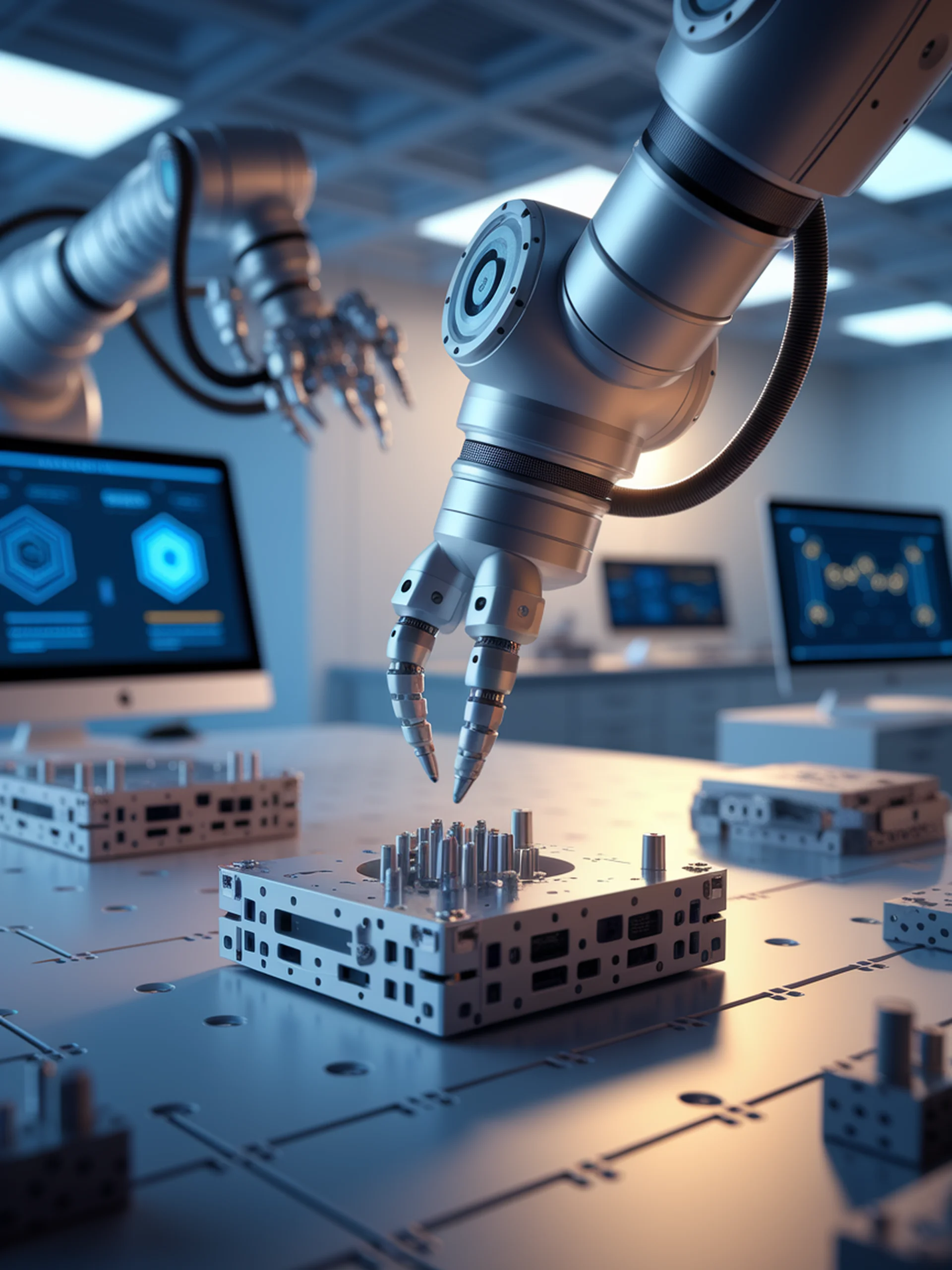
Multi-Modal Learning for Robotic Manipulation
Enhancing LLMs with vision and force feedback for precision tasks
LEMMo-Plan integrates vision, force feedback, and language models to enable robots to learn complex contact-rich manipulation tasks from demonstrations.
- Combines visual perception with force-torque feedback to capture subtle movements and contact interactions
- Implements a multi-modal encoder-decoder architecture that processes both visual and force data
- Achieves 85% success rate on contact-rich tasks compared to 35% for vision-only approaches
- Enables robots to handle tasks requiring precise force control like assembly and insertion
This breakthrough addresses critical limitations in factory automation by allowing robots to learn complex manual tasks that previously required human dexterity, potentially accelerating manufacturing automation for high-precision assembly tasks.