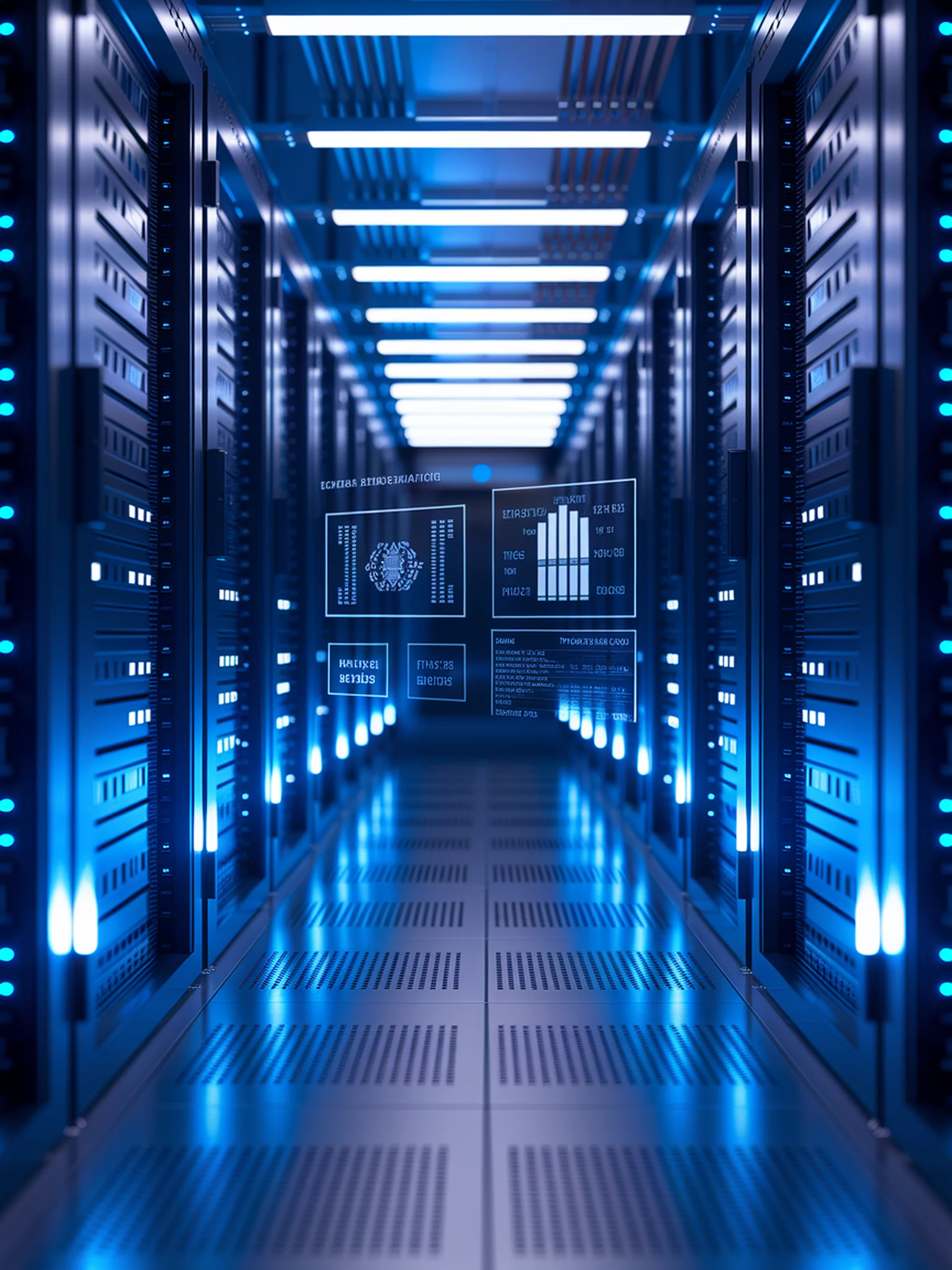
Scaling Up Transformer Training: A Memory-Focused Approach
Optimizing hardware resources for more efficient large model training
This research presents a novel approach for training massive transformer models by focusing on memory usage and network bandwidth optimization rather than computational power alone.
- Fully Sharded Data Parallel technique minimizes memory footprint while maximizing training speed
- Addresses key bottlenecks in distributed training of large language models
- Demonstrates that memory and bandwidth considerations are more critical than pure computational capacity
- Provides practical engineering solutions for scaling transformer model training
For engineering teams building AI infrastructure, this research offers valuable insights on how to design more efficient training environments for large-scale models, potentially reducing hardware costs while enabling the development of more powerful AI systems.
Memory and Bandwidth are All You Need for Fully Sharded Data Parallel