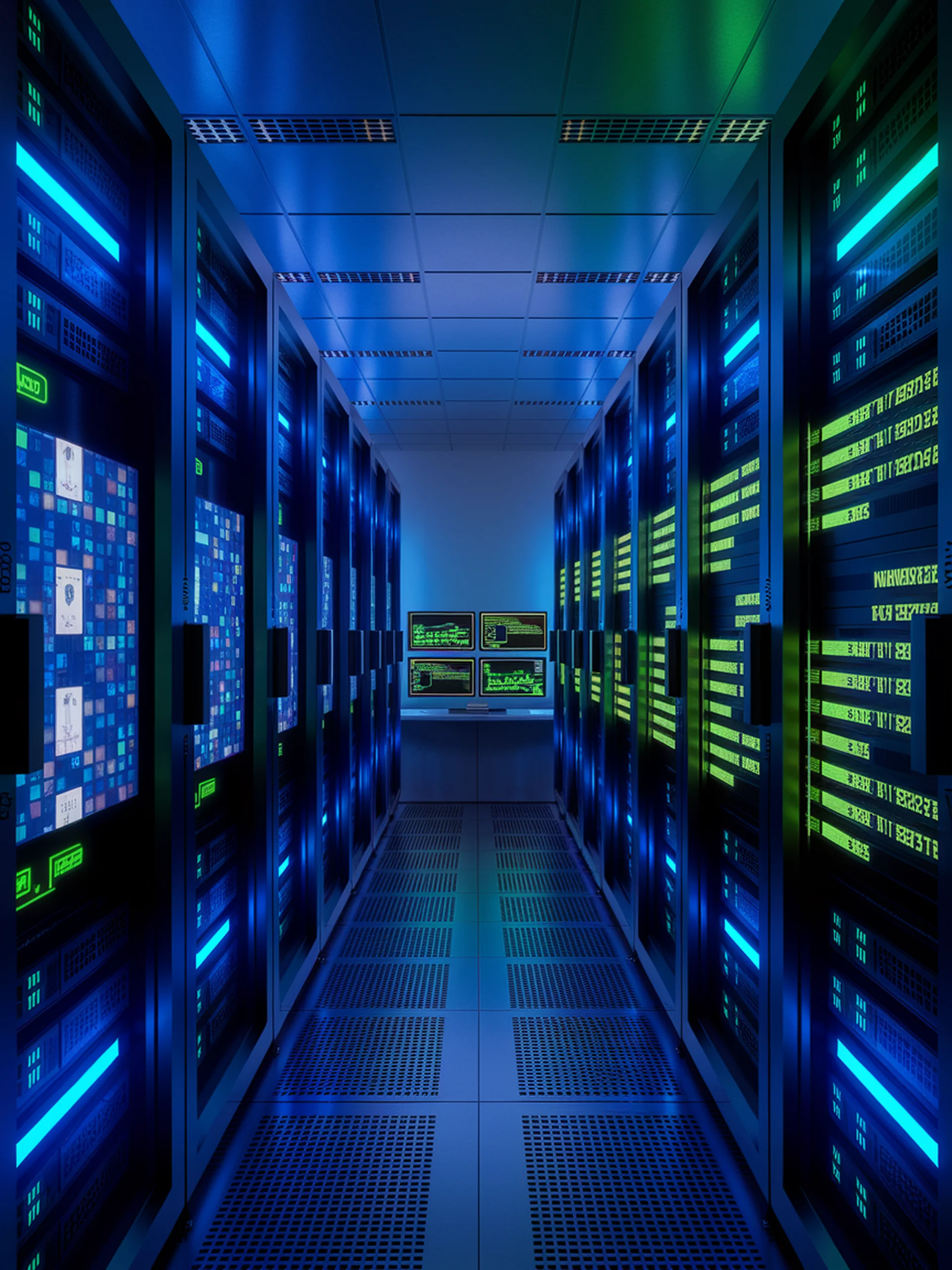
Optimizing LLM and Image Recognition Performance
Efficient task allocation strategies for multi-GPU systems
This research evaluates parallelization techniques for distributed processing of image classification and large language models across multi-GPU systems.
- Compares multiple parallelization methods including simple data parallelism, distributed data parallelism, and fully distributed processing
- Analyzes performance tradeoffs between different hardware and software configurations
- Provides implementation strategies for efficiently scaling ML workloads across multiple GPUs
- Demonstrates how proper task allocation can significantly improve training and inference efficiency
For engineering teams, this research offers practical approaches to maximize computational resources when deploying complex ML models, potentially reducing costs and accelerating development cycles.
Efficient allocation of image recognition and LLM tasks on multi-GPU system