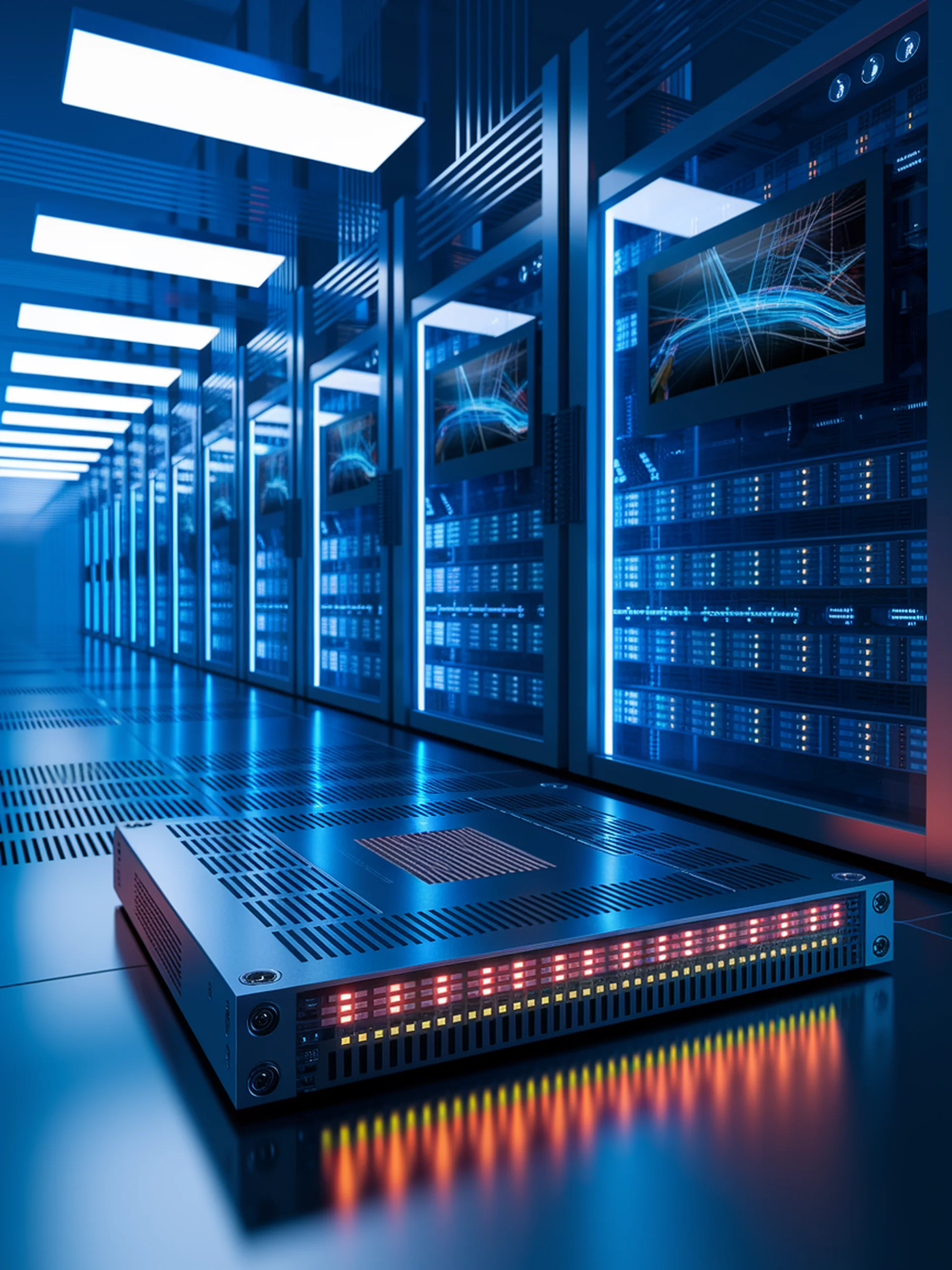
Accelerating Video LLMs with Dynamic Token Compression
Solving the efficiency bottleneck in video processing models
DyCoke introduces a novel dynamic compression approach for video Large Language Models that adapts token selection based on decoding context, significantly improving inference speed and reducing memory usage.
- Achieves up to 2.7× speedup with minimal performance degradation
- Dynamically retains only the most relevant visual tokens at each decoding step
- Reduces memory requirements by over 70% in some configurations
- Demonstrates effectiveness across multiple video understanding benchmarks
This innovation addresses a critical engineering challenge in deploying video AI systems at scale, enabling more efficient real-time video understanding applications in resource-constrained environments.
DyCoke: Dynamic Compression of Tokens for Fast Video Large Language Models