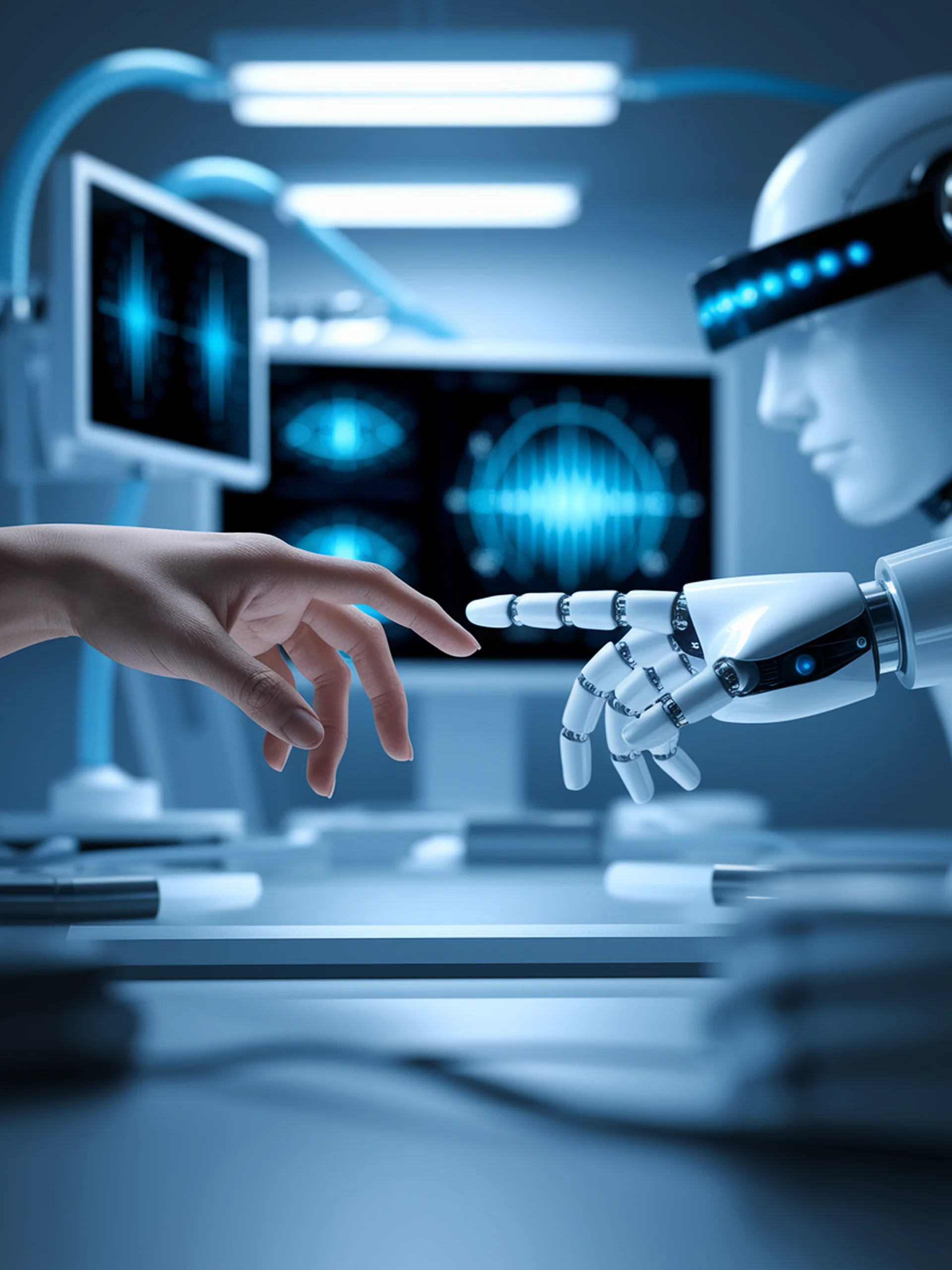
Making Robots Understand Human Intent
Integrating Gaze and Speech for Intuitive Human-Robot Interaction
This research presents SemanticScanpath, a novel approach that enables robots to understand human intent by combining speech and gaze data using Large Language Models (LLMs).
- Helps robots interpret ambiguous verbal commands by tracking where humans are looking
- Enhances human-robot communication by creating a multimodal understanding system
- Grounds conversations in the physical environment for more natural interaction
- Demonstrates how LLMs can process both verbal and non-verbal cues simultaneously
This engineering advancement significantly improves robotic assistants' ability to understand human intentions in real-world environments, making human-robot collaboration more intuitive and efficient for industrial, healthcare, and domestic applications.
SemanticScanpath: Combining Gaze and Speech for Situated Human-Robot Interaction Using LLMs