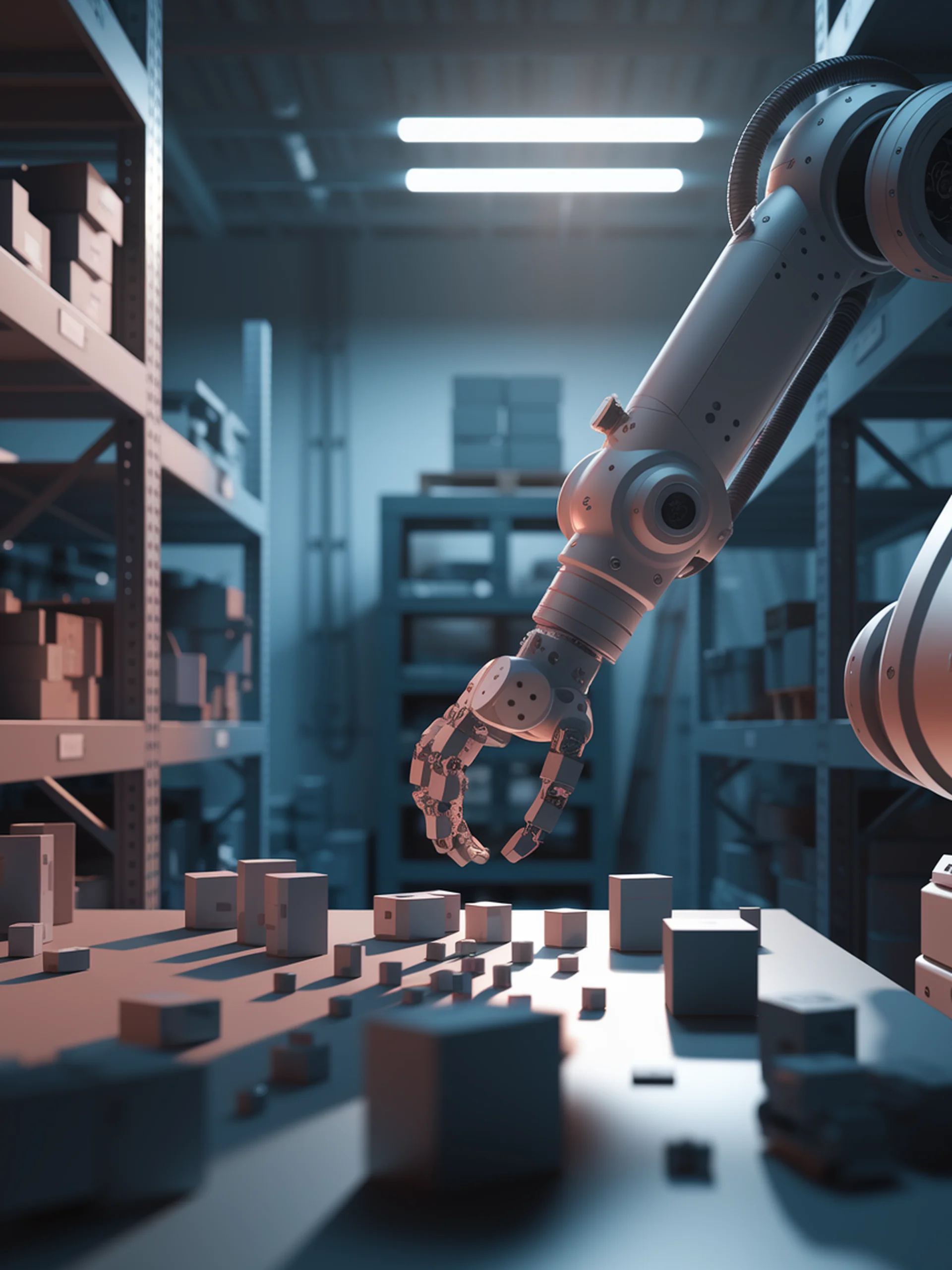
Smarter Robots for Cluttered Environments
Efficient language-guided pick and place using unconditioned action priors
This research introduces a novel approach for robots to perform language-guided pick and place tasks in cluttered environments by efficiently aligning unconditioned action priors with language commands.
- Combines vision foundation models with action priors to reduce training data requirements
- Implements an alignment module that connects language commands to appropriate robot actions
- Achieves state-of-the-art performance with 40% less training data than competing approaches
- Demonstrates practical robustness in real-world cluttered environments
This advancement matters for engineering by bridging the gap between high-level language commands and low-level robotic actions, making industrial automation more flexible and intuitive to program without extensive datasets.
Efficient Alignment of Unconditioned Action Prior for Language-conditioned Pick and Place in Clutter