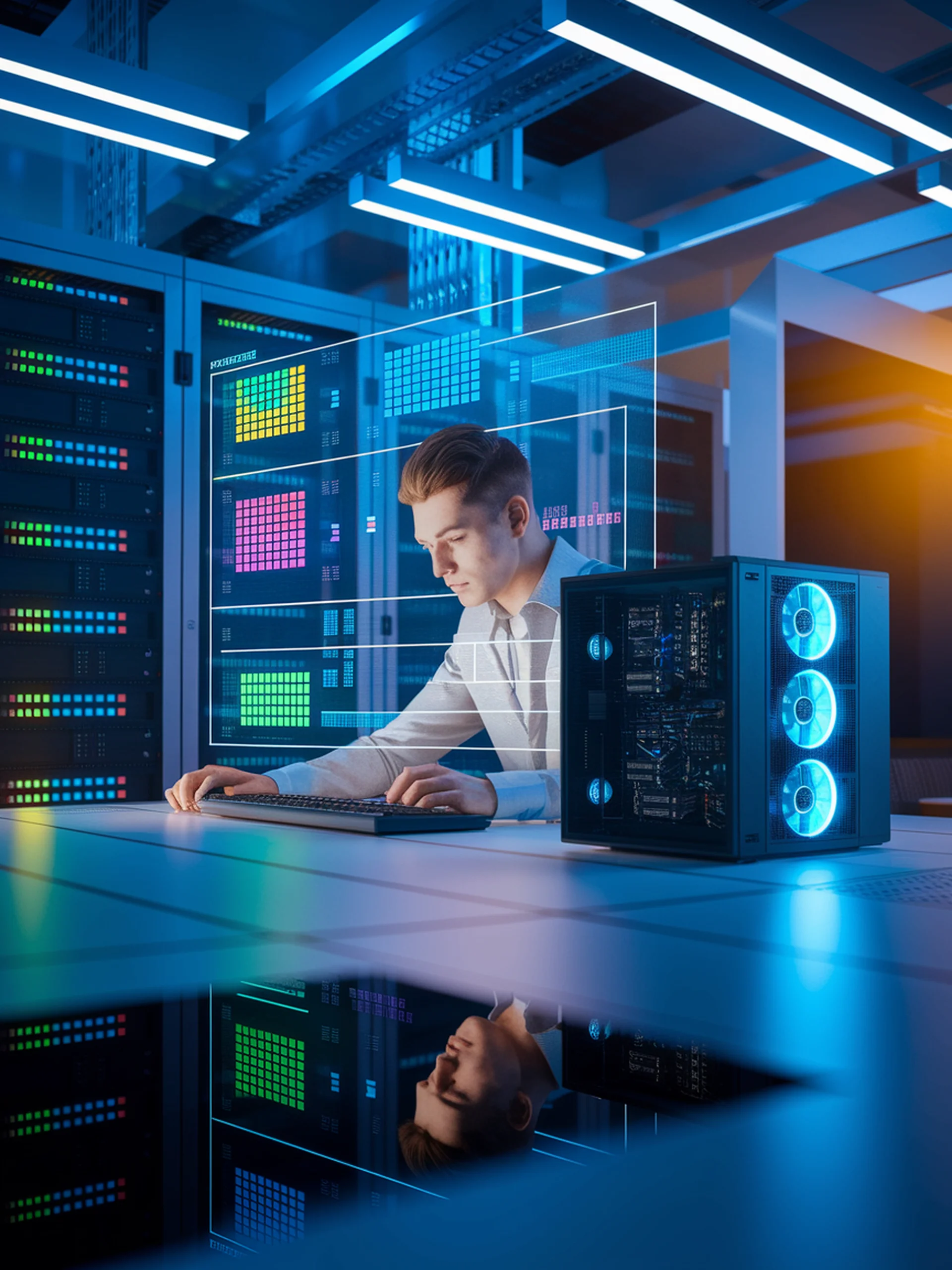
Cocktail: Optimizing LLM Performance for Long Contexts
Chunk-adaptive quantization boosts memory efficiency and inference speed
Cocktail introduces a novel chunk-adaptive mixed-precision quantization approach for LLM inference that dramatically reduces memory usage and latency for long-context scenarios.
- Uses chunk-based quantization instead of token-level approaches for better hardware efficiency
- Achieves up to 3.8× throughput improvement over FP16 on 4096-context inference
- Maintains model quality comparable to FP16 while significantly reducing memory requirements
- Compatible with existing attention optimization techniques for compounded efficiency gains
This research directly addresses a critical engineering challenge: as context lengths grow, traditional methods become prohibitively slow and memory-intensive. Cocktail's approach enables practical deployment of long-context LLMs with existing hardware resources.
Cocktail: Chunk-Adaptive Mixed-Precision Quantization for Long-Context LLM Inference