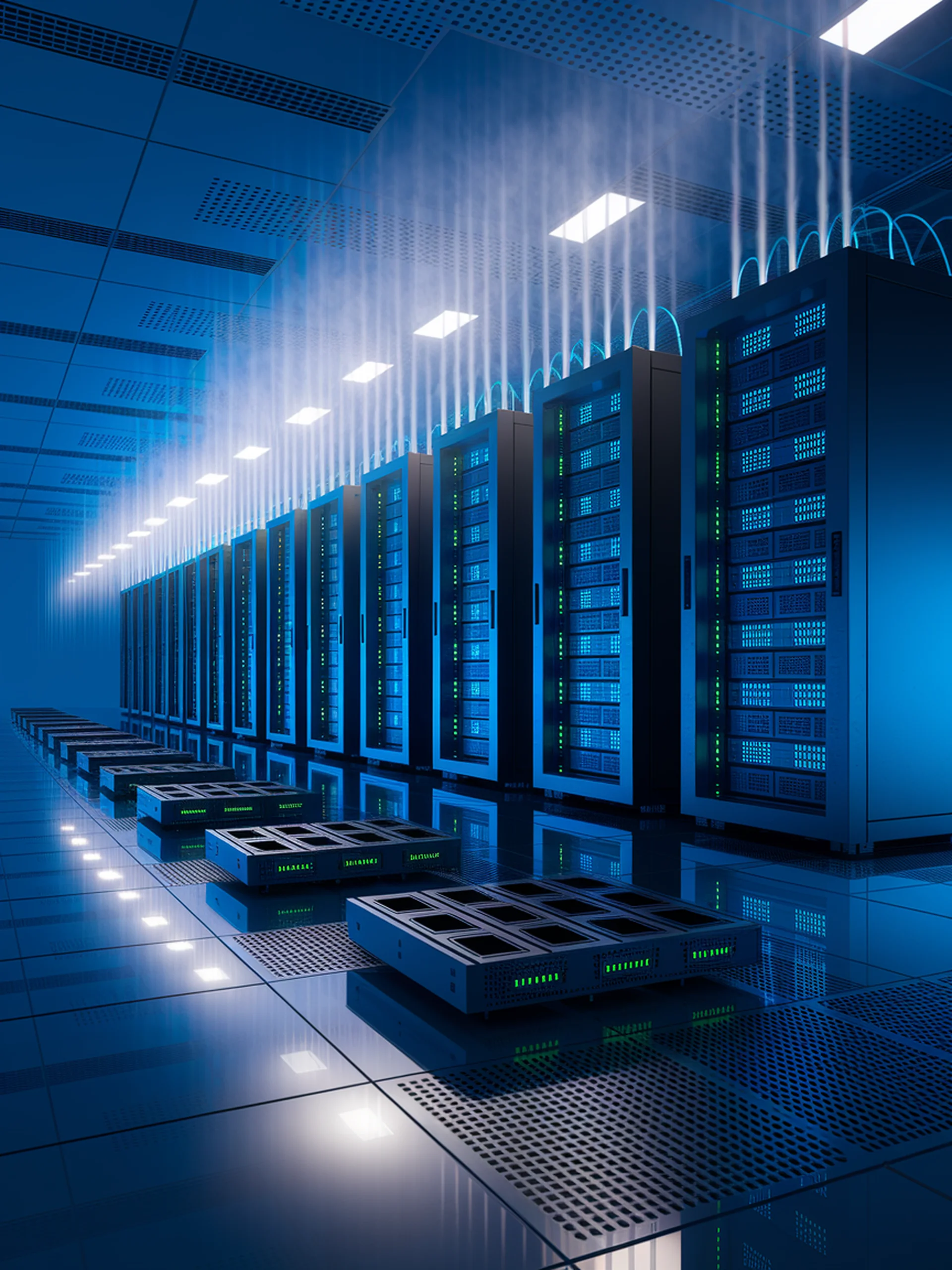
Optimizing LLM Training Efficiency
Memory and Parallelism Co-optimization for Faster LLM Training
Mist introduces a novel approach to distributed LLM training by simultaneously optimizing parallelism strategies and memory management techniques.
- Addresses the complex challenge of finding optimal combinations of parallelism (data, tensor, pipeline) and memory optimization techniques
- Develops a hierarchical search algorithm that efficiently navigates the vast configuration space
- Achieves up to 1.68× speedup over state-of-the-art distributed training systems
- Incorporates overlap-awareness between computation and communication for more accurate performance modeling
This research significantly advances engineering capabilities for training larger models with limited resources, making cutting-edge AI development more accessible and cost-effective for organizations with constrained computing budgets.
Mist: Efficient Distributed Training of Large Language Models via Memory-Parallelism Co-Optimization