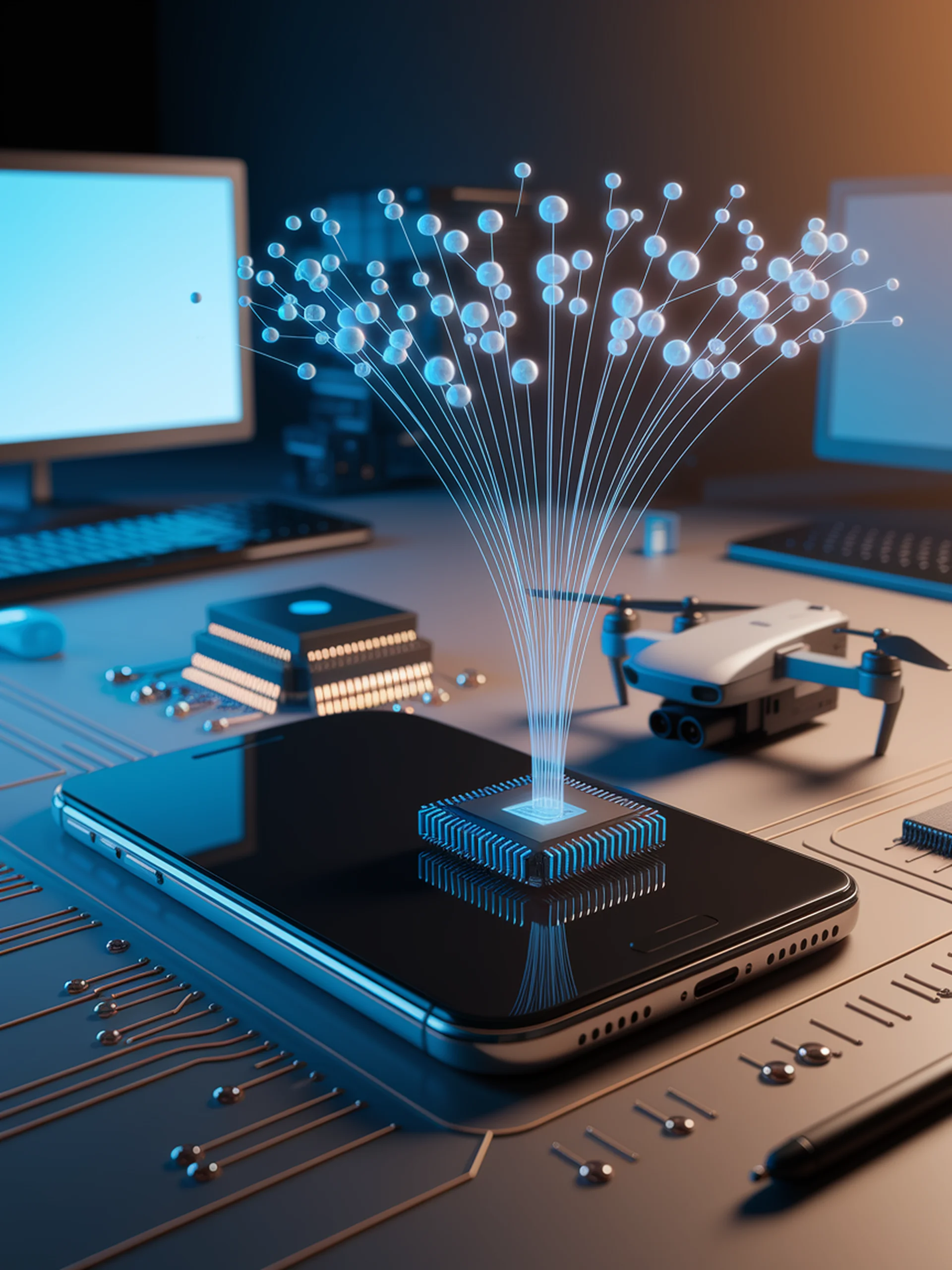
Shrinking AI Giants for Edge Devices
Knowledge Distillation to Deploy Large Models on Resource-Constrained Devices
This research tackles a critical challenge in AI deployment: how to compress large foundation models for use on edge devices without significant performance loss.
- Knowledge distillation techniques transfer knowledge from large "teacher" models to compact "student" models
- Enables deployment of powerful AI capabilities on resource-constrained devices
- Addresses key engineering challenges including runtime efficiency and memory consumption
- Particularly valuable for recent large-scale foundation models and Vision-Language Models
This work provides a comprehensive survey of techniques that allow organizations to bring advanced AI capabilities to mobile devices, IoT sensors, and other edge computing environments—expanding real-world applications while reducing infrastructure costs.