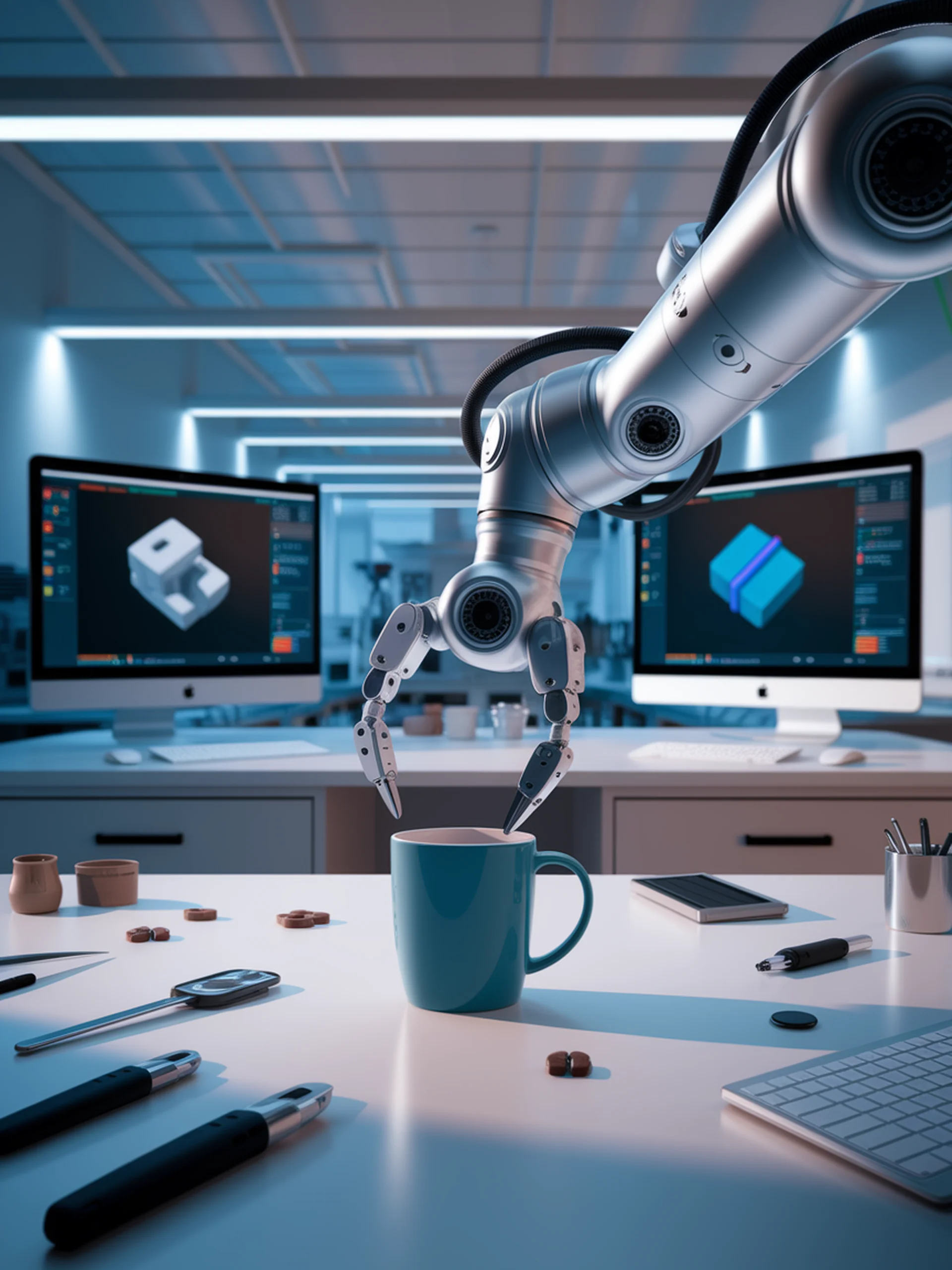
Smarter Robotic Grasping with AI
Combining LLMs and Quality Diversity for Zero-Shot Task-Aware Manipulation
This research introduces a novel framework that combines Large Language Models with Quality Diversity algorithms to enable robots to grasp objects intelligently based on intended tasks without prior training.
- Creates structured representations by segmenting objects into meaningful subparts with semantic labels
- Leverages LLMs to understand task requirements and select appropriate grasping strategies
- Demonstrates zero-shot performance for task-conditioned grasp synthesis
- Integrates semantic understanding with geometric reasoning for more effective robotic manipulation
This advancement has significant implications for engineering and factory automation by enabling more versatile and intuitive robotic systems that can understand the purpose of grasps in different contexts.
Task-Aware Robotic Grasping by evaluating Quality Diversity Solutions through Foundation Models