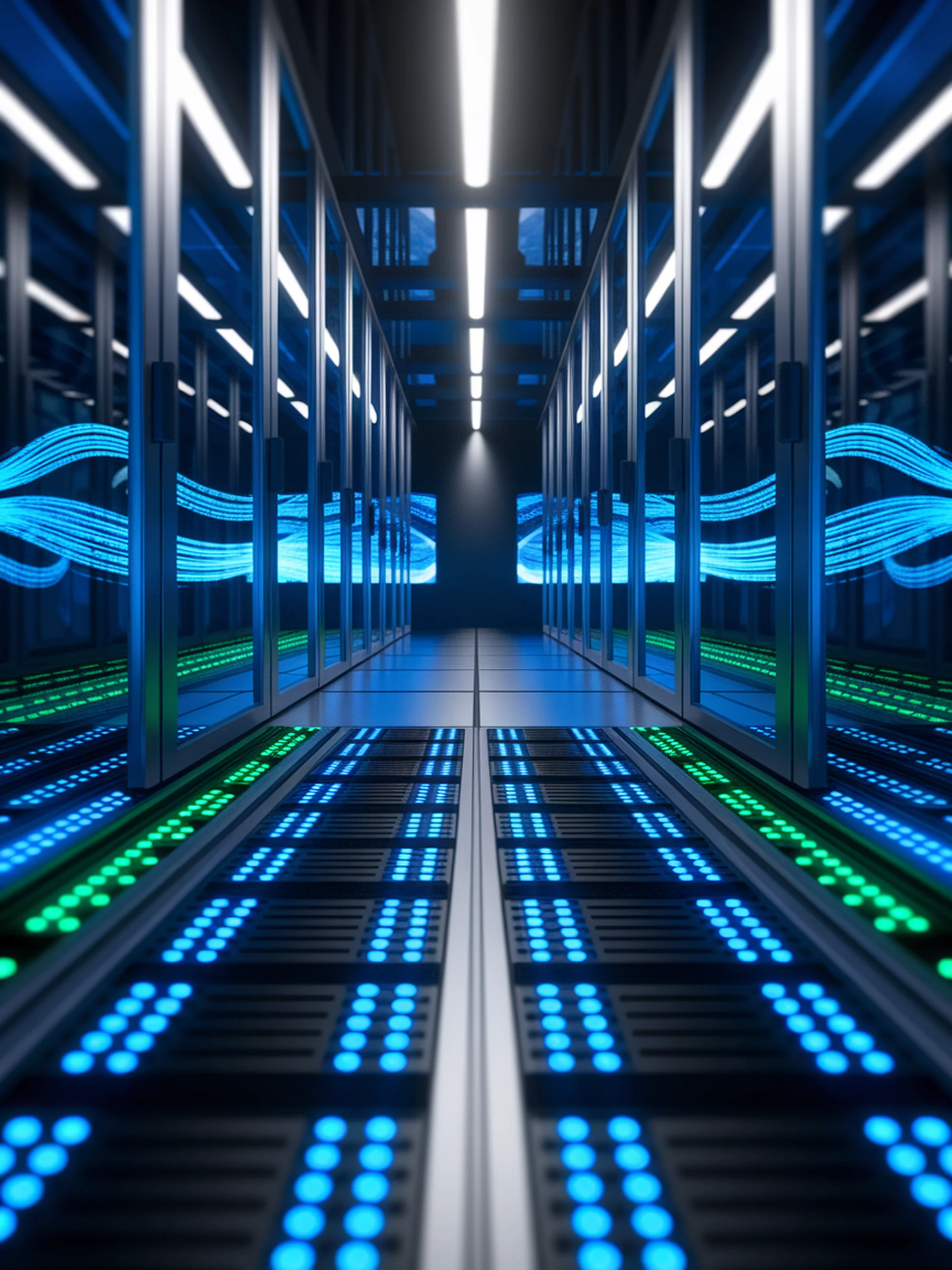
Optimizing LLM Memory: The KeepKV Approach
Achieving efficient inference without sacrificing output quality
KeepKV introduces a novel compression technique for the key-value cache in Large Language Models that eliminates output perturbations while maintaining efficiency.
- Addresses the growing memory bottleneck in LLM inference by optimizing KV cache usage
- Preserves critical information that other compression methods typically lose
- Prevents the hallucinations commonly seen with traditional eviction-based approaches
- Demonstrates superior engineering efficiency compared to existing merging-based strategies
This research matters because it enables more efficient deployment of large language models in resource-constrained environments without compromising output quality, potentially reducing infrastructure costs and energy consumption for AI applications.