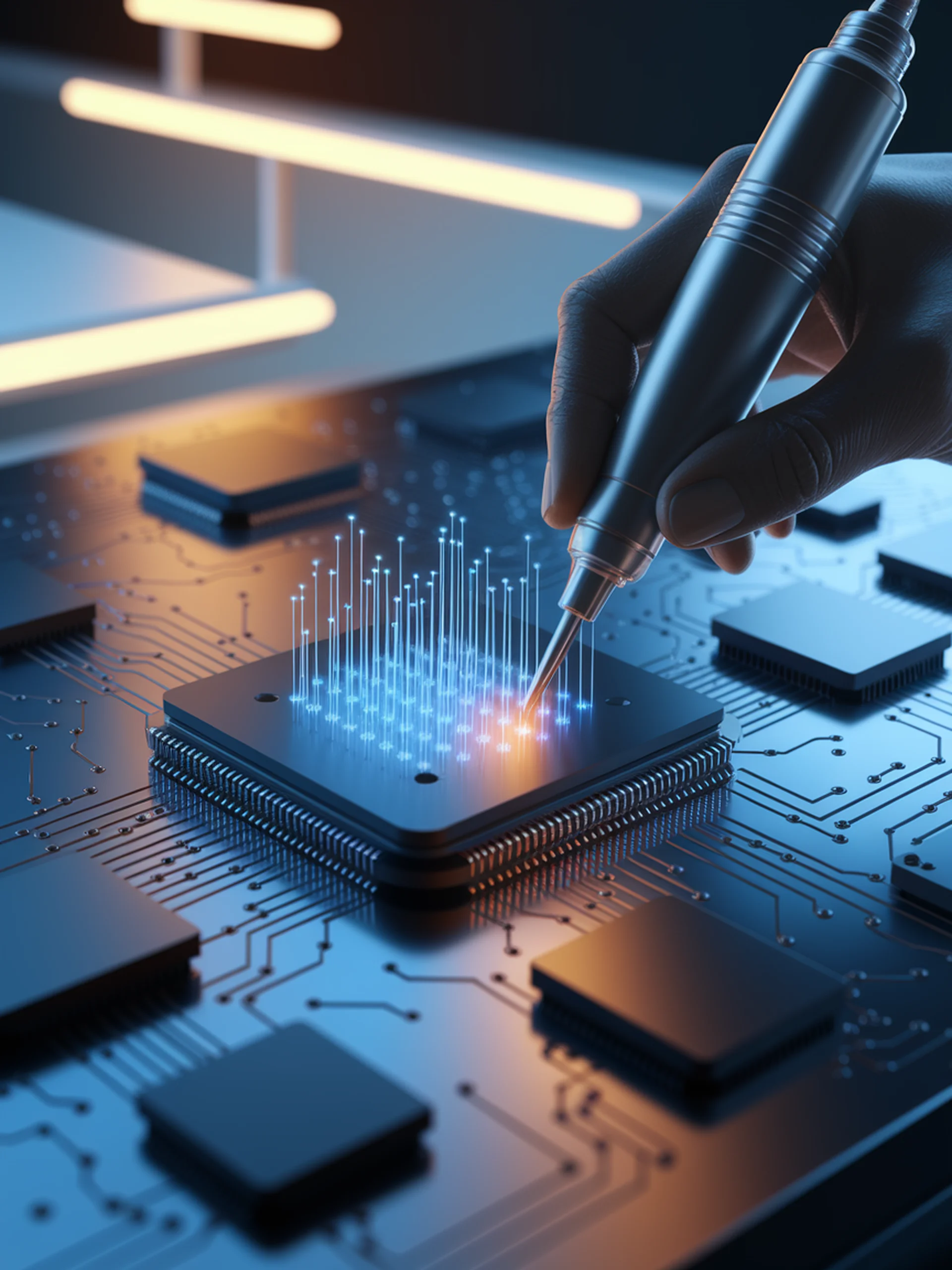
Smart Pruning for Leaner LLMs
Tailoring Sparsity Patterns for Optimal Performance
This research introduces a plug-and-play mixed sparsity approach that intelligently prunes large language models by recognizing different sensitivity levels across model layers.
- Achieves up to 75% parameter reduction with minimal performance drop
- Utilizes Fisher Information Matrix to identify crucial network components
- Implements adaptive N:M sparsity patterns tailored to each layer's sensitivity
- Demonstrates effectiveness across various model sizes and architectures
This engineering breakthrough enables more efficient deployment of LLMs on resource-constrained devices while maintaining performance, potentially expanding real-world applications in edge computing and mobile environments.
Towards Extreme Pruning of LLMs with Plug-and-Play Mixed Sparsity