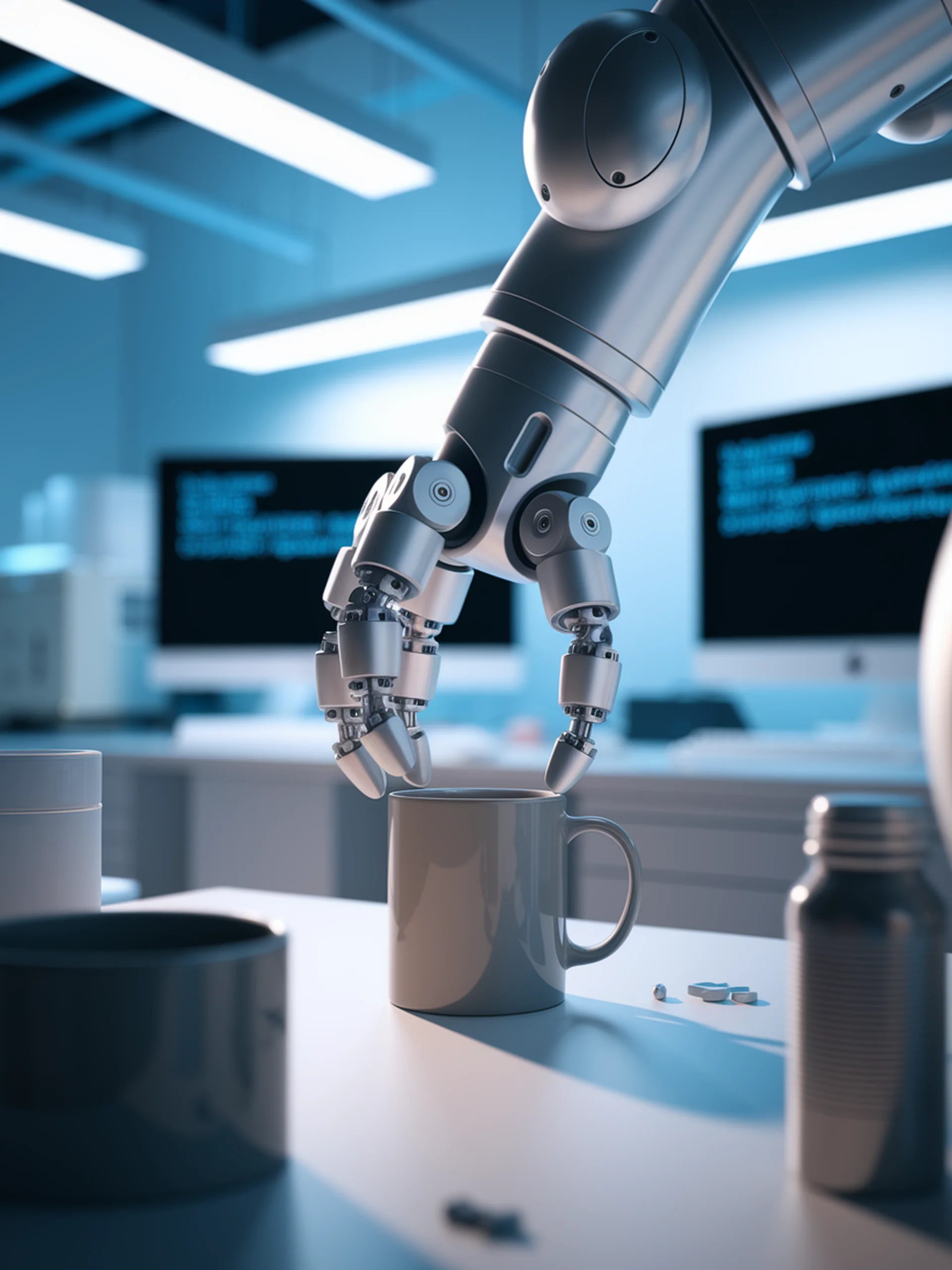
Efficient Language-Guided Robot Grasping
A Parameter-Efficient Framework for Robot Vision-Language Integration
This research introduces a lightweight, parameter-efficient framework for robots to understand natural language commands and grasp objects accurately without requiring massive computational resources.
- Overcomes limitations of resource-intensive Multimodal Large Language Models (MLLMs)
- Enables local deployment and customization through parameter-efficient tuning (PET)
- Integrates CLIP-based visual features with linguistic understanding
- Achieves comparable performance to larger models with significantly reduced computational demands
For engineering teams, this approach represents a practical path to implementing language-guided robotics in resource-constrained environments like factories and warehouses, making advanced robotic capabilities more accessible and deployable.
A Parameter-Efficient Tuning Framework for Language-guided Object Grounding and Robot Grasping