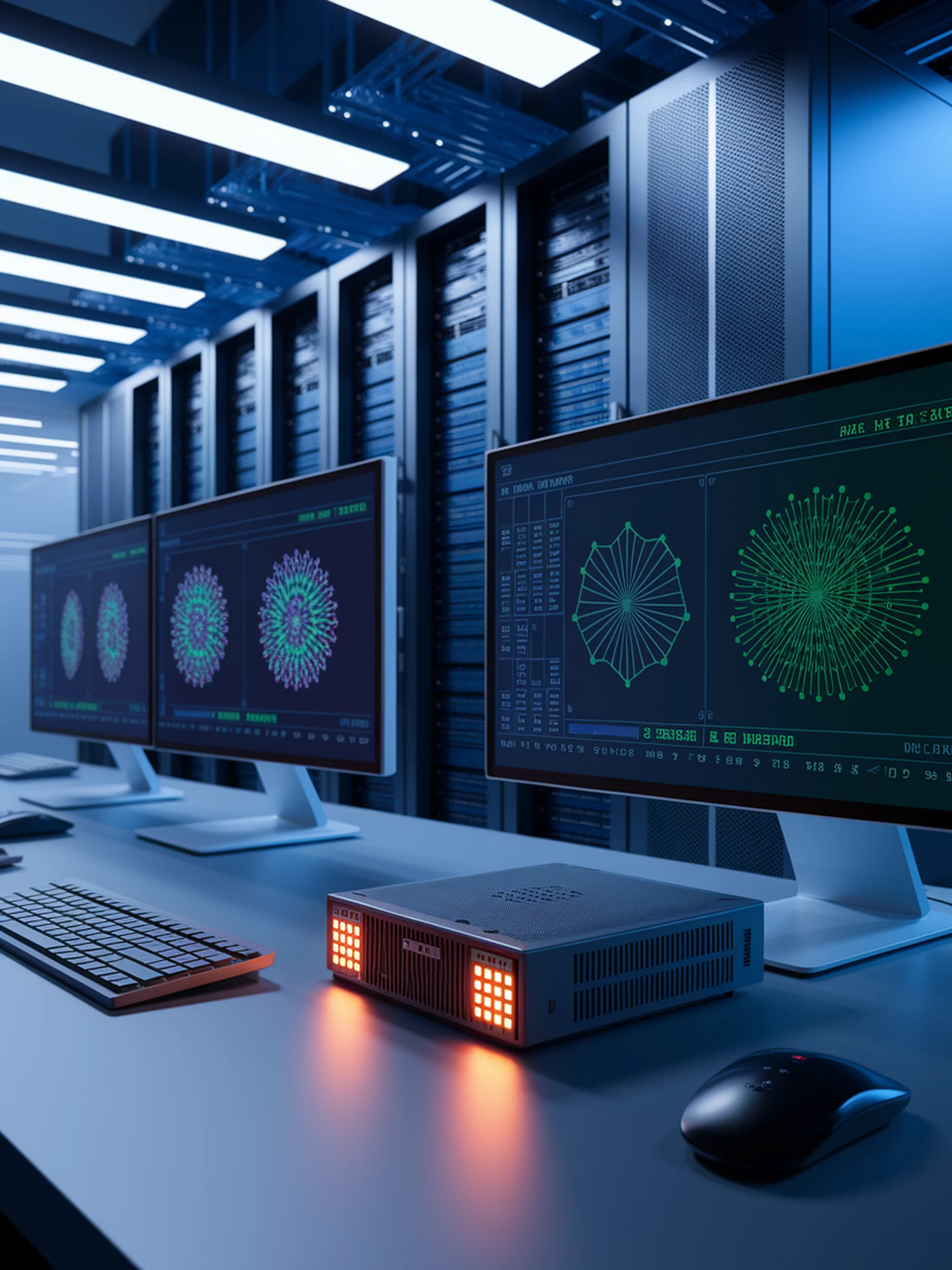
Smarter AI, Smaller Footprint
Strategic Expert Pruning for More Efficient Language Models
This research introduces Cluster-Driven Expert Pruning (CDEP), a novel approach that reduces the size of large language models while preserving performance.
- Addresses the massive parameter footprint challenge of Mixture-of-Experts (MoE) models
- Leverages expert clustering to identify and eliminate redundant components
- Achieves up to 25.3% parameter reduction with minimal performance degradation
- Demonstrates that strategic pruning outperforms random expert removal
For engineering teams, this breakthrough enables more resource-efficient deployment of advanced language models in production environments, potentially reducing infrastructure costs while maintaining model capabilities.
Cluster-Driven Expert Pruning for Mixture-of-Experts Large Language Models