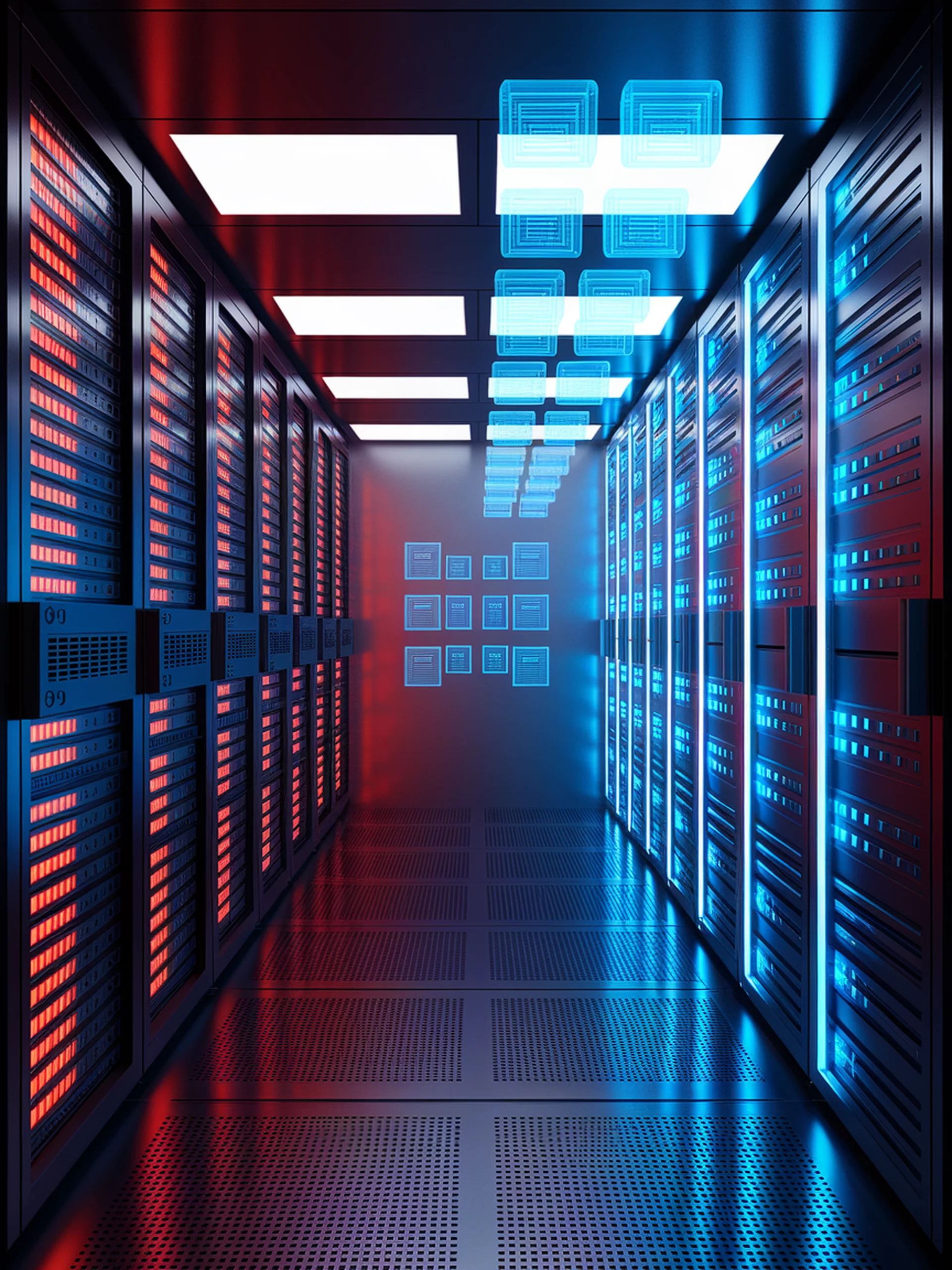
Bridging the Gap: Encoder-Decoder Gemma
Converting decoder-only LLMs for better efficiency-quality balance
This research demonstrates how to adapt decoder-only LLMs to encoder-decoder architecture for improved inference efficiency while maintaining quality.
- Achieves 32x faster inference with comparable performance metrics
- Introduces techniques for effective parameter initialization and optimization
- Develops novel pretraining objectives specifically for adaptation
- Creates a path to leverage strengths from both model architectures
For engineering teams, this approach offers a practical solution to deploy high-quality LLMs in resource-constrained environments where inference speed matters, without sacrificing core capabilities.
Original Paper: Encoder-Decoder Gemma: Improving the Quality-Efficiency Trade-Off via Adaptation