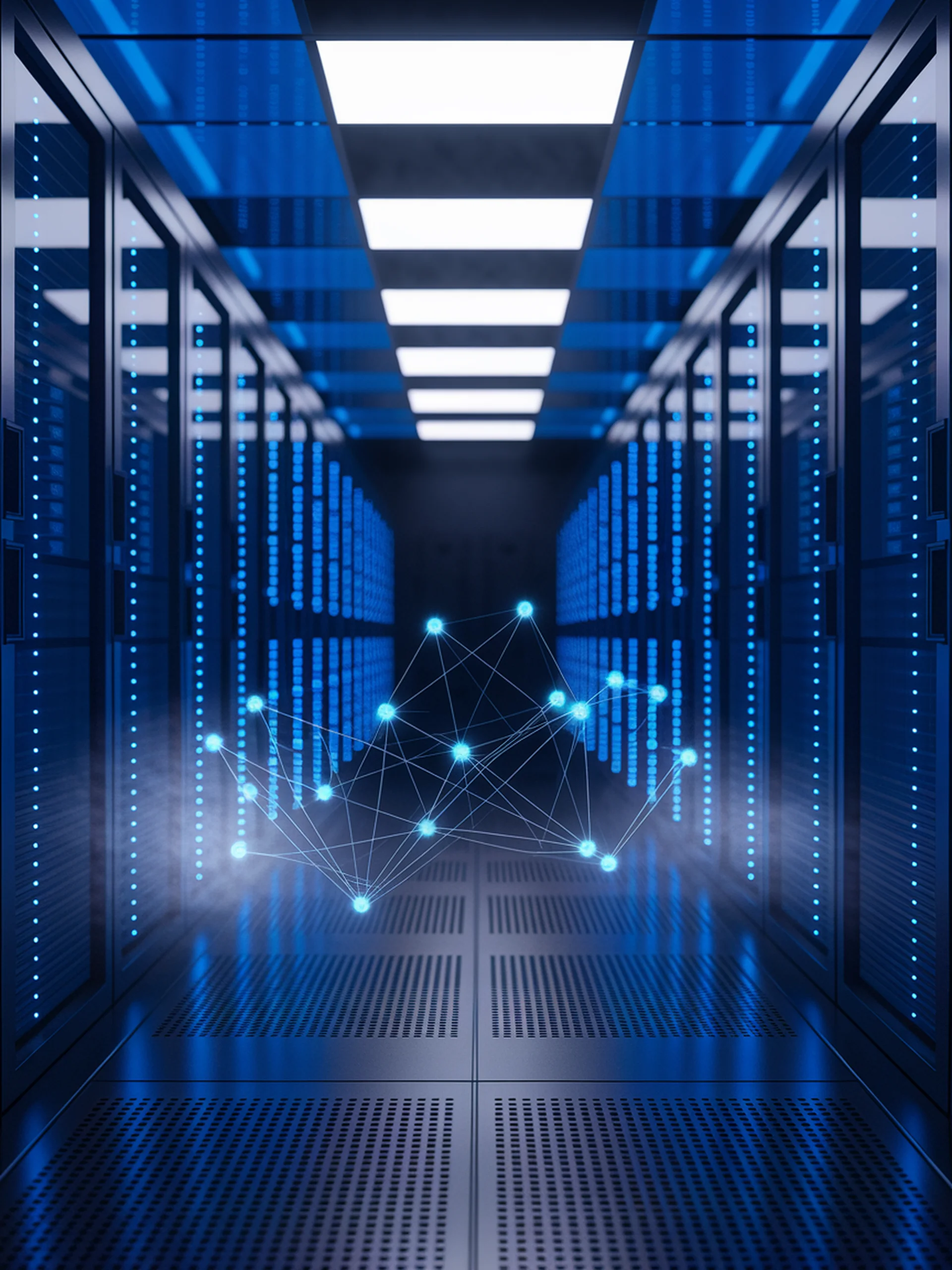
Smart KV Cache Optimization
Using lag-relative information to identify important tokens
LagKV introduces an efficient approach to reduce KV cache size in large language models without compromising performance.
- Uses lag-relative information to determine token importance without relying on attention weights
- Achieves up to 95% KV cache reduction with minimal accuracy loss
- Requires no modification to existing inference infrastructure
- Demonstrates effectiveness across various long-context tasks including retrieval and reasoning
This innovation addresses a critical engineering challenge in LLM deployment, enabling more efficient inference with longer contexts while maintaining performance integrity.
LagKV: Lag-Relative Information of the KV Cache Tells Which Tokens Are Important