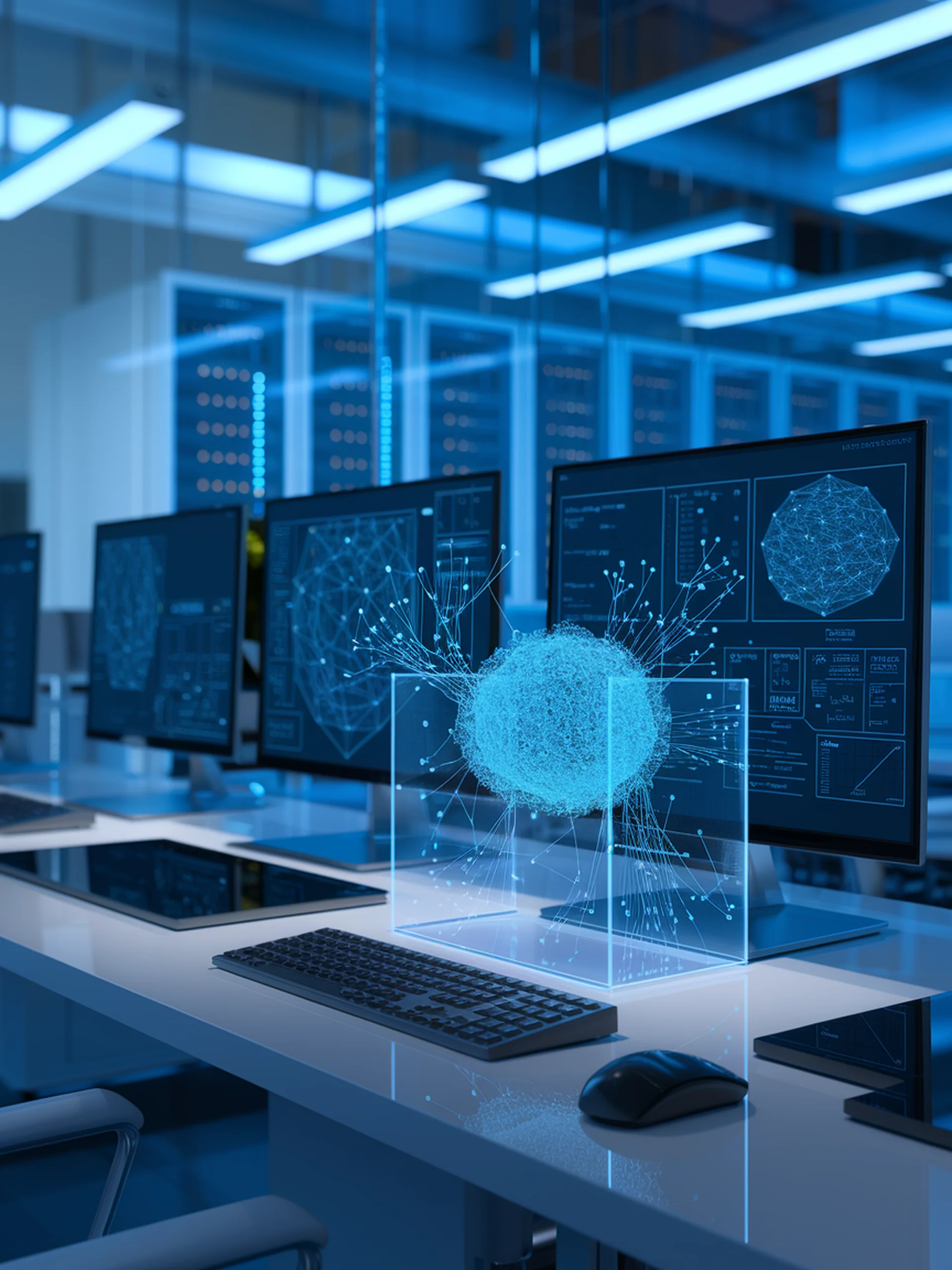
Expanding to Shrink: A New Approach to Model Efficiency
How post-training expansion can improve quantized LLMs
This research introduces a counterintuitive approach to model optimization that increases model size after training to improve performance of quantized models.
- Demonstrates how strategic model expansion can enhance quality in quantized models
- Introduces novel techniques that transform the traditional size-quality trade-off
- Achieves better performance-to-cost ratios than standard quantization methods
- Works effectively across various model architectures and sizes
For engineering teams, this research offers practical pathways to deploy more efficient LLMs without sacrificing quality, potentially reducing inference costs while maintaining model capabilities.