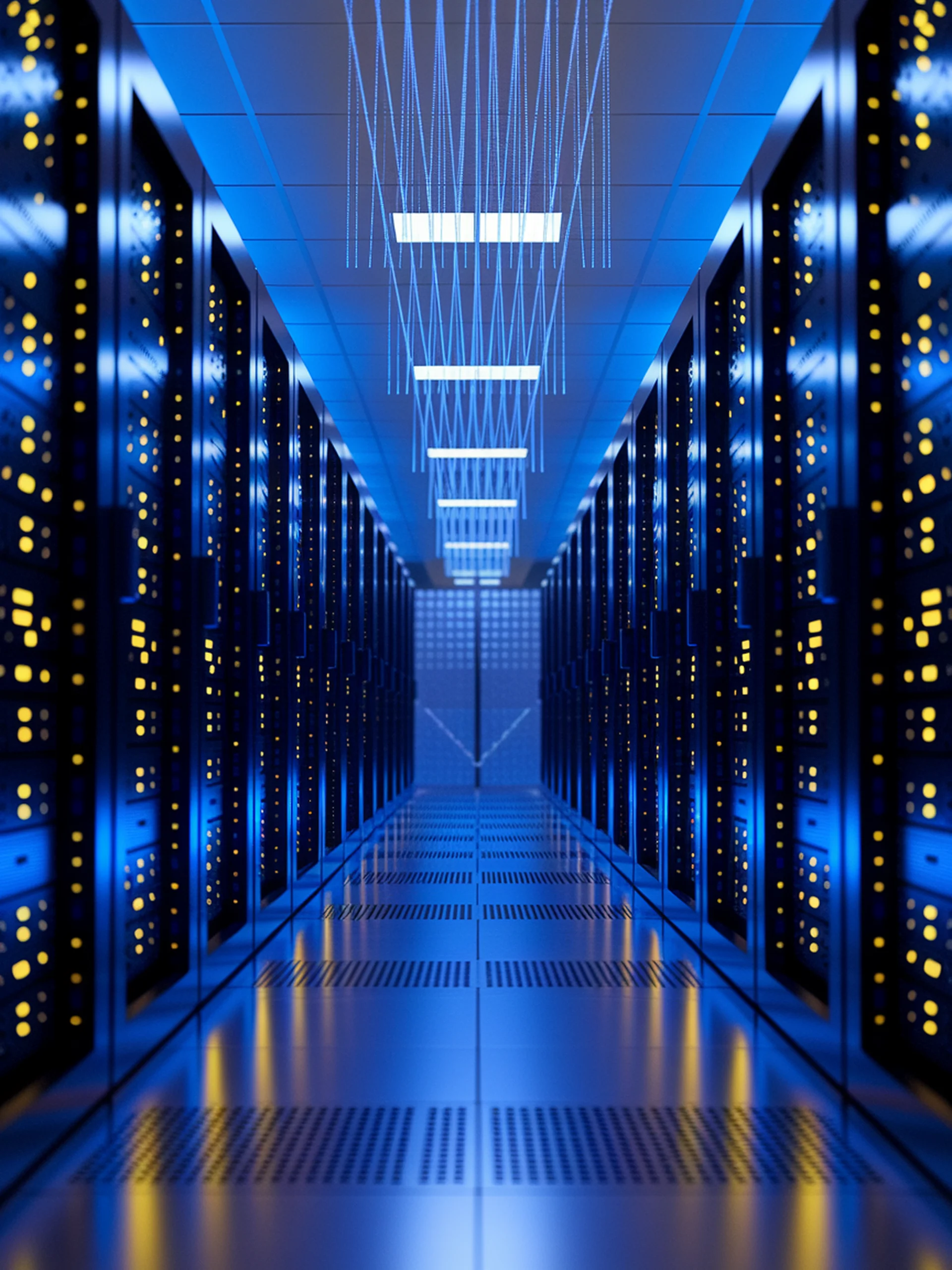
Boosting LLM Performance with Attention Disaggregation
A novel approach to optimize resource utilization in LLM serving systems
This research introduces Attention Disaggregation, a technique that significantly improves throughput for large language model serving by optimizing how computational resources are allocated.
- Addresses the resource underutilization problem in current prefill-decoding disaggregation approaches
- Enhances throughput by 1.8-2.9× compared to state-of-the-art serving systems
- Maintains efficient resource utilization across both compute-intensive (prefill) and memory-intensive (decoding) phases
- Implements a practical system design that prevents performance interference while maximizing hardware efficiency
For engineering teams, this approach offers a practical path to serve more LLM requests using existing hardware resources, potentially reducing infrastructure costs and improving system responsiveness.