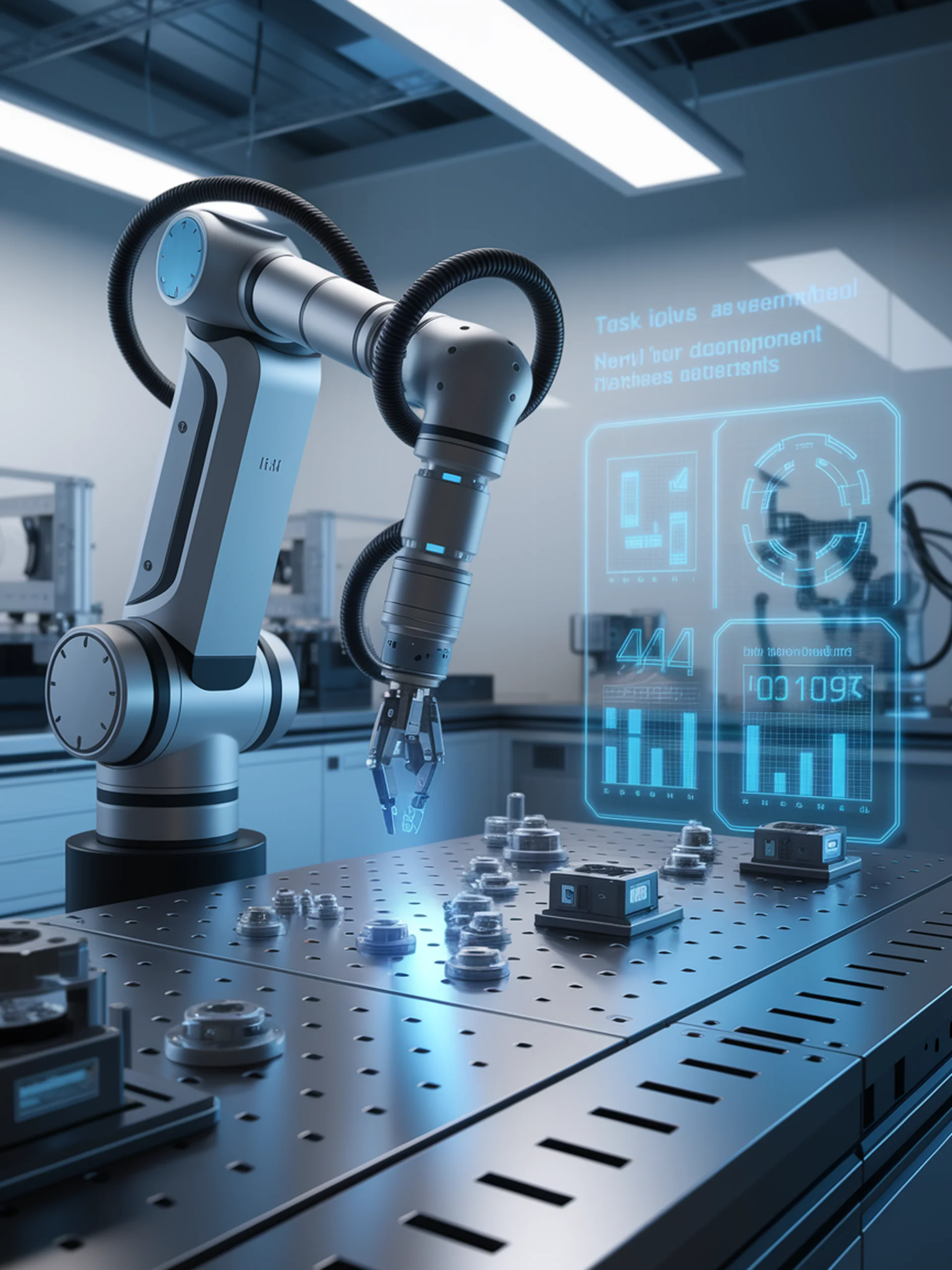
Smarter Robot Analysis using LLMs
Automating the evaluation of robotic tasks with language models
This research introduces an automated framework that uses large language models to decompose and evaluate robot trajectories into meaningful sub-tasks with natural language descriptions.
- Addresses the critical challenge of data scarcity in robot learning by enhancing task evaluation
- Leverages temporal and semantic metrics to analyze robot performance at a granular level
- Enables more effective post-hoc analysis of robotic task execution without requiring additional labeled data
- Creates a foundation for improved task planning systems through better understanding of robot behaviors
For engineering teams, this approach offers a scalable method to evaluate complex robotic operations without extensive human annotation, potentially accelerating development cycles and improving robot performance in manufacturing and automation contexts.