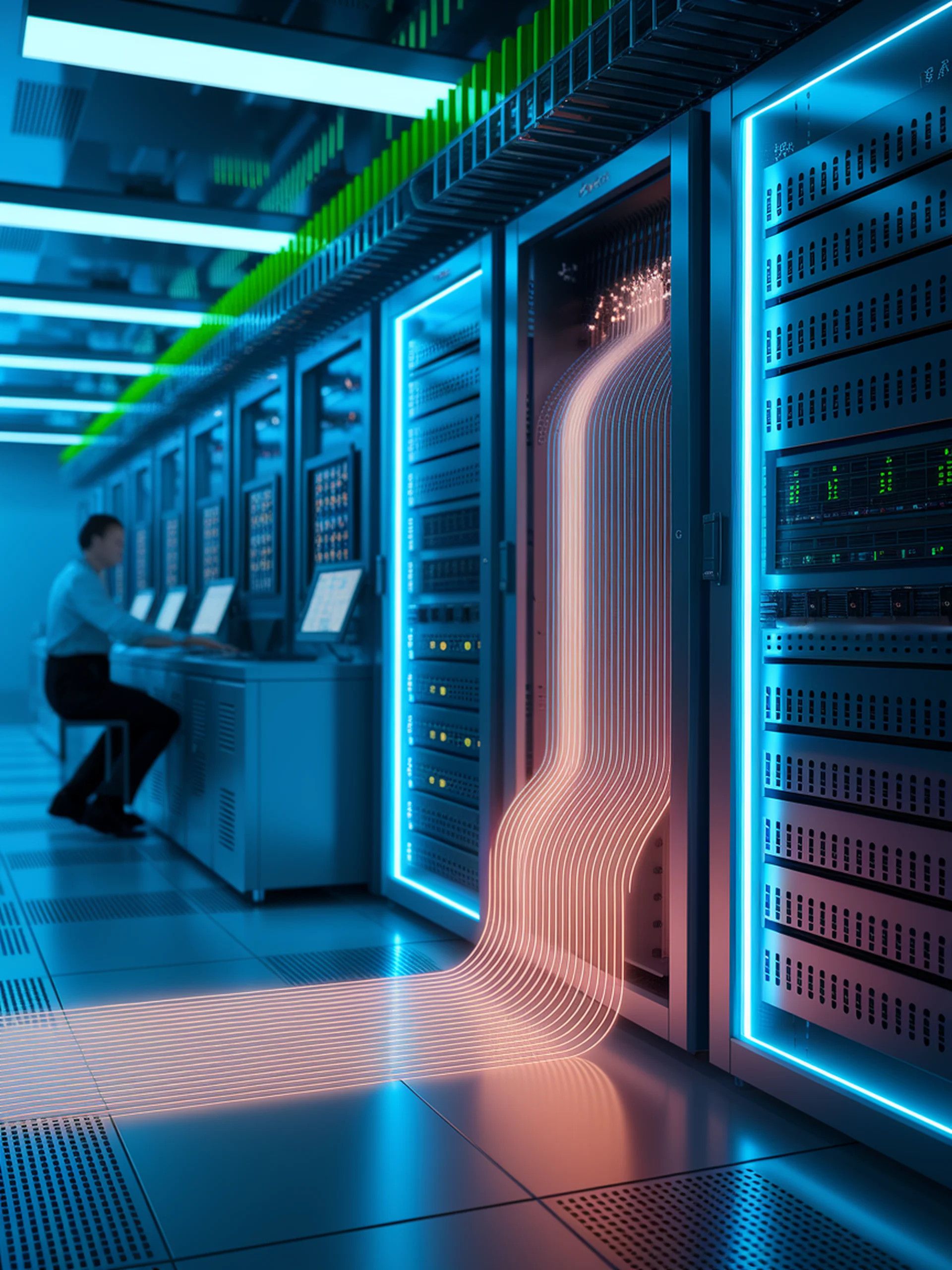
Smart Compression for Next-Gen AI Models
Enhancing MoE Model Efficiency Through Multi-stage Quantization
MoQa introduces a novel approach to compress and accelerate Mix-of-Experts (MoE) language models through specialized quantization techniques.
- Addresses the unique distributions in MoE models, unlike traditional dense model quantization methods
- Implements a multi-stage framework that analyzes both data and model distribution patterns
- Achieves superior compression while maintaining model performance compared to existing approaches
- Provides practical acceleration benefits, making large MoE models more deployable
This research matters for engineering teams deploying large AI systems, enabling more efficient resource utilization and faster inference without sacrificing model capabilities.
MoQa: Rethinking MoE Quantization with Multi-stage Data-model Distribution Awareness