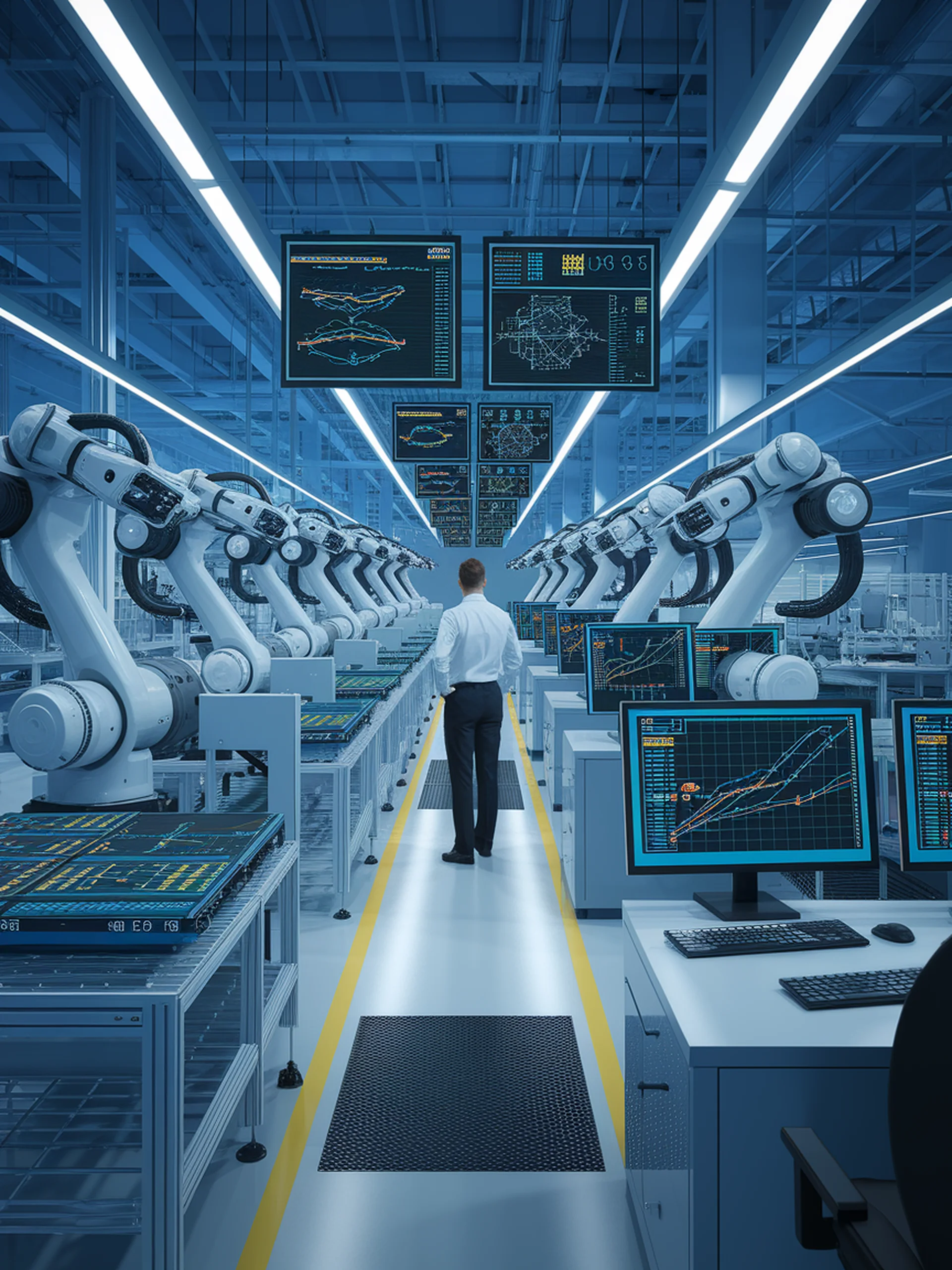
Self-Evaluation in Optimization
Applying LLM techniques to improve scheduling algorithms
This research introduces a novel self-evaluation framework for the Job-Shop Scheduling Problem, inspired by Large Language Model techniques to reduce error accumulation in combinatorial optimization.
- Addresses the critical challenge of error propagation in sequential decision-making processes
- Implements a neural network-based evaluation mechanism that assesses solution quality
- Demonstrates improved scheduling outcomes by identifying and correcting suboptimal decisions
- Provides a transferable approach that could benefit multiple industrial optimization scenarios
The framework represents a significant advancement for engineering applications in manufacturing, logistics, and resource planning, where even small improvements in scheduling efficiency can yield substantial operational benefits.