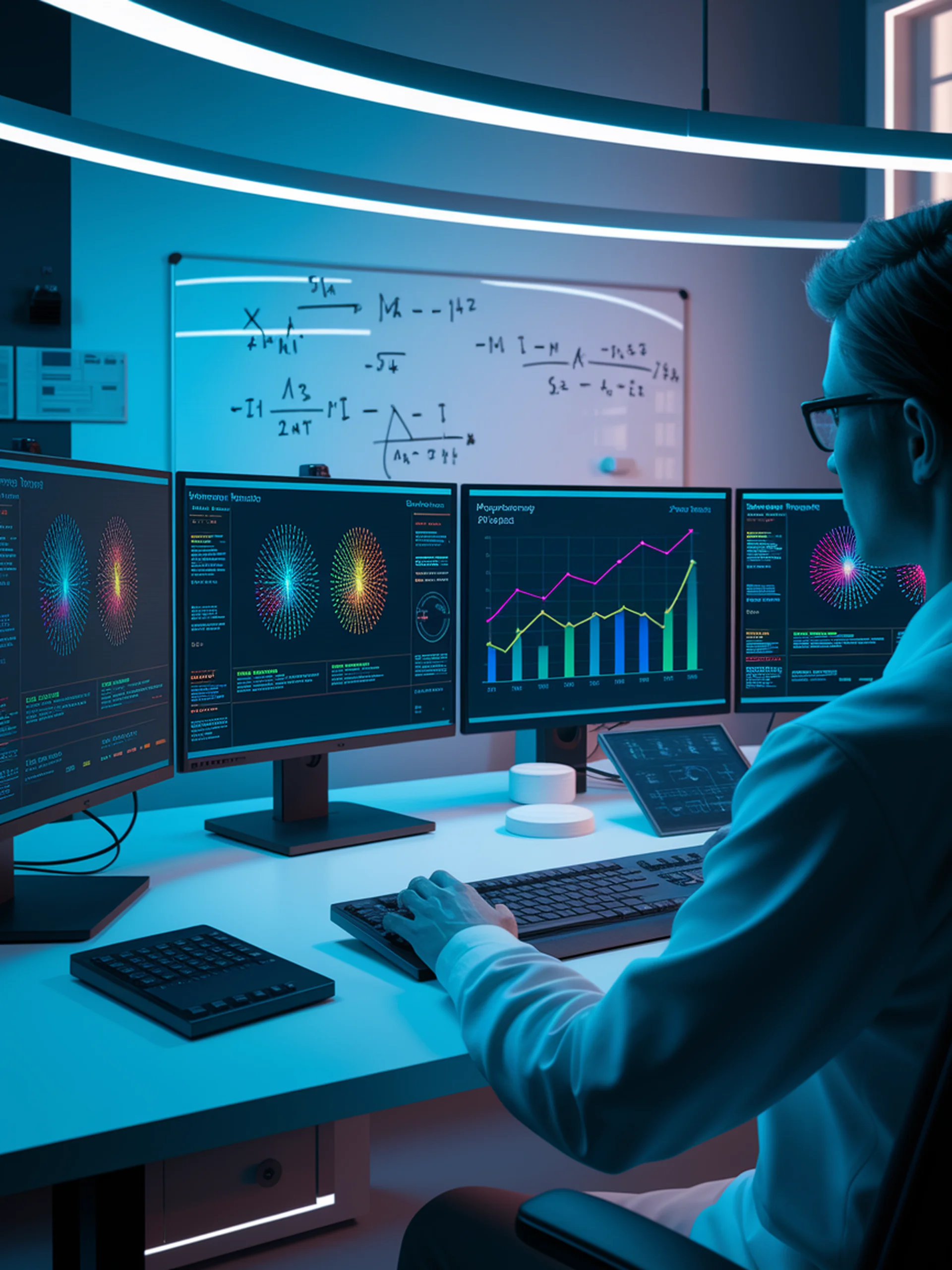
Making RLHF More Efficient
Breakthrough in scaling reinforcement learning from human feedback
This research introduces PbE: Preference-based Exploration, a novel algorithm that dramatically improves the sample efficiency of RLHF for large language model alignment.
- Overcomes the exponential scaling problem (exp(R_max)) that plagues current RLHF methods
- Achieves nearly optimal sample complexity that scales only with the reward function's effective dimension
- Demonstrated superior performance over existing approaches in practical experiments
- Enables more efficient training of aligned AI systems with significantly fewer human preference samples
For security teams, this advancement means more robust AI alignment capabilities with fewer resources, reducing potential safety risks in deployed language models while improving efficiency.
Original Paper: Avoiding exp(R_max) scaling in RLHF through Preference-based Exploration