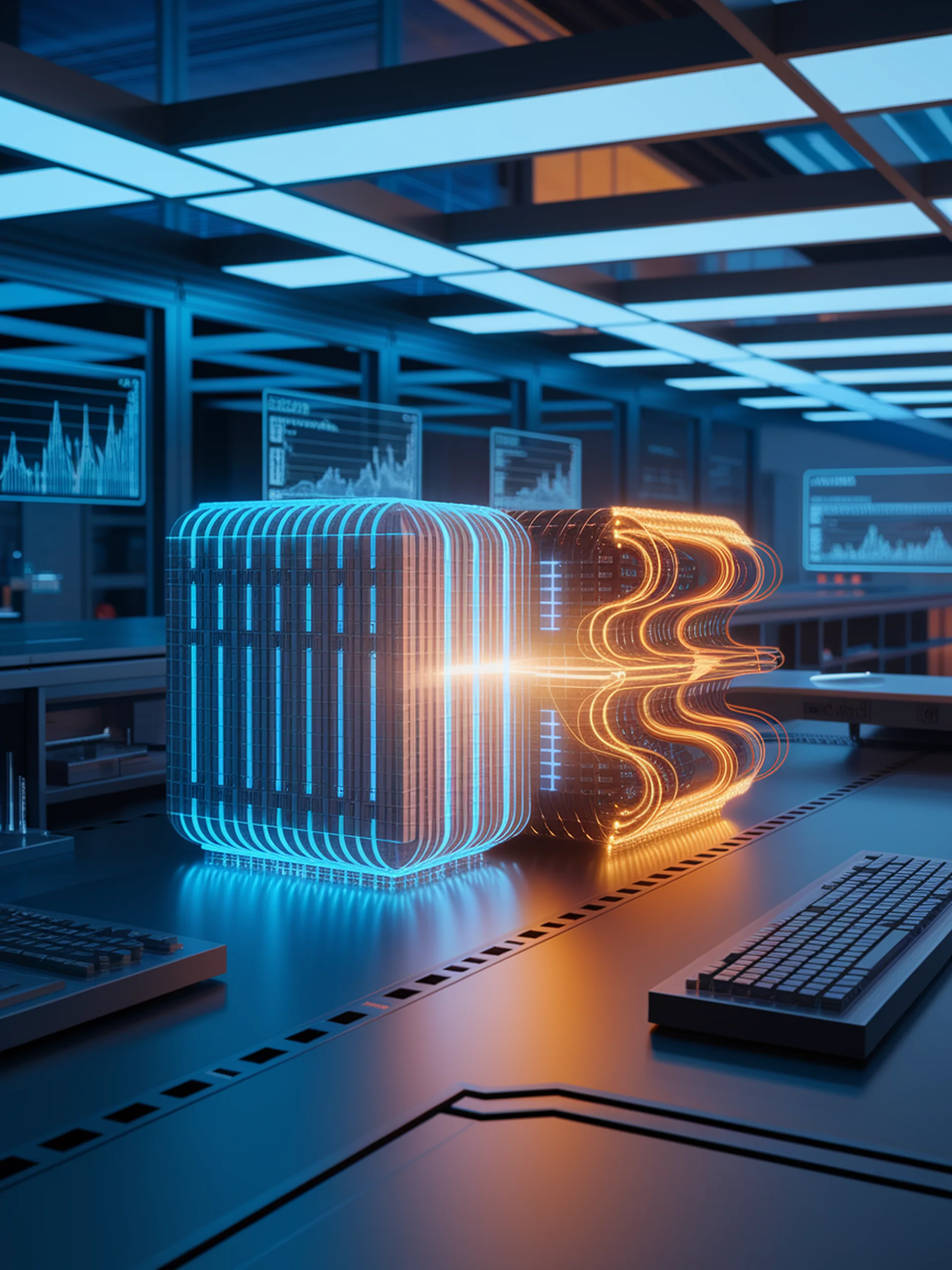
TransMamba: Best of Both Worlds
A hybrid architecture combining Transformer efficiency with Mamba performance
TransMamba addresses the efficiency-performance tradeoff in large language models by unifying Transformer and Mamba architectures through shared parameter matrices.
- Solves key limitations: Overcomes Transformer's quadratic complexity for long sequences while fixing Mamba's contextual learning instability
- Flexible switching: Dynamically leverages strengths of both architectures based on computational needs
- Engineering breakthrough: Creates a unified framework that maintains performance while improving efficiency for long-context processing
- Practical implications: Enables more efficient LLMs that can handle longer sequences without sacrificing performance
TransMamba: Flexibly Switching between Transformer and Mamba