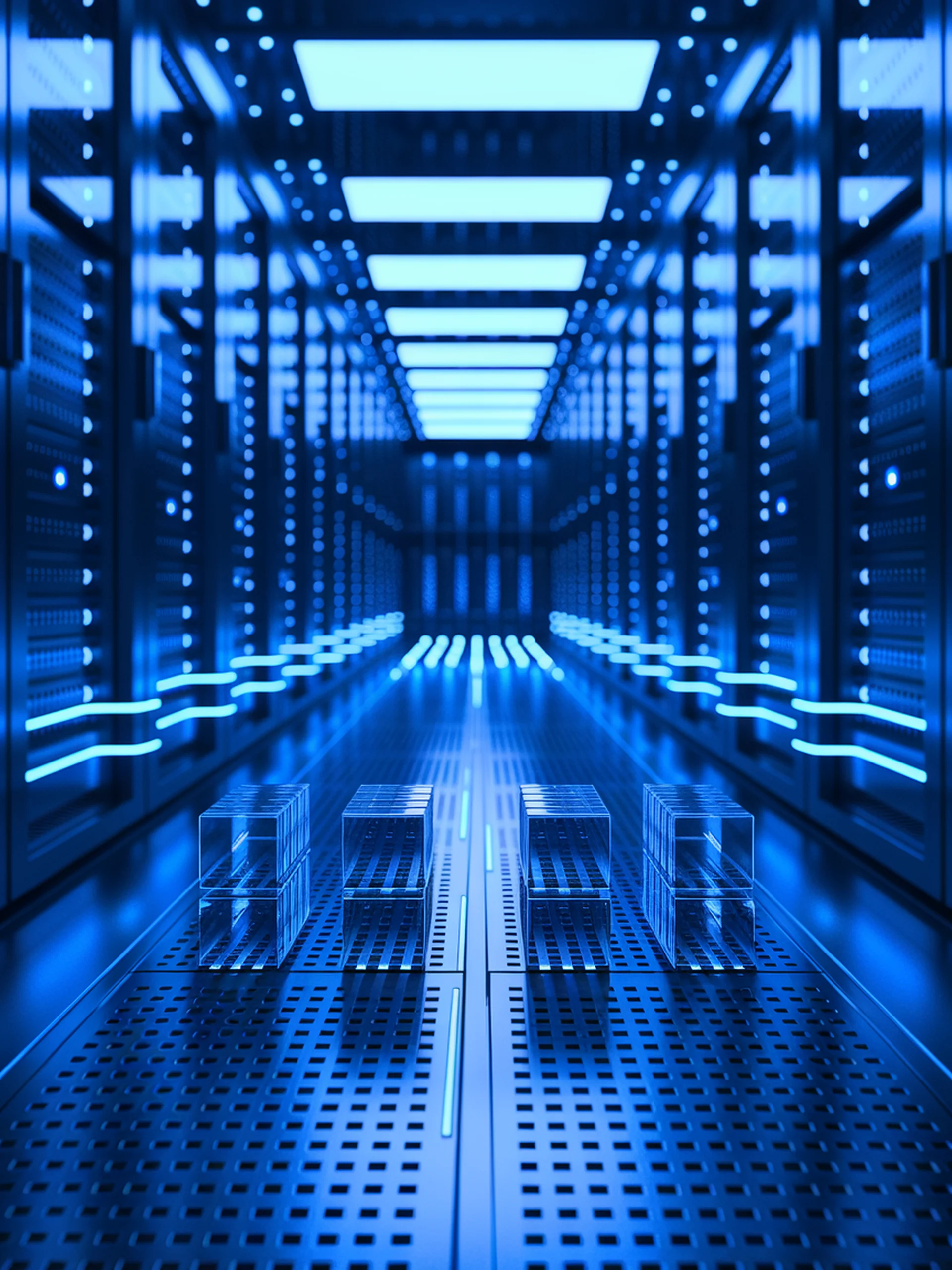
Optimizing LLM Memory Efficiency
Systematic analysis of KV cache compression techniques
This research systematically explores approaches to reduce memory requirements for large language models by compressing the Key-Value (KV) cache, enabling longer context windows with less computational resources.
- KV cache compression tackles the quadratic growth in attention costs as context length increases
- Presents a comprehensive taxonomy of compression methods categorized by underlying principles
- Analyzes performance trade-offs between compression ratios and model accuracy
- Offers practical insights for engineering more efficient LLM architectures
For AI engineers, this research provides crucial guidance on implementing memory optimization techniques that can significantly reduce infrastructure costs while maintaining model performance.
Key, Value, Compress: A Systematic Exploration of KV Cache Compression Techniques