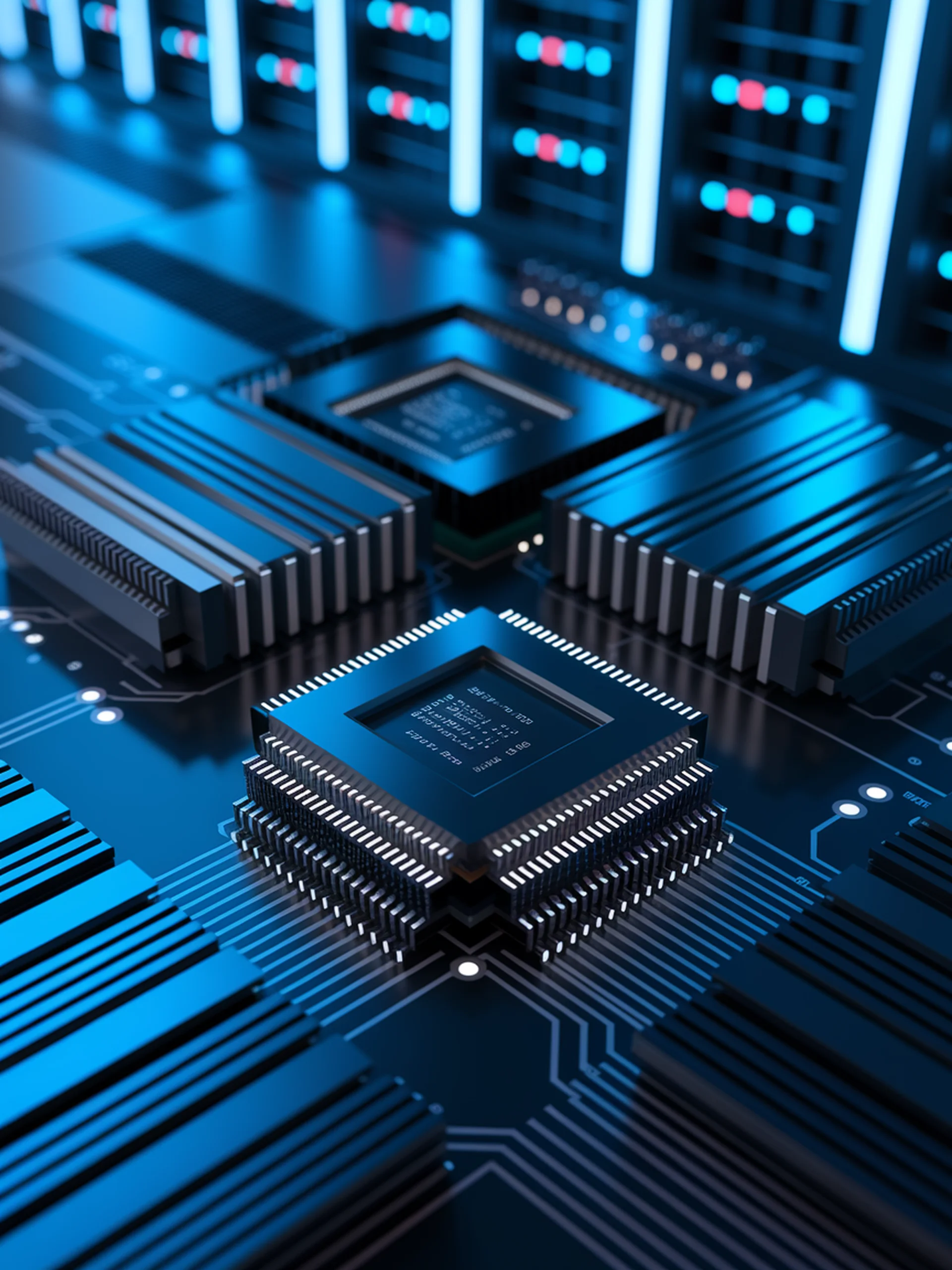
Efficient LLM Inference Through Smart Quantization
A novel approach combining low-rank decomposition with quantization-aware training
This research introduces DL-QAT (Weight-Decomposed Low-Rank Quantization-Aware Training), a technique that significantly improves LLM inference efficiency while maintaining performance.
- Combines the accuracy benefits of QAT with reduced computational requirements
- Uses weight decomposition to make quantization more effective at low-bit precision
- Enables practical deployment of efficiently quantized LLMs for downstream tasks
- Addresses critical inference bottlenecks in large language model deployment
Engineering impact: DL-QAT makes high-performance LLMs more accessible by reducing computational costs and memory requirements during inference, enabling deployment on more constrained hardware platforms.
DL-QAT: Weight-Decomposed Low-Rank Quantization-Aware Training for Large Language Models