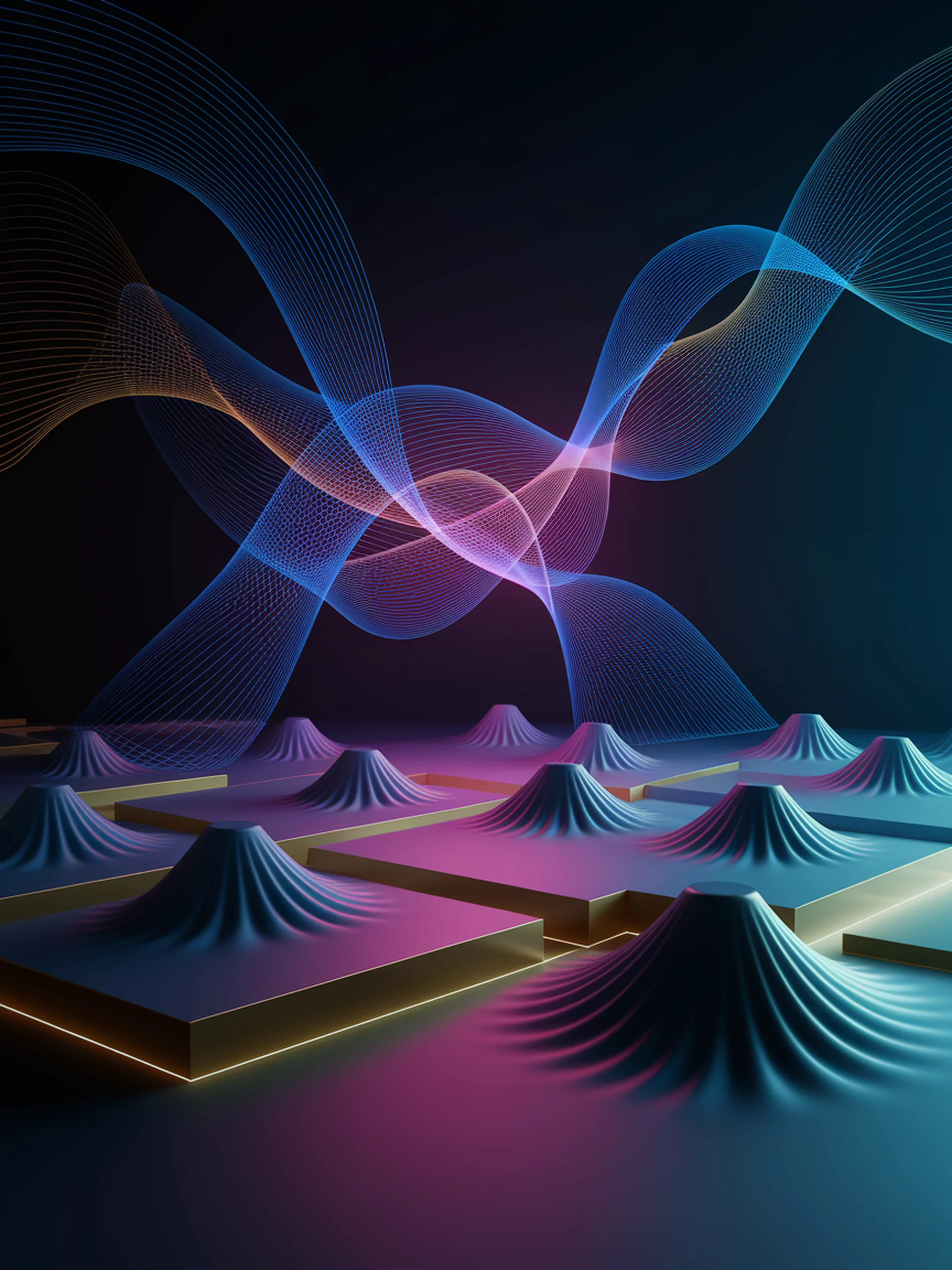
Balancing Multiple Objectives in Deep Learning
Advanced gradient-based methods for handling competing priorities
This research introduces enhanced gradient-based multi-objective optimization (MOO) techniques that enable deep learning systems to effectively balance competing objectives.
- Delivers flexible solution types from single balanced outcomes to complete Pareto sets
- Provides mathematical frameworks for understanding trade-offs between objectives
- Demonstrates practical applications across multi-task learning scenarios
- Establishes foundations for more reliable and versatile AI systems
For engineering teams, this research offers crucial algorithms to build AI systems that can simultaneously optimize for multiple requirements (e.g., accuracy, efficiency, fairness) without manual trial-and-error tuning.
Gradient-Based Multi-Objective Deep Learning: Algorithms, Theories, Applications, and Beyond