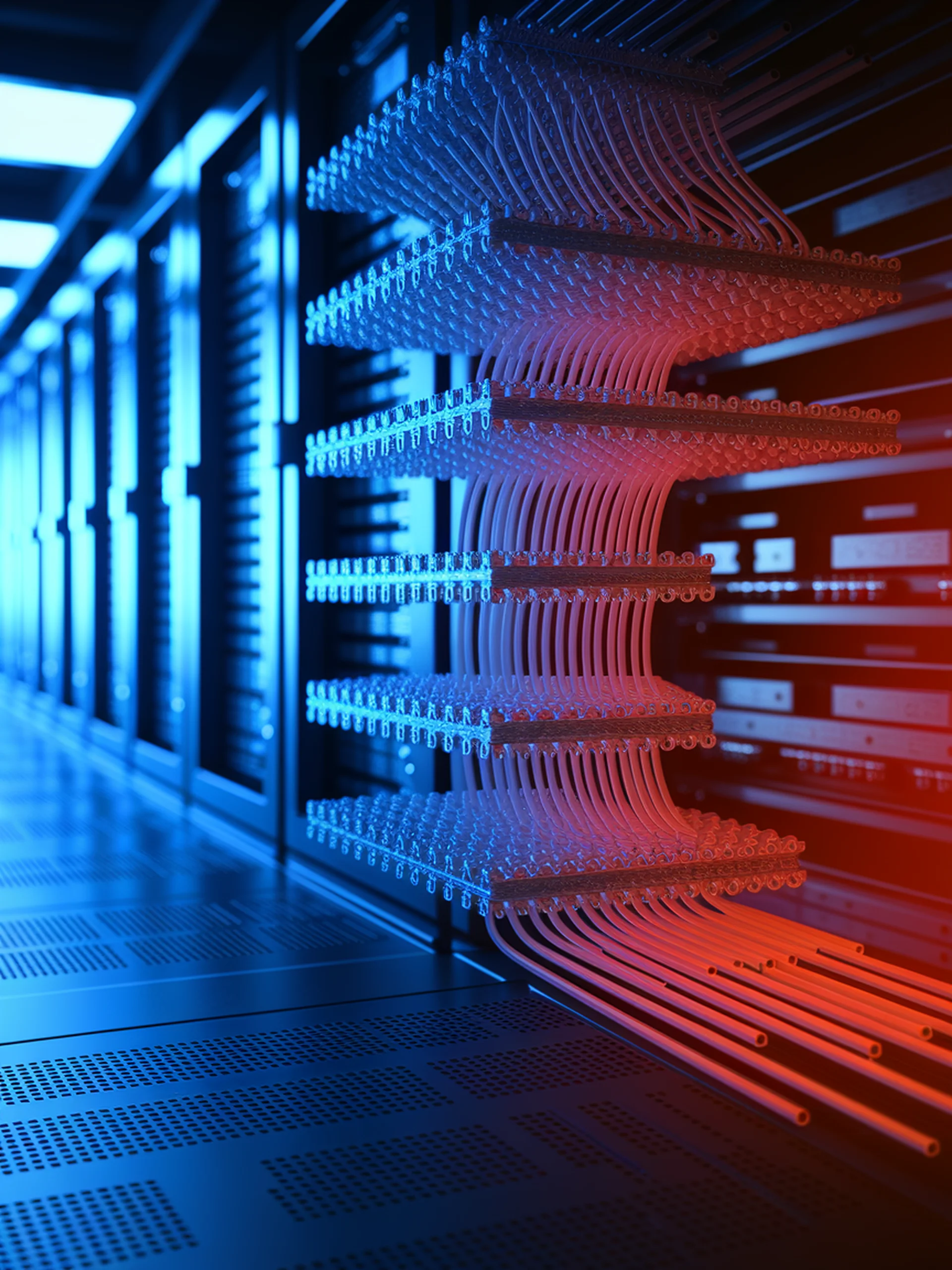
Solving Quantization Error Cascades in LLMs
Addressing the Critical Bottleneck in Model Compression
This research identifies and addresses a fundamental limitation in layer-wise post-training quantization: the accumulation of quantization errors across model layers that significantly degrades performance.
- Reveals error propagation as the key bottleneck in existing quantization methods
- Introduces a new framework for quantifying and mitigating error accumulation
- Demonstrates particular benefits in low-bit compression scenarios
- Enables more efficient deployment of large language models with minimal performance loss
This engineering breakthrough matters because it enables more effective compression of LLMs for deployment on resource-constrained devices without requiring expensive retraining, potentially democratizing access to advanced AI capabilities.
Original Paper: Quantization Error Propagation: Revisiting Layer-Wise Post-Training Quantization