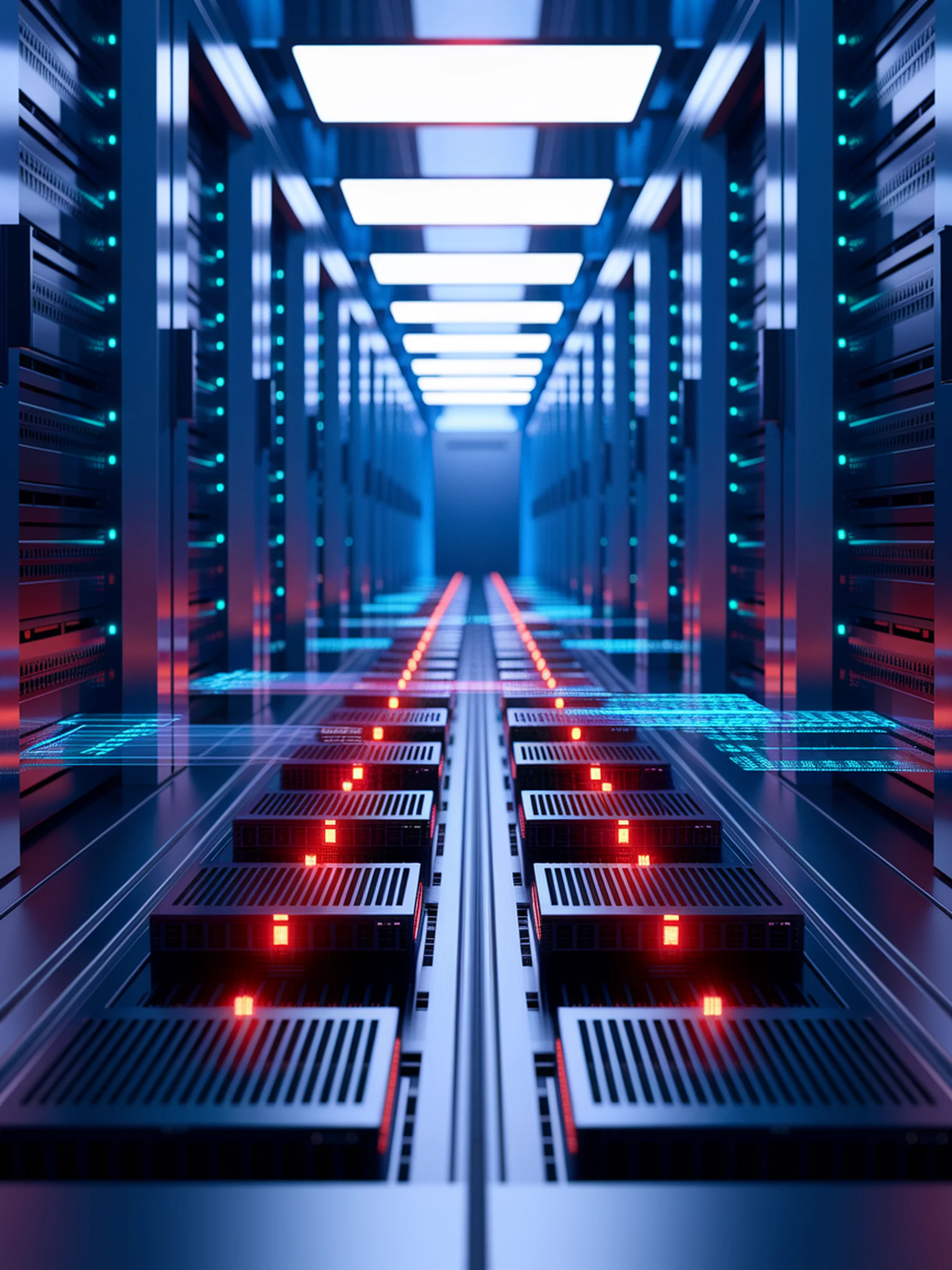
Optimizing Multi-LLM Workflows
Enhancing Efficiency for Complex AI Systems
This research introduces a novel approach for optimizing offline inference efficiency when running multiple large language models on multi-GPU systems.
- Focuses on end-to-end efficiency for applications that utilize multiple LLMs concurrently
- Addresses unique challenges of offline inference scenarios often overlooked in current research
- Develops optimization strategies for parallelism, scheduling, and resource allocation
- Uses sampling and simulation techniques to maximize throughput
This engineering advancement is particularly valuable for enterprise AI systems that need to process large batches of requests across different models efficiently, potentially reducing computation costs and improving system performance.