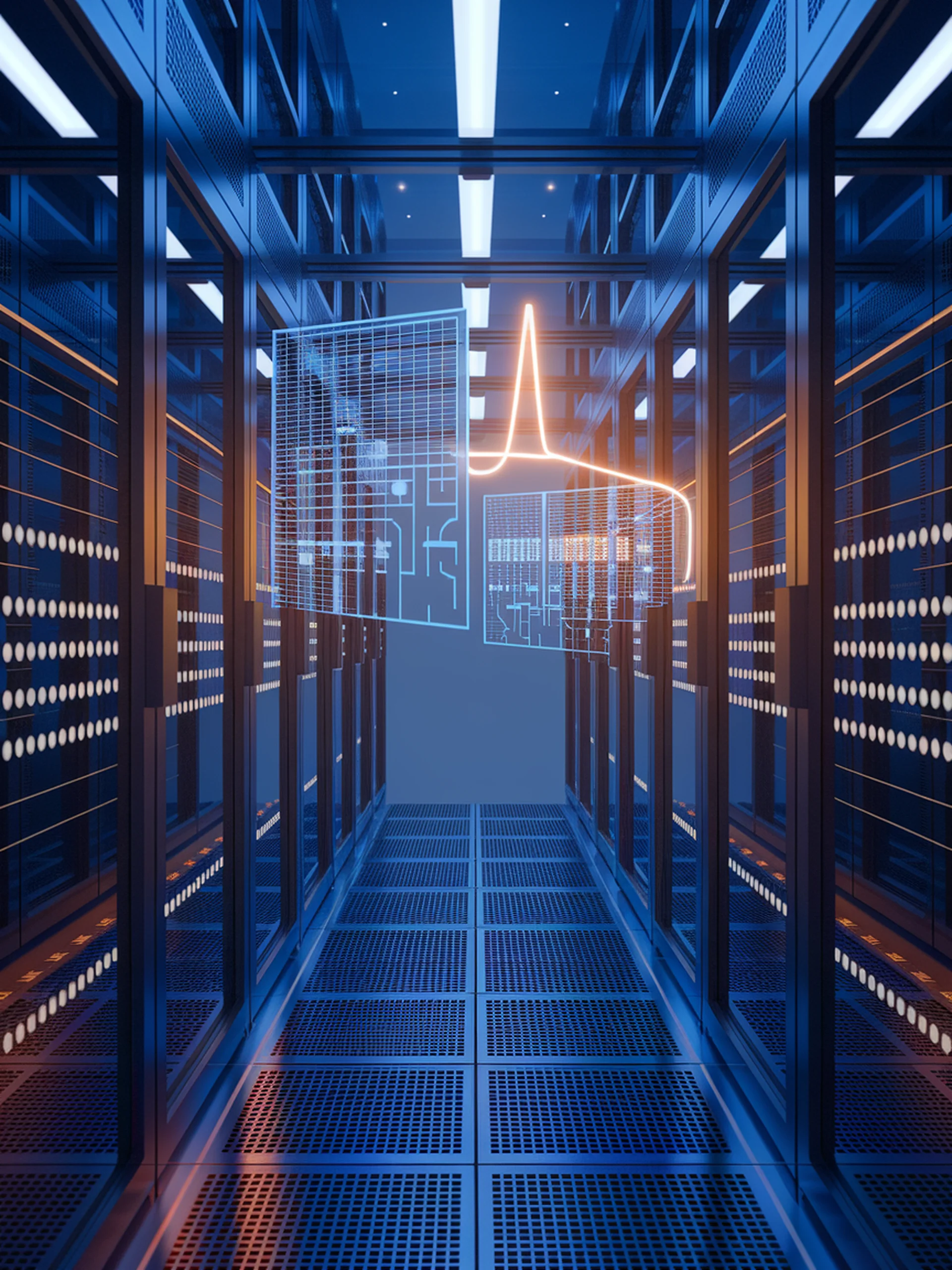
ShortV: Making Multimodal LLMs Faster
Freezing Visual Tokens Where They Don't Matter
ShortV introduces an efficient approach to reduce computational costs in Multimodal Large Language Models by identifying and freezing visual tokens in layers where they contribute minimally.
- Introduces Layer Contribution (LC) metric to quantify each layer's impact on visual and text tokens
- Achieves up to 60% computation reduction with negligible performance impact
- Demonstrates effectiveness across multiple MLLM architectures including LLaVA and MiniGPT-4
- Provides theoretical framework for understanding layer-wise redundancy in MLLMs
This research matters for Engineering by offering practical techniques to deploy more efficient multimodal AI systems with lower computational requirements, making advanced vision-language models more accessible for real-world applications.
ShortV: Efficient Multimodal Large Language Models by Freezing Visual Tokens in Ineffective Layers