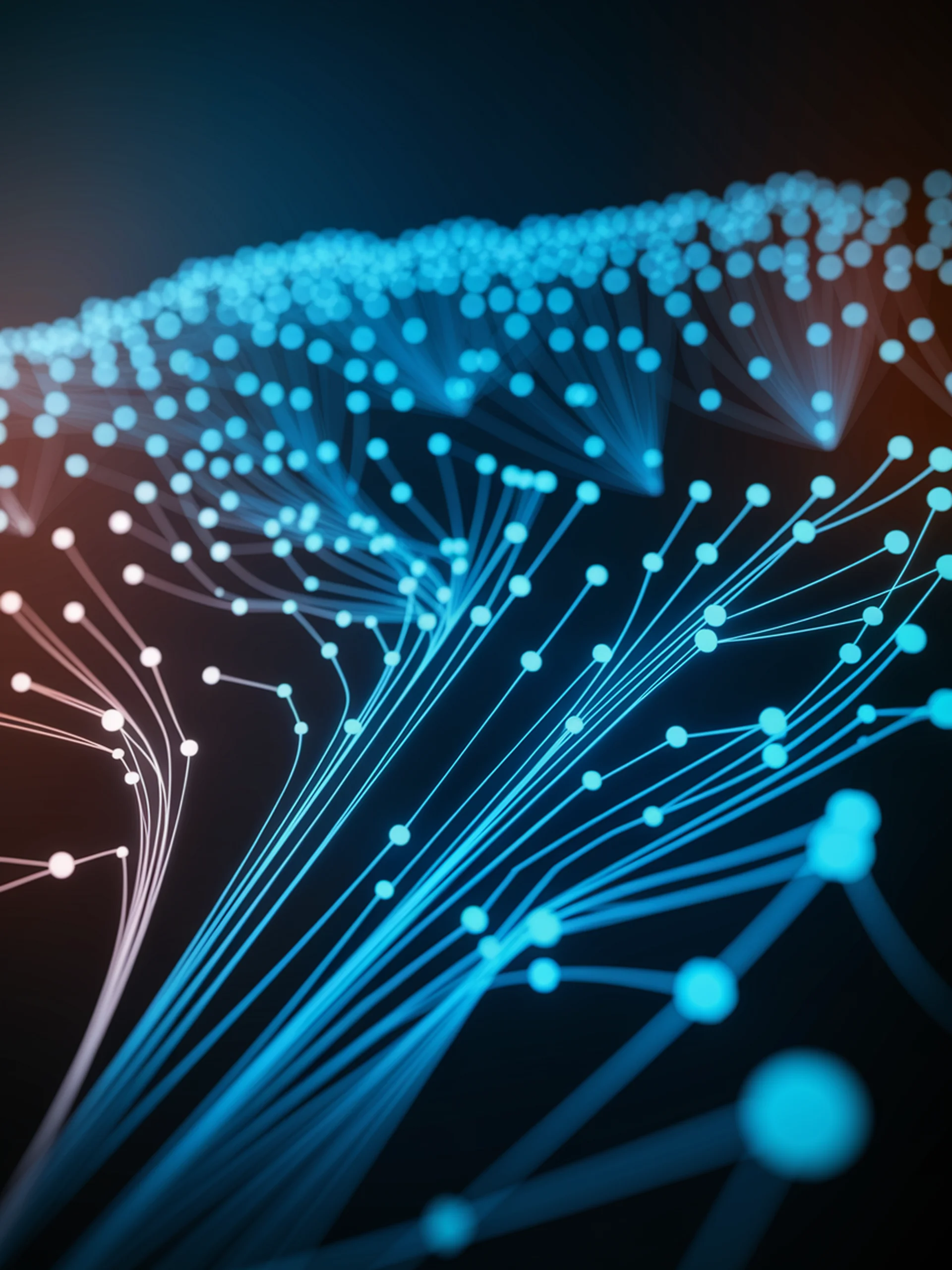
Accelerating LLMs with Smart Token Pruning
Using Saliency Analysis to Reduce Computational Complexity
SDTP addresses the computational bottleneck of LLMs when processing long sequences by intelligently identifying and removing less important tokens during inference.
- Leverages feature attribution theory to determine token importance
- Implements a dynamic pruning strategy that adapts throughout the inference process
- Significantly reduces computational costs while maintaining output quality
- Enables more efficient processing of long-context scenarios
This engineering innovation makes LLMs more practical for real-world applications by addressing one of their fundamental limitations: the quadratic complexity of attention mechanisms when handling long inputs.
Saliency-driven Dynamic Token Pruning for Large Language Models