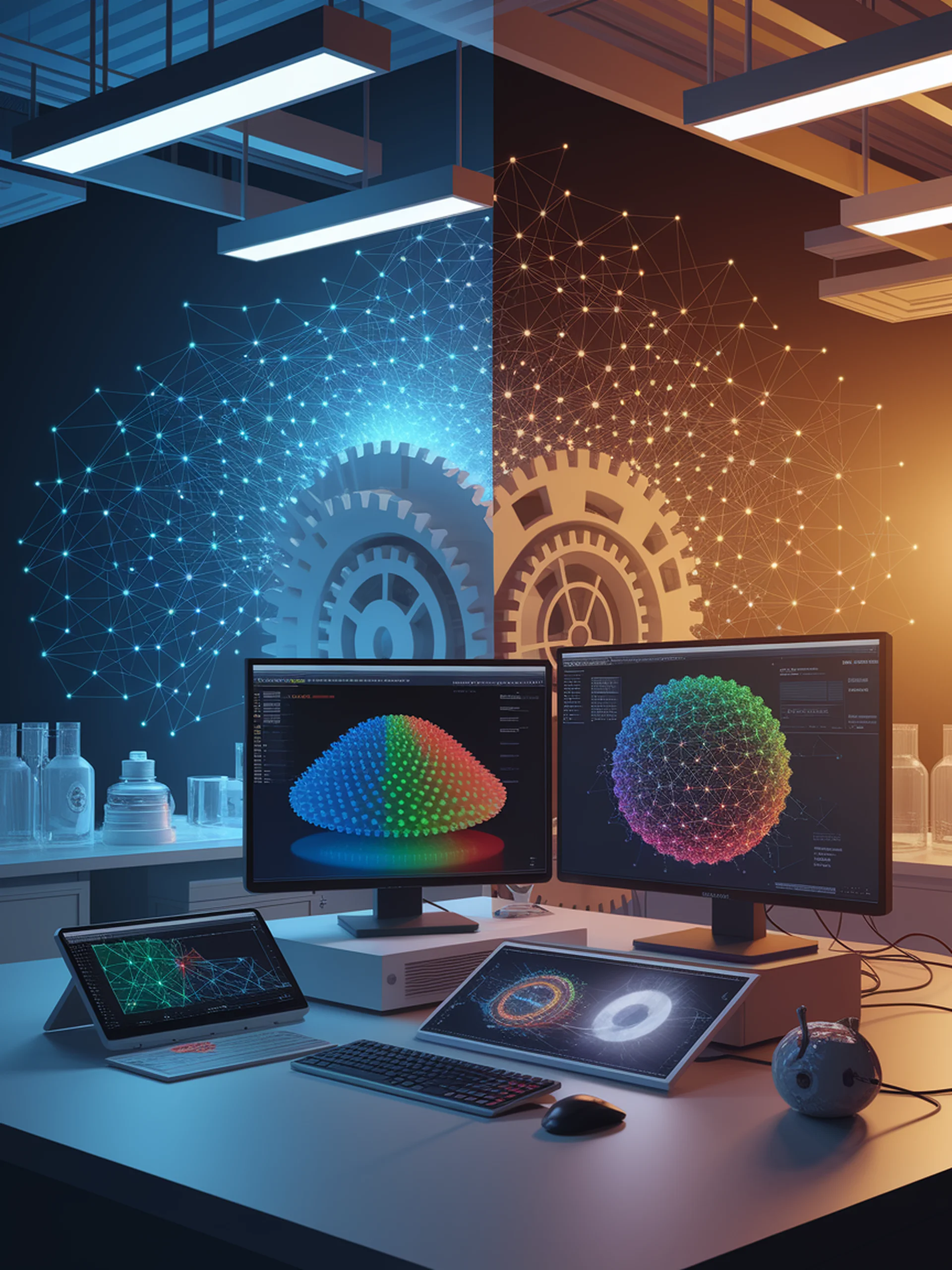
Benchmarking LLMs for Evolutionary Optimization
Using large language models to enhance multi-objective optimization algorithms
This research explores how large language models (LLMs) can be leveraged to design and benchmark evolutionary multi-objective optimization (EMO) algorithms, addressing the critical need for explicit target optimization problems.
- Emphasizes the importance of clearly defining target problems when using LLMs for algorithm design
- Identifies gaps in current LLM-based EMO algorithm development where optimization targets aren't explicitly specified
- Establishes a framework for evaluating the effectiveness of LLM-designed optimization algorithms
- Bridges AI language models with traditional engineering optimization techniques
For engineering applications, this work provides a structured approach to using AI-assisted algorithm design for solving complex multi-objective problems, potentially improving solutions for design optimization, resource allocation, and systems engineering challenges.